TEA+ : A Novel Temporal Graph Random Walk Engine With Hybrid Storage Architecture
ACM Transactions on Architecture and Code Optimization(2024)
摘要
Many real-world networks are characterized by being temporal and dynamic, wherein the temporal information signifies the changes in connections, such as the addition or removal of links between nodes. Employing random walks on these temporal networks is a crucial technique for understanding the structural evolution of such graphs over time. However, existing state-of-the-art sampling methods are designed for traditional static graphs, and as such, they struggle to efficiently handle the dynamic aspects of temporal networks. This deficiency can be attributed to several challenges, including increased sampling complexity, extensive index space, limited programmability, and a lack of scalability. In this paper, we introduce TEA+ , a robust, fast, and scalable engine for conducting random walks on temporal graphs. Central to TEA+ is an innovative hybrid sampling method that amalgamates two Monte Carlo sampling techniques. This fusion significantly diminishes space complexity while maintaining a fast sampling speed. Additionally, TEA+ integrates a range of optimizations that significantly enhance sampling efficiency. This is further supported by an effective graph updating strategy, skilled in managing dynamic graph modifications and adeptly handling the insertion and deletion of both edges and vertices. For ease of implementation, we propose a temporal-centric programming model, designed to simplify the development of various random walk algorithms on temporal graphs. To ensure optimal performance across storage constraints, TEA+ features a degree-aware hybrid storage architecture, capable of adeptly scaling in different memory environments. Experimental results showcase the prowess of TEA+ , as it attains up to three orders of magnitude speedups compared to current random walk engines on extensive temporal graphs.
更多查看译文
AI 理解论文
溯源树
样例
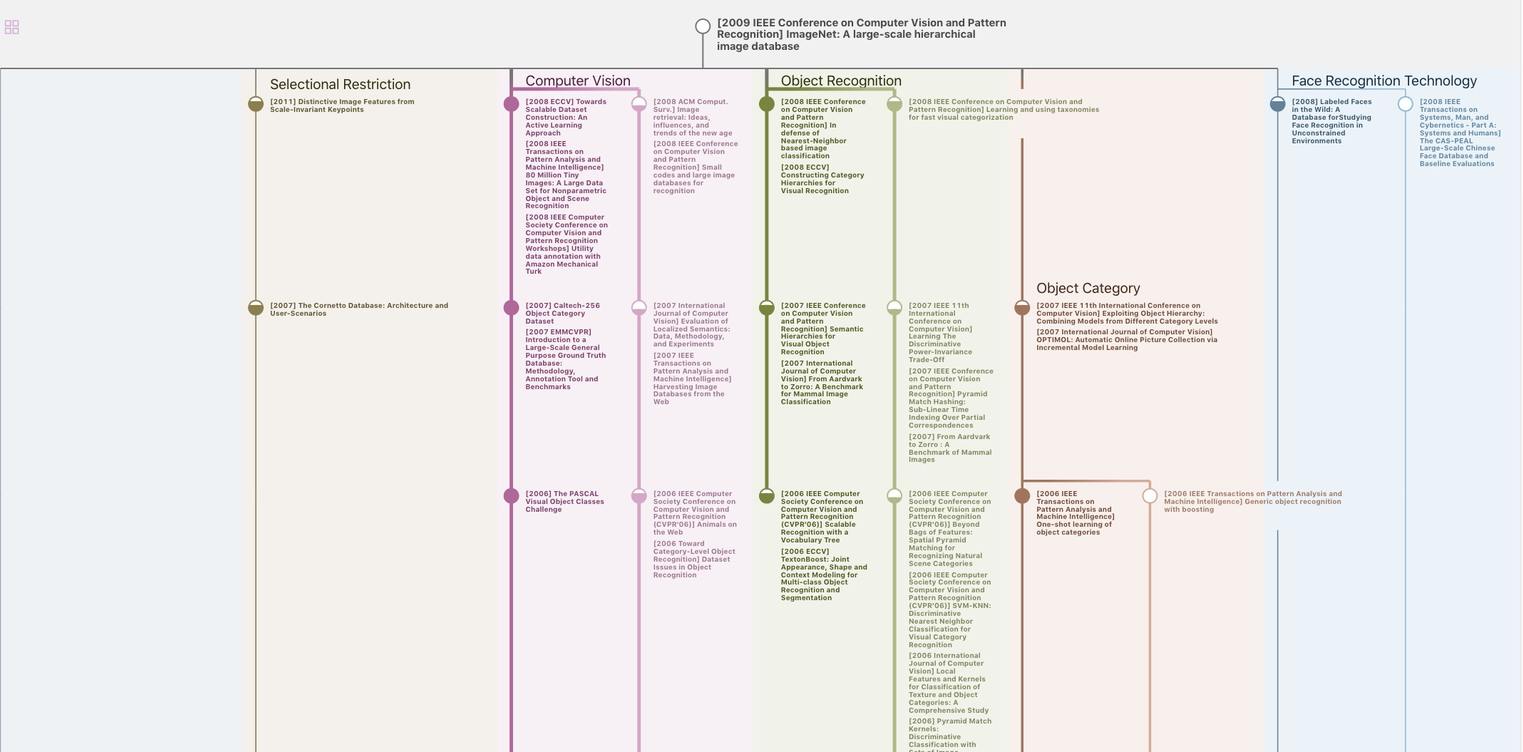
生成溯源树,研究论文发展脉络
Chat Paper
正在生成论文摘要