Structure Sensitivity of Metal Catalysts Revealed by Interpretable Machine Learning and First-Principles Calculations.
Journal of the American Chemical Society(2024)
摘要
The nature of the active sites and their structure sensitivity are the keys to rational design of efficient catalysts but have been debated for almost one century in heterogeneous catalysis. Though the Brønsted-Evans-Polanyi (BEP) relationship along with linear scaling relation has long been used to study the reactivity, explicit geometry, and composition properties are absent in this relationship, a fact that prevents its exploration in structure sensitivity of supported catalysts. In this work, based on interpretable multitask symbolic regression and a comprehensive first-principles data set, we discovered a structure descriptor, the topological under-coordinated number mediated by number of valence electrons and the lattice constant, to successfully address the structure sensitivity of metal catalysts. The database used for training, testing, and transferability investigation includes bond-breaking barriers of 20 distinct chemical bonds over 10 transition metals, two metal crystallographic phases, and 17 different facets. The resulting 2D descriptor composing the structure term and the reaction energy term shows great accuracy to predict the reaction barriers and generalizability over the data set with diverse chemical bonds in symmetry, bond order, and steric hindrance. The theory is physical and concise, providing a constructive strategy not only to understand the structure sensitivity but also to decipher the entangled geometric and electronic effects of metal catalysts. The insights revealed are valuable for the rational design of the site-specific metal catalysts.
更多查看译文
AI 理解论文
溯源树
样例
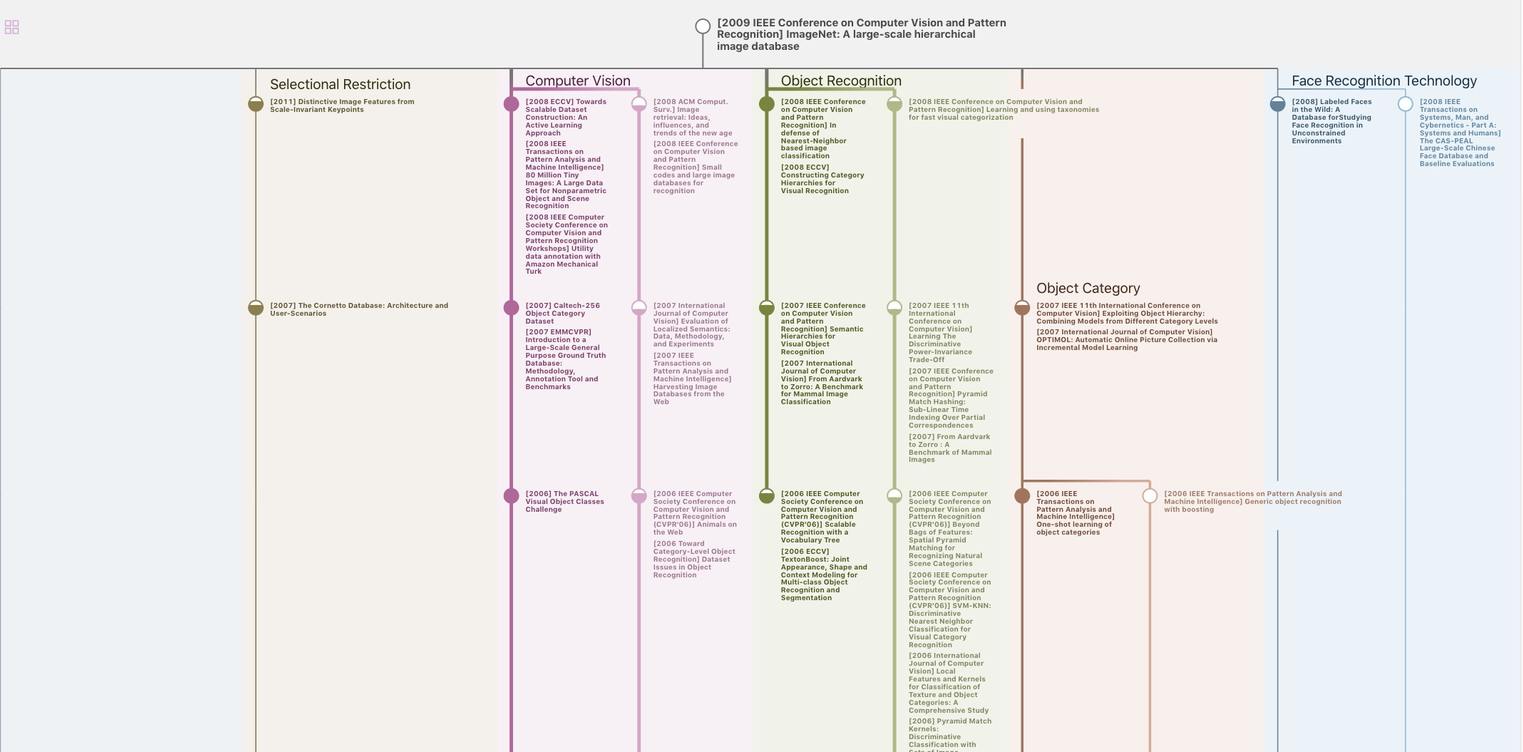
生成溯源树,研究论文发展脉络
Chat Paper
正在生成论文摘要