An improved federated deep learning for plant leaf disease detection
Multimedia Tools and Applications(2024)
摘要
Leaf diseases are hazardous to the yield and quality of food crops. There are many deep learning algorithms developed for their detection that may require large computational resources to train a single model with voluminous amounts of data. In the agriculture domain, various plant diseases are found across the country. Accumulating such a huge dataset from various regions is a tedious task, and training them with a single model can also be challenging. This paper proposes Federated Deep Learning (FDL) for Plant Leaf Disease Detection. This concept allows multiple local models to get trained with their region-based datasets and share their knowledge with siblings through the parent, instead of sharing complete datasets. Knowledge transfer significantly reduces the computational costs. This paper formulates the federated dataset using PlantVillage, to simulate the configuration of the FDL. A lightweight and efficient Hierarchical Convolutional Neural Network (H-CNN) is proposed for parent model and child models with 0.09 million parameters and 0.35MB model size. The simulation results show that the proposed FDL attains 93
更多查看译文
关键词
Federated deep learning,Knowledge transfer,Centralized learning,Plant leaf disease detection
AI 理解论文
溯源树
样例
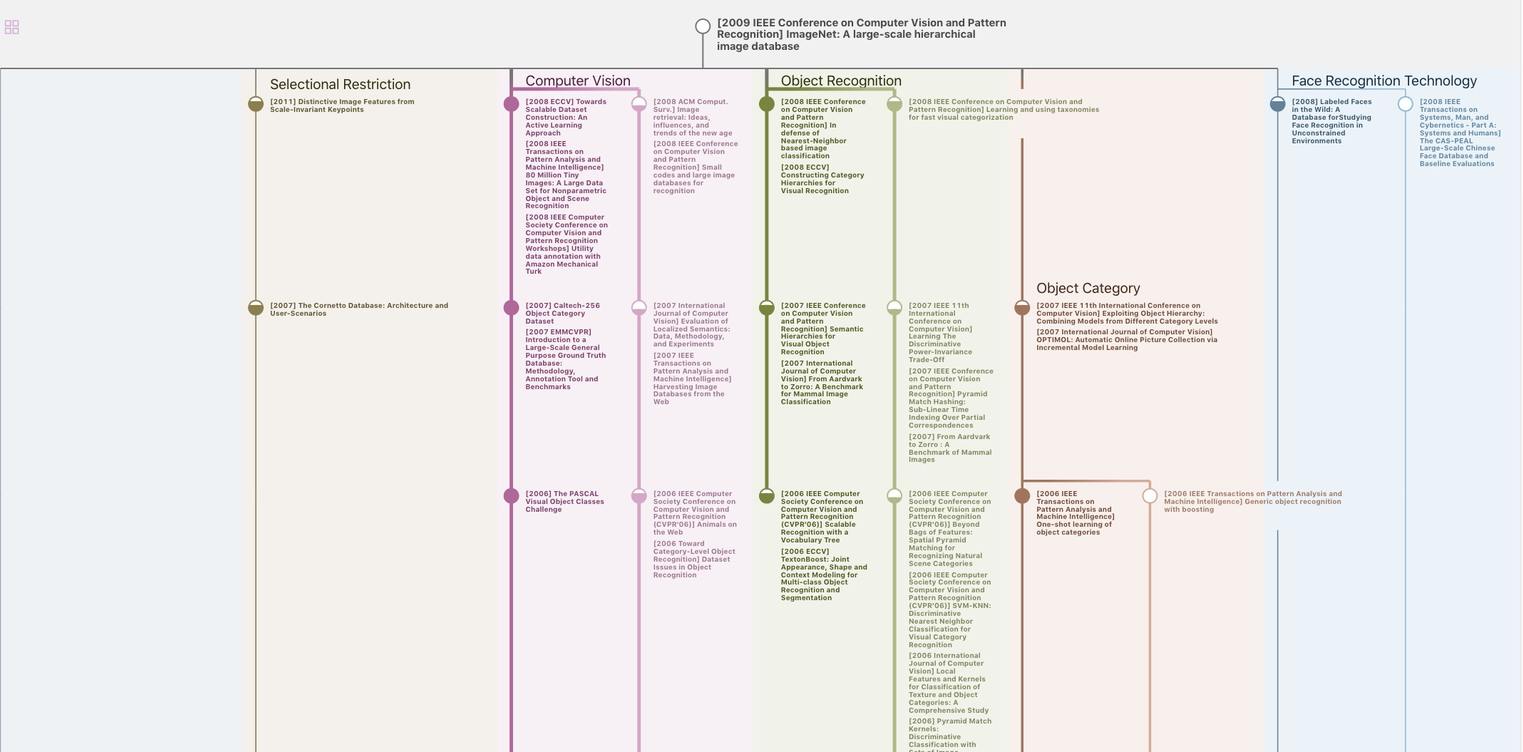
生成溯源树,研究论文发展脉络
Chat Paper
正在生成论文摘要