A Probabilistic Method for Integrating Physics-Based and Data-Driven Storm Outage Prediction Models for Power Systems
ASCE-ASME JOURNAL OF RISK AND UNCERTAINTY IN ENGINEERING SYSTEMS PART A-CIVIL ENGINEERING(2024)
摘要
To predict power outages for upcoming storm events and assess the resilience of power systems, accurate models of power outage probabilities are necessary. Although physics-based fragility models have been developed to forecast structural failure probabilities under strong winds, such methods may capture limited failure modes and could struggle to predict more-random outages that are not directly related to strong winds. Data-driven machine learning methods have emerged for power outage predictions considering multiple failure modes, although they may struggle to predict cases with limited data, such as extreme events. To integrate the two different approaches, a novel model is proposed to probabilistically combine physics-based and machine-learning predictions. Fragility analyses of various transmission structure types and tree species subject to wind loads were conducted for the prediction of wind-induced structural damages. An extreme gradient boosting (XGboost) machine learning model trained on infrastructure, weather, topographic, and vegetation characteristics is used for the prediction of nonstructural outage probabilities. The framework is demonstrated through a case study of the overhead transmission system in the New England region of the US over 44 storm events from 2015 to 2020. The results show an improved agreement between predicted and observed outages, as well as correlations between predicted probability and observed outage rate, highlighting the model's effectiveness for estimations of storm impacts and grid vulnerability.
更多查看译文
关键词
Transmission power outage prediction,Fragility curve,Machine learning,Outage probability,Failure modes,Tree impact
AI 理解论文
溯源树
样例
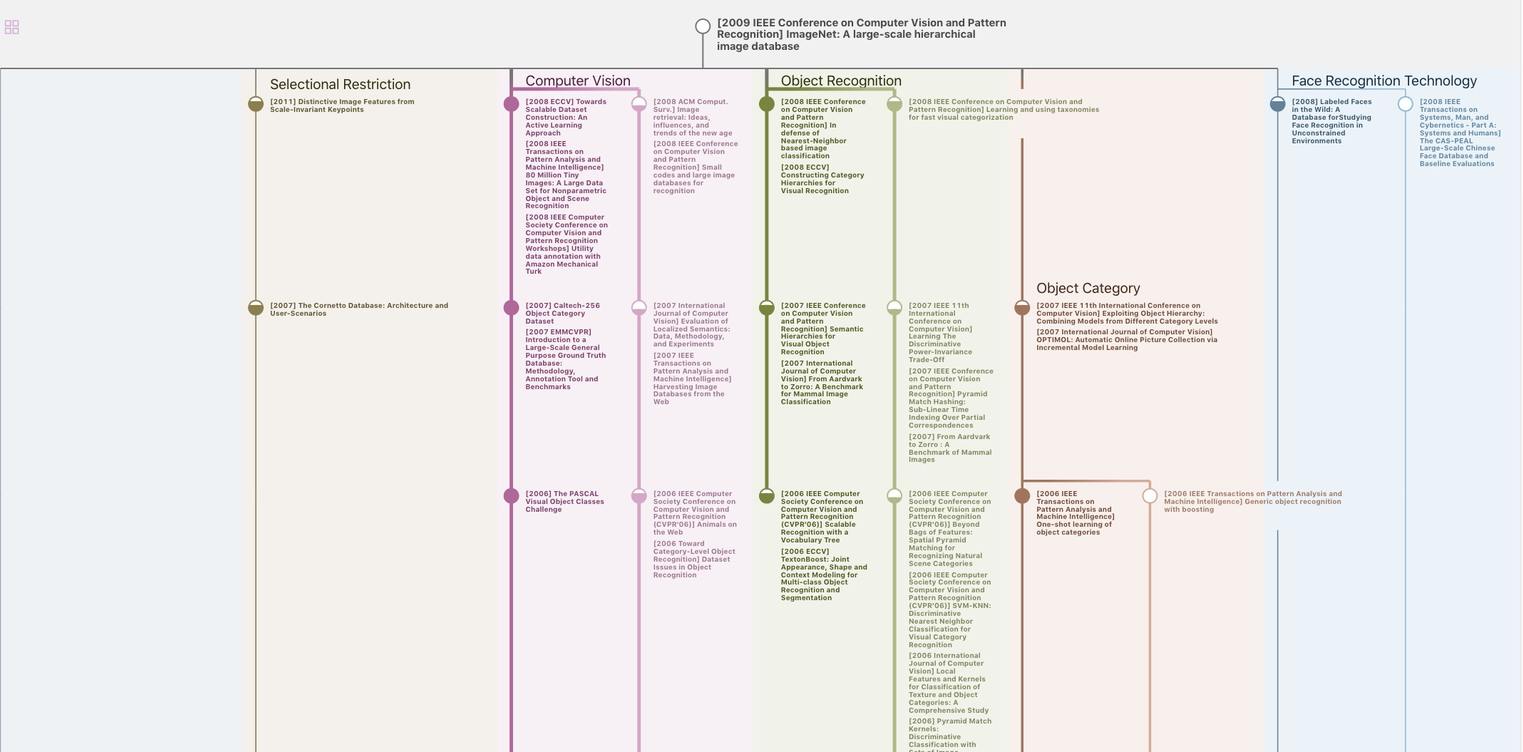
生成溯源树,研究论文发展脉络
Chat Paper
正在生成论文摘要