PhaDe: Practical Phantom Spoofing Attack Detection for Autonomous Vehicles
IEEE Transactions on Information Forensics and Security(2024)
摘要
Despite their prevalence and indispensability in the perception modules of autonomous vehicles, cameras have shown susceptibility to numerous attacks. Among them, the phantom spoofing attack is of significant concern. In such attacks, malefactors employ electronic display devices like projectors and display monitors to generate deceptive objects, thereby duping the object detectors in autonomous vehicles. However, existing detection methodologies are narrowly focused on a single device category, ignoring the multitude of devices that could be leveraged for attacks. Furthermore, the artificial modality-based solution presently in use lacks efficacious fusion mechanisms. In response to these limitations, we propose PhaDe, a practical deep learning-based system adept at detecting phantom spoofing attacks from a variety of and even unfamiliar attack devices. Our approach introduces two image processing techniques to construct artificial modalities and further advances a multi-head self-attention MSA-based fusion module for more versatile integration of disparate modalities. To boost the generalization capacity of our system against novel, unseen attacks, we incorporate two representation-level losses to align feature distributions from various domains. Evaluations conducted on our own dataset, encompassing fake objects from several device types, attest to the efficacy of our system. Our results indicate an accuracy of 98.80% on familiar domains and a detection success rate of 94.03% on unfamiliar domains. Additionally, PhaDe demonstrates a swift response time, fulfilling the practicality requisites.
更多查看译文
关键词
Vehicles security,phantom attack,detection system,deep learning,multi-modal fusion
AI 理解论文
溯源树
样例
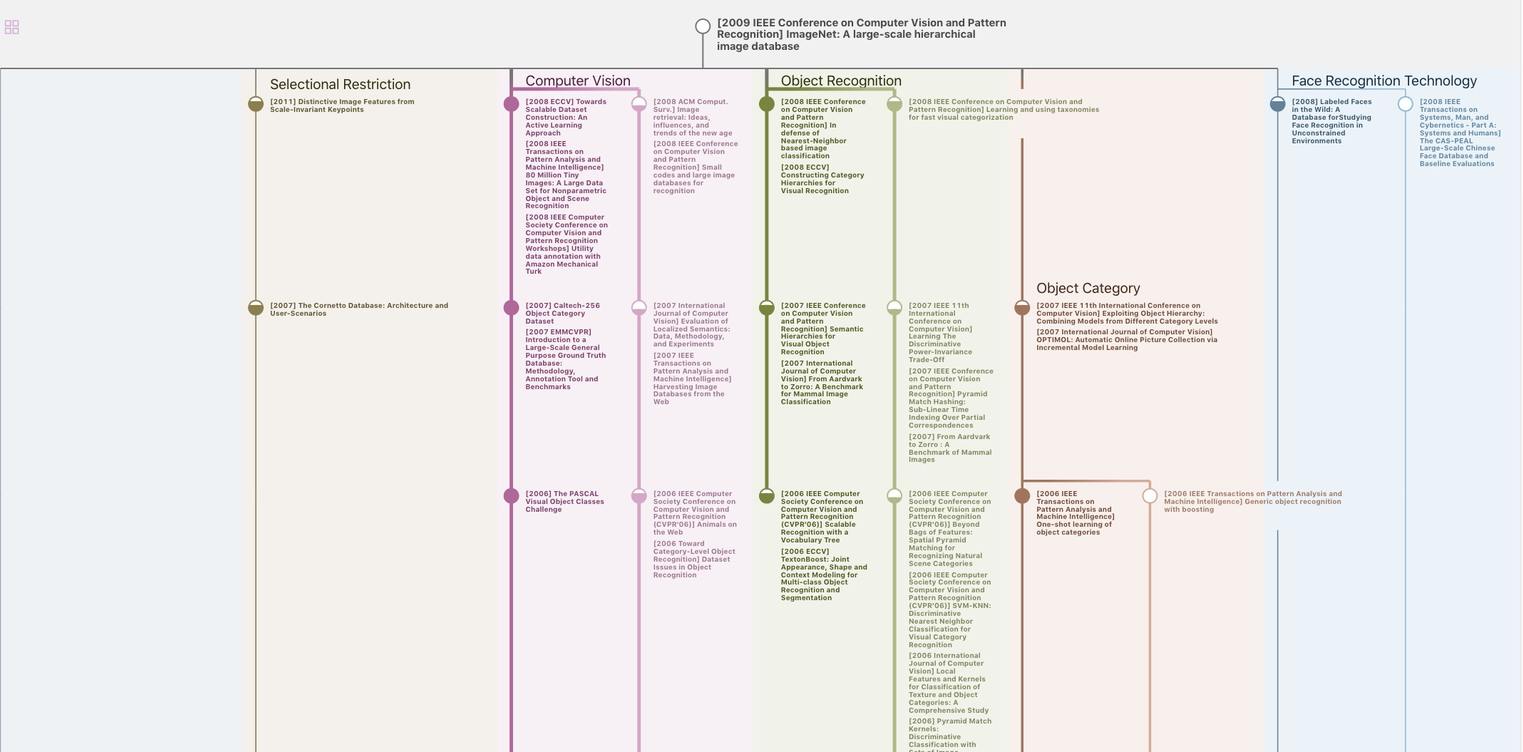
生成溯源树,研究论文发展脉络
Chat Paper
正在生成论文摘要