SPD Manifold Deep Metric Learning for Image Set Classification
IEEE TRANSACTIONS ON NEURAL NETWORKS AND LEARNING SYSTEMS(2024)
摘要
By characterizing each image set as a nonsingular covariance matrix on the symmetric positive definite (SPD) manifold, the approaches of visual content classification with image sets have made impressive progress. However, the key challenge of unhelpfully large intraclass variability and interclass similarity of representations remains open to date. Although, several recent studies have mitigated the two problems by jointly learning the embedding mapping and the similarity metric on the original SPD manifold, their inherent shallow and linear feature transformation mechanism are not powerful enough to capture useful geometric features, especially in complex scenarios. To this end, this article explores a novel approach, termed SPD manifold deep metric learning (SMDML), for image set classification. Specifically, SMDML first selects a prevailing SPD manifold neural network (SPDNet) as the backbone (encoder) to derive an SPD matrix nonlinear representation. To counteract the degradation of structural information during multistage feature embedding, we construct a Riemannian decoder at the end of the encoder, trained by a reconstruction error term (RT), to induce the generated low-dimensional feature manifold of the hidden layer to capture the pivotal information about the visual data describing the imaged scene. We demonstrate through theory and experiments that it is feasible to replace the Riemannian metric with Euclidean distance in RT. Then, the ReCov layer is introduced into the established Riemannian network to regularize the local statistical information within each input feature matrix, which enhances the effectiveness of the learning process. The theoretical analysis of the activation function used in the ReCov layer in terms of continuity and conditions for generating positive definite matrices is beneficial for network design. Inspired by the fact that the single cross-entropy loss used for training is unable to effectively parse the geometric distribution of the deep representations, we finally endow the suggested model with a novel metric learning regularization term. By explicitly incorporating the encoding and processing of the data variations into the network learning process, this term can not only derive a powerful Riemannian representation but also train an effective classifier. The experimental results show the superiority of the proposed approach on three typical visual classification tasks.
更多查看译文
关键词
Training,Image set classification,metric learning,Riemannian neural networks,symmetric positive definite (SPD) manifold
AI 理解论文
溯源树
样例
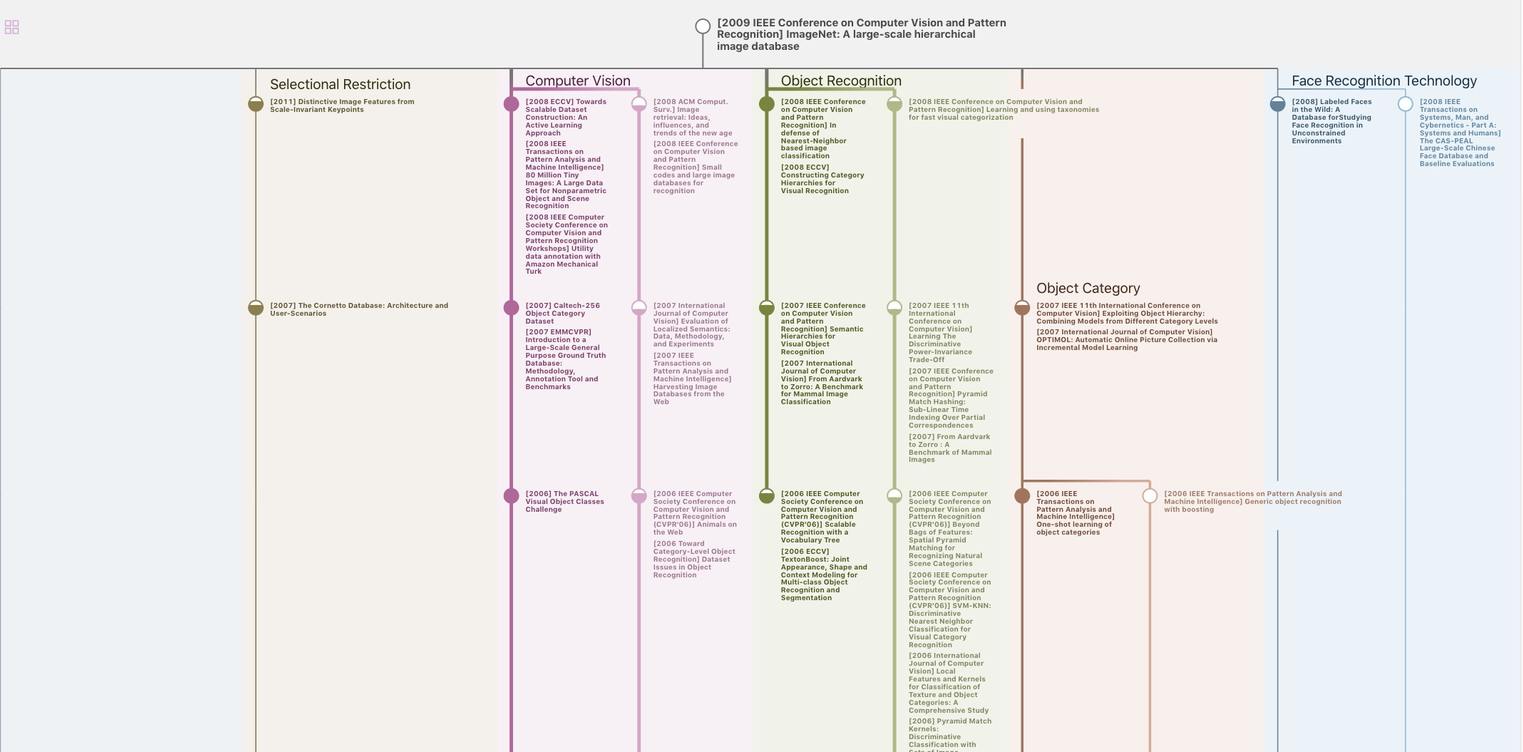
生成溯源树,研究论文发展脉络
Chat Paper
正在生成论文摘要