Upgrading the Density Functional Theory with Machine Learning for the Fast Prediction of Polyaromatic Reactivity at Bimetallic Catalysts
JOURNAL OF PHYSICAL CHEMISTRY C(2024)
摘要
Polyaromatic molecules are compounds of major importance in chemistry. However, simulating their reactivity at the solid catalyst surface with density functional theory (DFT) is very challenging. Indeed, such species require large slab models for their adsorption, hence resulting in a considerable number of atoms and thus significant computational time. In the recent context of increasing use in machine learning (ML), it is clear that such tools are of first interest to speed-up DFT calculations. Considering anthraquinone (AQ) hydrogenation on the surface of metal-doped Pd-based supported catalysts as a model reaction and focusing on the main reaction products, we propose here a method aiming at predicting the energy of the determining states from several descriptors related to a small molecular fragment, benzoquinone (BZQ) adsorbed at different surfaces. We were able to identify two distinct models, both performing with a high efficiency and based on different kinds of descriptors. While the first one involving a single thermodynamic descriptor is more accurate, the second one including a combination of electronic and geometric parameters is still relevant to predict reliable qualitative trends. Interestingly, we showed that simple linear regression tools can compete with other complex ML techniques, providing very accurate models with remarkable stability. Such an approach can be applied to easily assess the effective barriers of formation of several species on catalysts presenting different bimetallic compositions, hence enabling the screening of the catalytic activity and selectivity of various surfaces in a record time. While heavy DFT computations are generally required to optimize each intermediate and transition state, our strategy relies on a single adsorbate relaxation, hence, resulting in a tremendous gain of time. Therefore, our method is crucial for the accelerated computational design of solid catalysts and may have applications in various fields of the chemical industry.
更多查看译文
AI 理解论文
溯源树
样例
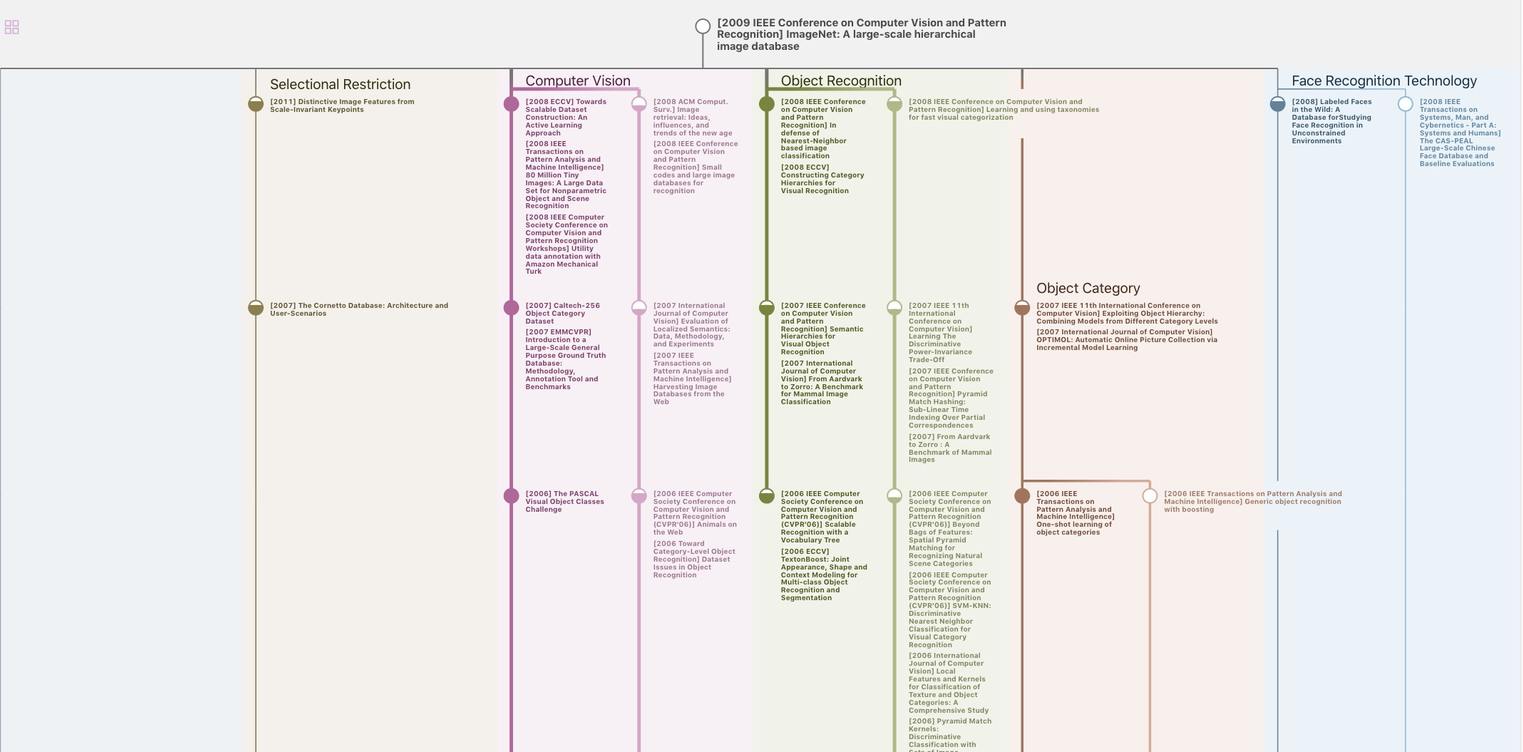
生成溯源树,研究论文发展脉络
Chat Paper
正在生成论文摘要