Hybrid Improved Concave Matching Algorithm and ResNet Image Recognition Model
IEEE ACCESS(2024)
摘要
With the increase of organic matter content in global water bodies, timely and accurate identification of algae objects in water bodies is of great significance for rapid treatment of water eutrophication. To improve the performance of seaweed microscopic image recognition model, an improved concave matching algorithm was designed and applied to the segmentation of original microscopic images. In addition, an IResNet algorithm with self-adjusting pooling layer is designed. In this study, an improved concave matching algorithm and an improved ResNet algorithm were used to construct a microscopic algae image recognition model. The average Accuracy and Precision of the proposed algorithm in the data set are 88.6% and 90.3%, which is significantly higher than all tr4aditional and mainstream image segmentation algorithms. Among the five modified ResNet algorithms with self-adjusting pooling layers designed in this study, the fifth modified scheme has the best performance on the training set. The model's recognition accuracy and accuracy corresponding to the improved ResNet algorithm on the whole test set are 84.1% and 82.8%, respectively. The experimental data on the real data set show that the algae microscopic image recognition model has certain practical potential.
更多查看译文
关键词
Concave programming,Neural networks,Microscopy,Algae,Water resources,Water monitoring,Pollution measurement,Organic materials,Image recognition,Seaweed,Object recognition,Data models,Water pollution,Eutrophication,Concave point matching algorithm,neural network,ResNet,algae microscopic image
AI 理解论文
溯源树
样例
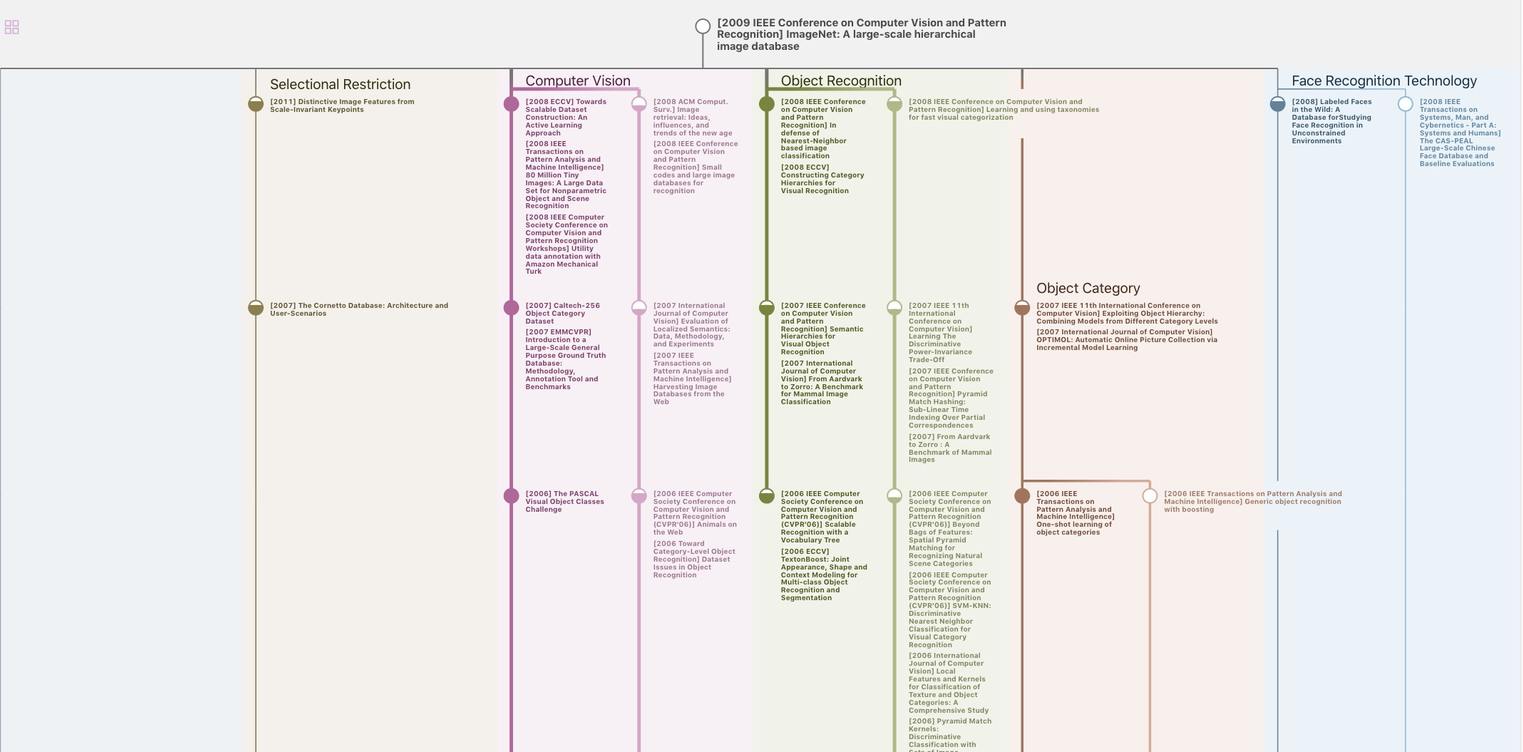
生成溯源树,研究论文发展脉络
Chat Paper
正在生成论文摘要