CLIP-BEVFormer: Enhancing Multi-View Image-Based BEV Detector with Ground Truth Flow
CVPR 2024(2024)
摘要
Autonomous driving stands as a pivotal domain in computer vision, shaping the
future of transportation. Within this paradigm, the backbone of the system
plays a crucial role in interpreting the complex environment. However, a
notable challenge has been the loss of clear supervision when it comes to
Bird's Eye View elements. To address this limitation, we introduce
CLIP-BEVFormer, a novel approach that leverages the power of contrastive
learning techniques to enhance the multi-view image-derived BEV backbones with
ground truth information flow. We conduct extensive experiments on the
challenging nuScenes dataset and showcase significant and consistent
improvements over the SOTA. Specifically, CLIP-BEVFormer achieves an impressive
8.5% and 9.2% enhancement in terms of NDS and mAP, respectively, over the
previous best BEV model on the 3D object detection task.
更多查看译文
AI 理解论文
溯源树
样例
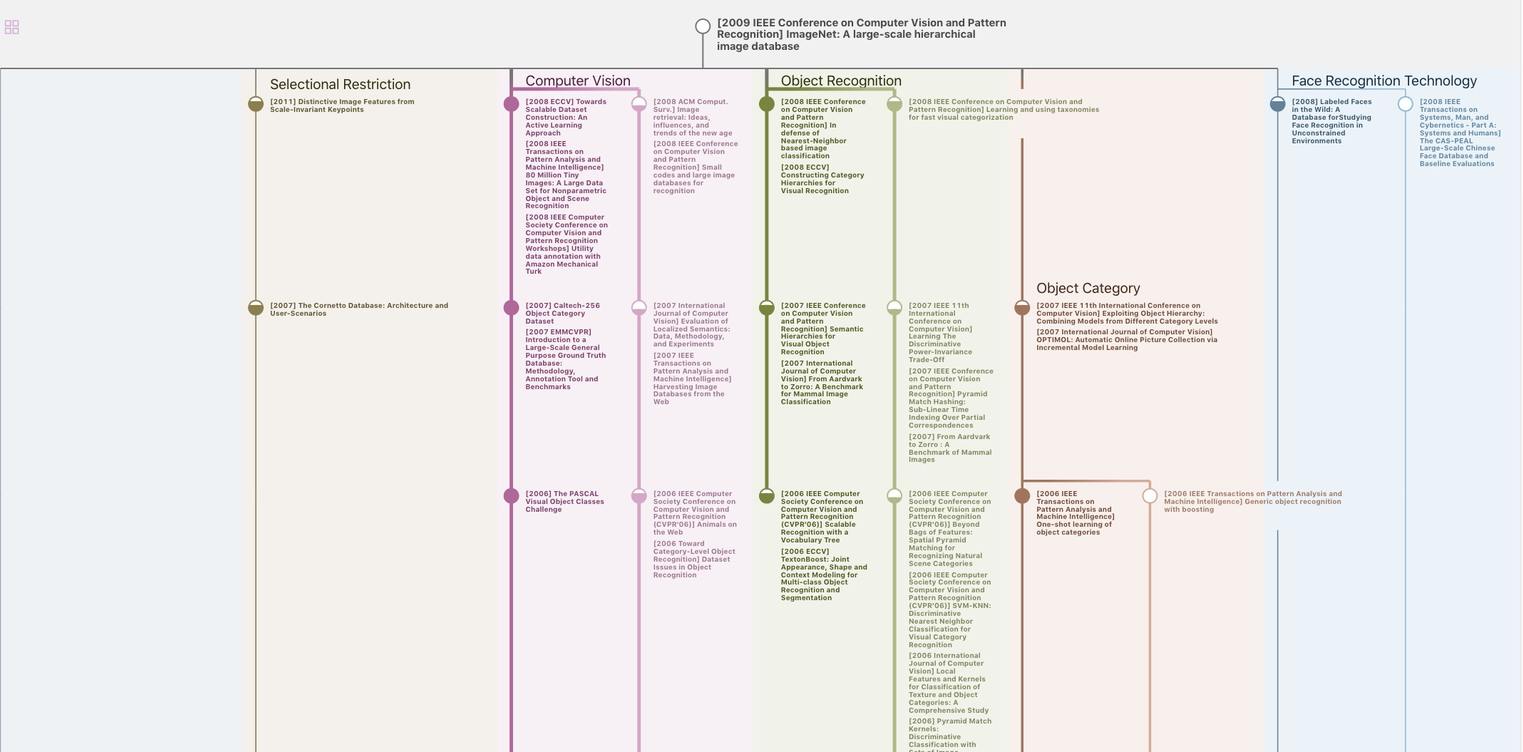
生成溯源树,研究论文发展脉络
Chat Paper
正在生成论文摘要