Anycost Network Quantization for Image Super-Resolution.
IEEE transactions on image processing : a publication of the IEEE Signal Processing Society(2024)
摘要
In this paper, we propose an anycost network quantization method for efficient image super-resolution with variable resource budgets. Conventional quantization approaches acquire discrete network parameters for deployment with fixed complexity constraints, while image super-resolution networks are usually applied on mobile devices with frequently modified resource budgets due to the change of battery levels or computing chips. Hence, exhaustively optimizing quantized networks with each complexity constraint results in unacceptable training costs. On the contrary, we construct a hyper-network whose parameters can efficiently adapt to different resource budgets with negligible finetuning cost, so that the image super-resolution networks can be feasibly deployed in diversified devices with variable resource budgets. Specifically, we dynamically search the optimal bitwidth for each patch in convolution according to feature maps and complexity constraints, which aims to achieve the best efficiency-accuracy trade-off in image super-resolution given the resource budget. To acquire the hyper-network that can be efficiently adapted to different bitwidth settings, we actively sample the patch-wise bitwidth during training and adaptively ensemble gradients from hyper-network in different precision for faster convergence and higher generalization ability. Compared with existing quantization methods, experimental results demonstrate that our method significantly reduces the cost of adapting models in new resource budgets with comparable efficiency-accuracy trade-offs.
更多查看译文
AI 理解论文
溯源树
样例
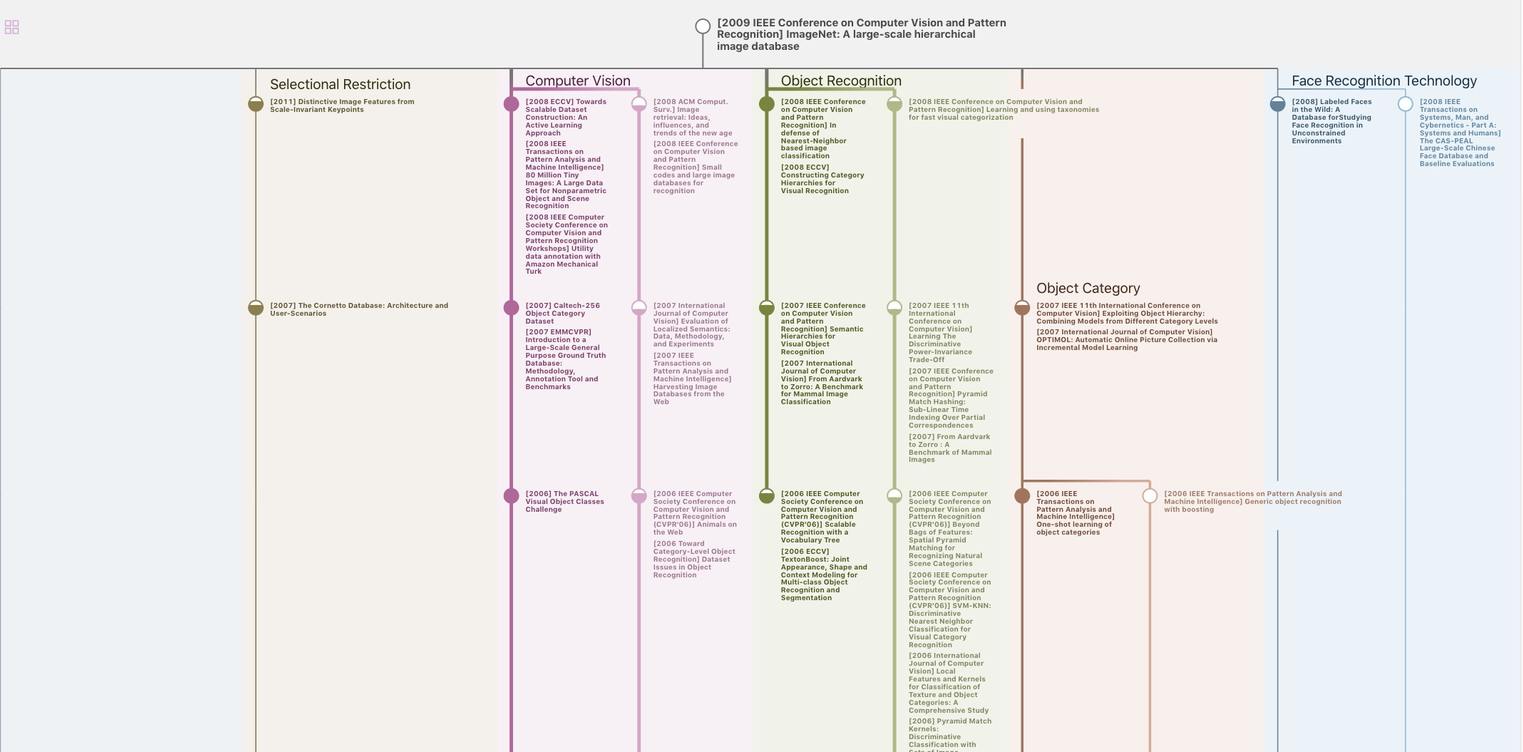
生成溯源树,研究论文发展脉络
Chat Paper
正在生成论文摘要