WT-FCTGN: A Wavelet-Enhanced Fully Connected Time-Gated Neural Network for Complex Noisy Traffic Flow Modeling
Chinese Physics B(2024)
摘要
Abstract Accurate forecasting of traffic flow is able to provide powerful traffic decision-making basis for intelligent transportation system, but the traffic data's complexity and fluctuation, as well as and the noise produced during collecting information and summarizing original data of traffic flow make the traffic flow forecasting result to have large error. This essay suggests a solution to the aforementioned issues and proposes a fully connected time-gated neural network based on wavelet reconstruction (WT-FCTGN). In order to eliminate the potential noise and strengthen the potential traffic trend in the data, we adopt the methods of wavelet reconstruction and periodic data introduction to preprocess the data. The model introduces fully connected time-series blocks to model the whole information including time sequence information and fluctuation information in the flow of traffic, and establishes the time gate block to comprehend the periodic characteristics of the flow of traffic and predict the flow of traffic. The performance of WT-FCTGN model is validated on the public PeMS data set. The experimental results show that WT-FCTGN model has higher accuracy, and its mean absolute error (MAE), mean absolute percentage error (MAPE) and root mean squared error(RMSE) are obviously lower than that of the other algorithms. The robust experimental results prove that WT-FCTGN model has good anti-noise ability.
更多查看译文
AI 理解论文
溯源树
样例
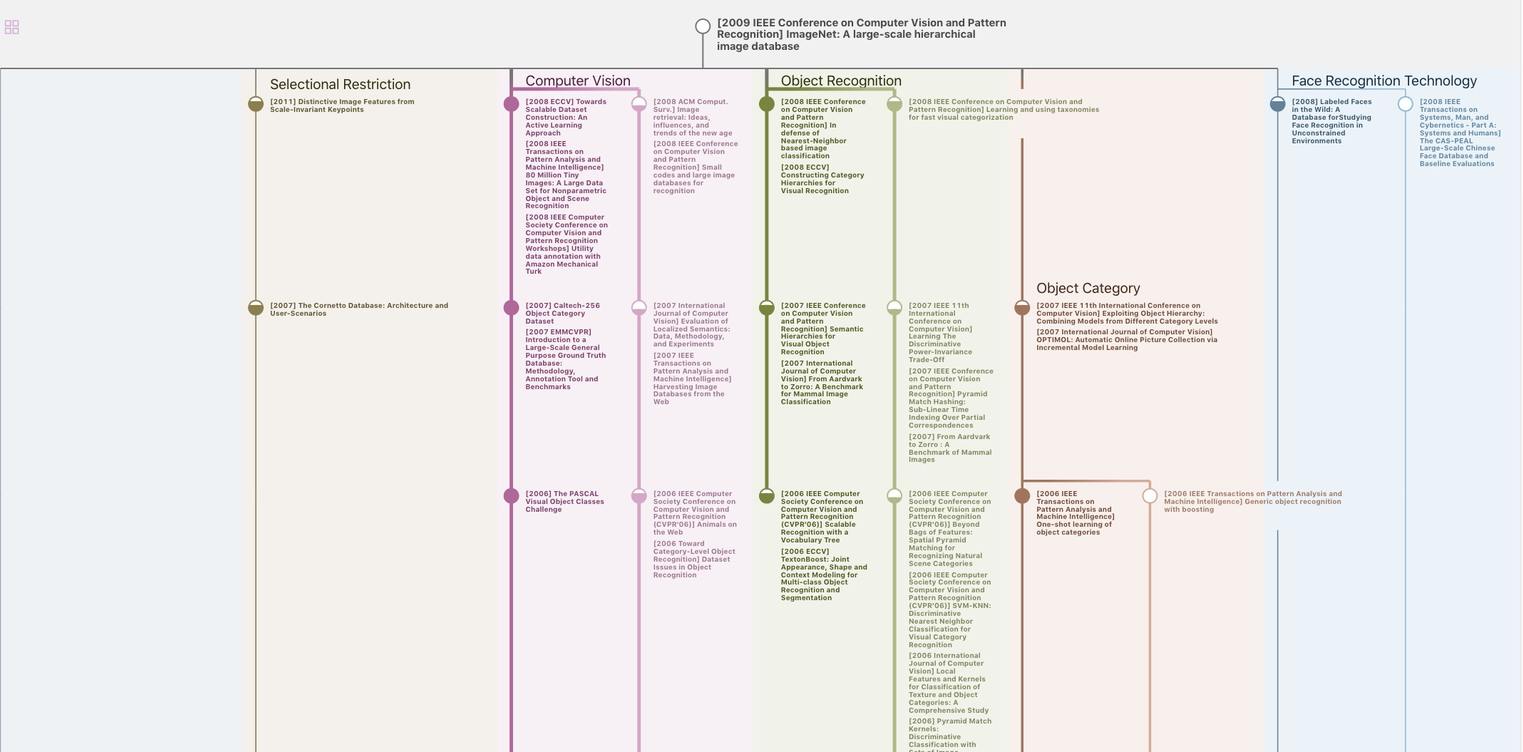
生成溯源树,研究论文发展脉络
Chat Paper
正在生成论文摘要