11.4 IBM NorthPole: An Architecture for Neural Network Inference with a 12nm Chip
2024 IEEE International Solid-State Circuits Conference (ISSCC)(2024)
摘要
The Deep Neural Network (DNN) era was ushered in by the triad of algorithms, big data, and more powerful hardware processors for training large-scale neural networks. Now, the ubiquitous deployment of DNNs for neural inference in edge, embedded, and data center applications demands more power-efficient hardware processors, while attaining increasingly higher computational performance. To address this Inference Challenge, we developed the NorthPole Architecture and implemented a NorthPole Chip instantiation [1, 2].
更多查看译文
关键词
Neural Network,North Pole,Deep Neural Network,Big Data,Weight Matrix,Functional Unit,Heat Sink,Computing Units,Cardinal Directions,Partial Sums,Precise Selection,Input Tensor,ResNet Model,Regeneration Buffer,Software Development Kit,Output Tensor,Voltage Scaling,Off-chip Memory
AI 理解论文
溯源树
样例
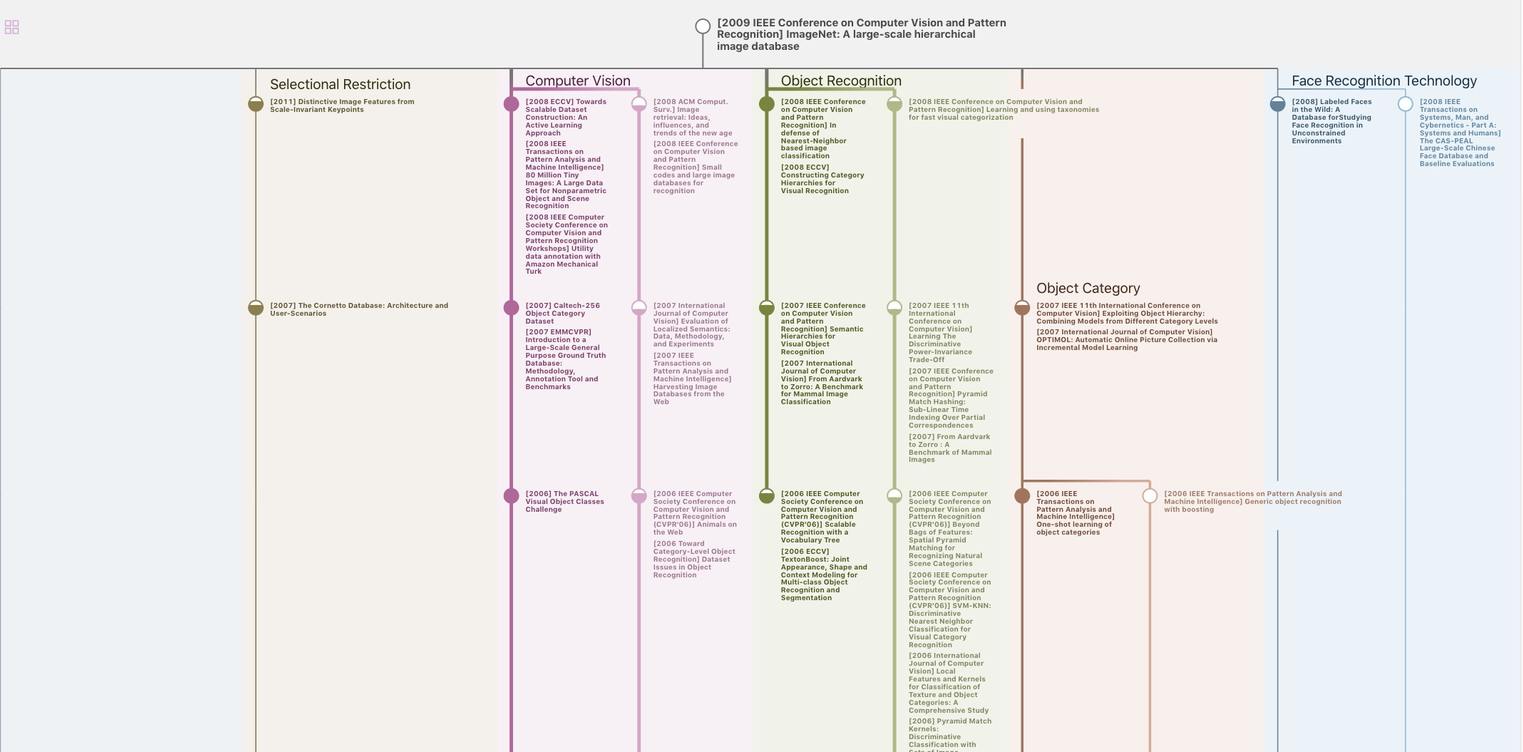
生成溯源树,研究论文发展脉络
Chat Paper
正在生成论文摘要