Temporal and spatial satellite data augmentation for deep learning-based rainfall nowcasting
JOURNAL OF HYDROINFORMATICS(2024)
摘要
The significance of improving rainfall prediction methods has escalated due to climate change-induced flash floods and severe flooding. In this study, rainfall nowcasting has been studied utilizing NASA Giovanni (Goddard Interactive Online Visualization and Analysis Infrastructure) satellite-derived precipitation products and the convolutional long short-term memory (ConvLSTM) approach. The goal of the study is to assess the impact of data augmentation on flood nowcasting. Due to data requirements of deep learning-based prediction methods, data augmentation is performed using eight different interpolation techniques. Spatial, temporal, and spatio-temporal interpolated rainfall data are used to conduct a comparative analysis of the results obtained through nowcasting rainfall. This research examines two catastrophic floods that transpired in the Turkiye Marmara Region in 2009 and the Central Black Sea Region in 2021, which are selected as the focal case studies. The Marmara and Black Sea regions are prone to frequent flooding, which, due to the dense population, has devastating consequences. Furthermore, these regions exhibit distinct topographical characteristics and precipitation patterns, and the frontal systems that impact them are also dissimilar. The nowcast results for the two regions exhibit a significant difference. Although data augmentation significantly reduced the error values by 59% for one region, it did not yield the same effectiveness for the other region. HIGHLIGHTS center dot A ConvLSTM model was created for rainfall nowcasting. center dot Satellite data augmentation was conducted by employing eight distinct interpolation approaches. center dot The investigation examined two flood case studies and their nowcast outcomes using original and upgraded data. center dot Although the use of augmented data reduced forecast error by 50% in one flood event, its impact on the nowcast result in the other event was found to be negligible.
更多查看译文
关键词
data augmentation,deep learning,flood,interpolation,nowcasting,rainfall
AI 理解论文
溯源树
样例
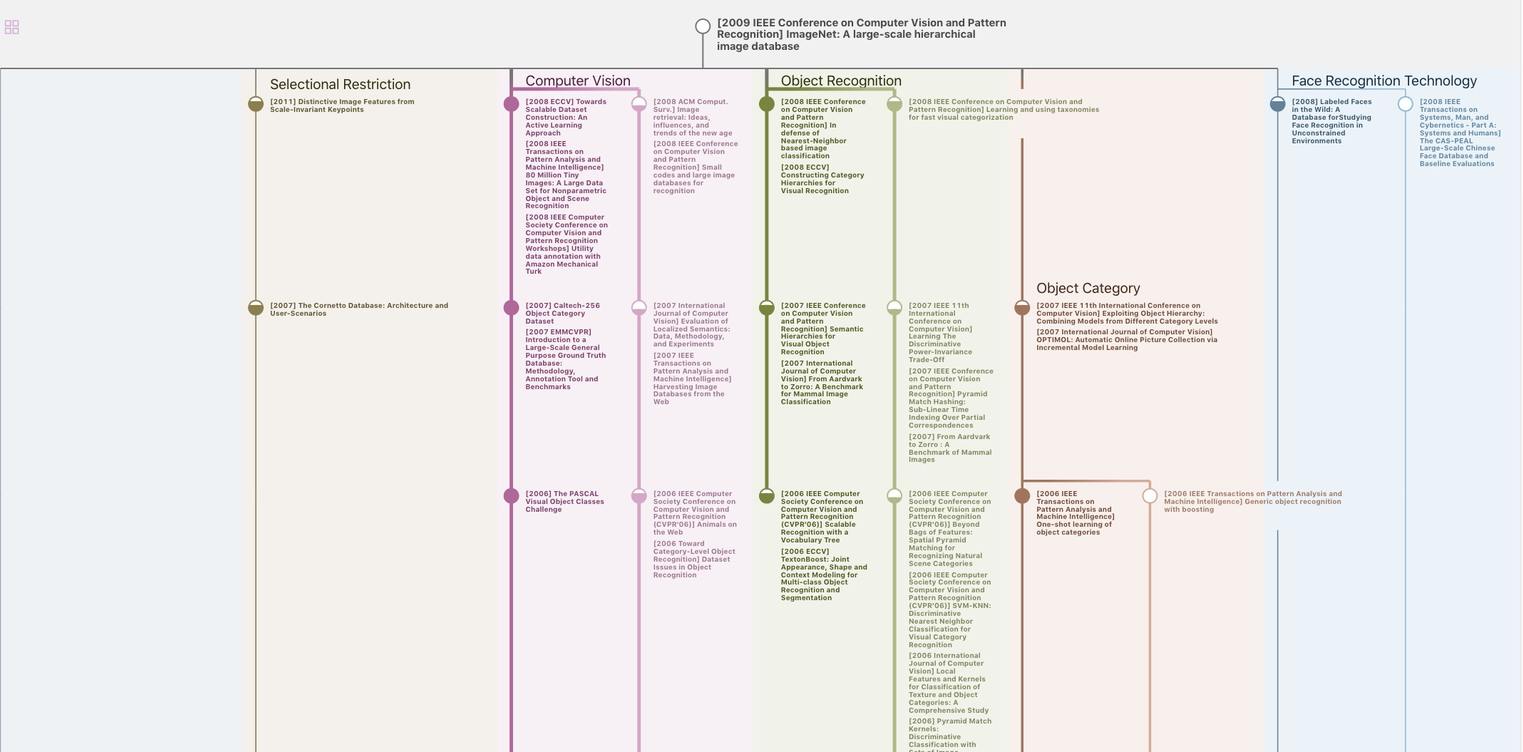
生成溯源树,研究论文发展脉络
Chat Paper
正在生成论文摘要