Globalized distributionally robust optimization with multi core sets
arxiv(2024)
摘要
It is essential to capture the true probability distribution of uncertain
data in the distributionally robust optimization (DRO). The uncertain data
presents multimodality in numerous application scenarios, in the sense that the
probability density function of the uncertain data has two or more modes (local
maximums). In this paper, we propose a globalized distributionally robust
optimization framework with multiple core sets (MGDRO) to handle the multimodal
data. This framework captures the multimodal structure via a penalty function
composed of the minimum distances from the random vector to all core sets.
Under some assumptions, the MGDRO model can be reformulated as tractable
semi-definite programs for both moment-based and metric-based ambiguity sets.
We applied the MGDRO models to a multi-product newswendor problem with
multimodal demands. The numerical results turn out that the MGDRO models
outperform traditional DRO models and other multimodal models greatly.
更多查看译文
AI 理解论文
溯源树
样例
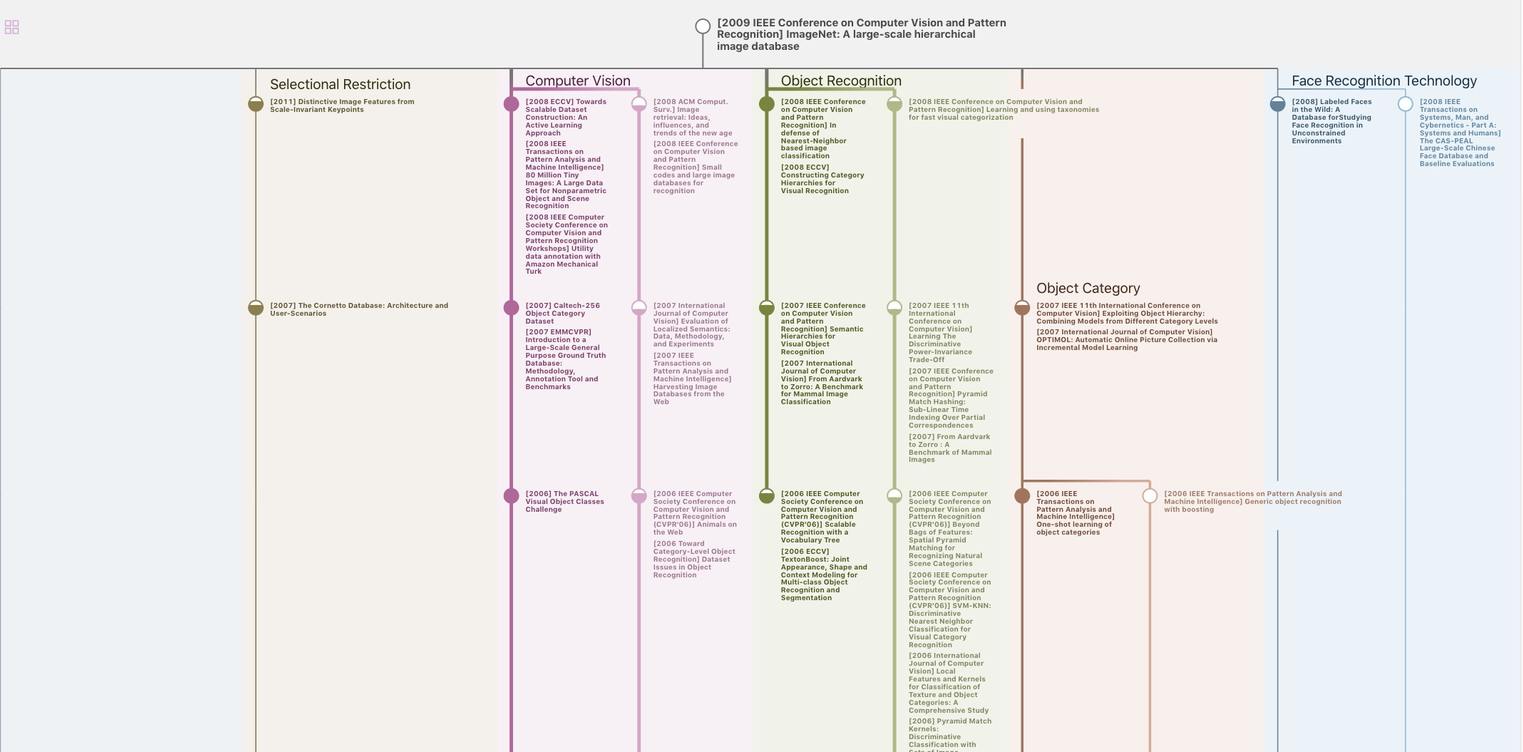
生成溯源树,研究论文发展脉络
Chat Paper
正在生成论文摘要