MM-Net: A MixFormer-Based Multi-Scale Network for Anatomical and Functional Image Fusion.
IEEE transactions on image processing : a publication of the IEEE Signal Processing Society(2024)
摘要
Anatomical and functional image fusion is an important technique in a variety of medical and biological applications. Recently, deep learning (DL)-based methods have become a mainstream direction in the field of multi-modal image fusion. However, existing DL-based fusion approaches have difficulty in effectively capturing local features and global contextual information simultaneously. In addition, the scale diversity of features, which is a crucial issue in image fusion, often lacks adequate attention in most existing works. In this paper, to address the above problems, we propose a MixFormer-based multi-scale network, termed as MM-Net, for anatomical and functional image fusion. In our method, an improved MixFormer-based backbone is introduced to sufficiently extract both local features and global contextual information at multiple scales from the source images. The features from different source images are fused at multiple scales based on a multi-source spatial attention-based cross-modality feature fusion (CMFF) module. The scale diversity of the fused features is further enriched by a series of multi-scale feature interaction (MSFI) modules and feature aggregation upsample (FAU) modules. Moreover, a loss function consisting of both spatial domain and frequency domain components is devised to train the proposed fusion model. Experimental results demonstrate that our method outperforms several state-of-the-art fusion methods on both qualitative and quantitative comparisons, and the proposed fusion model exhibits good generalization capability. The source code of our fusion method will be available at https://github.com/yuliu316316.
更多查看译文
AI 理解论文
溯源树
样例
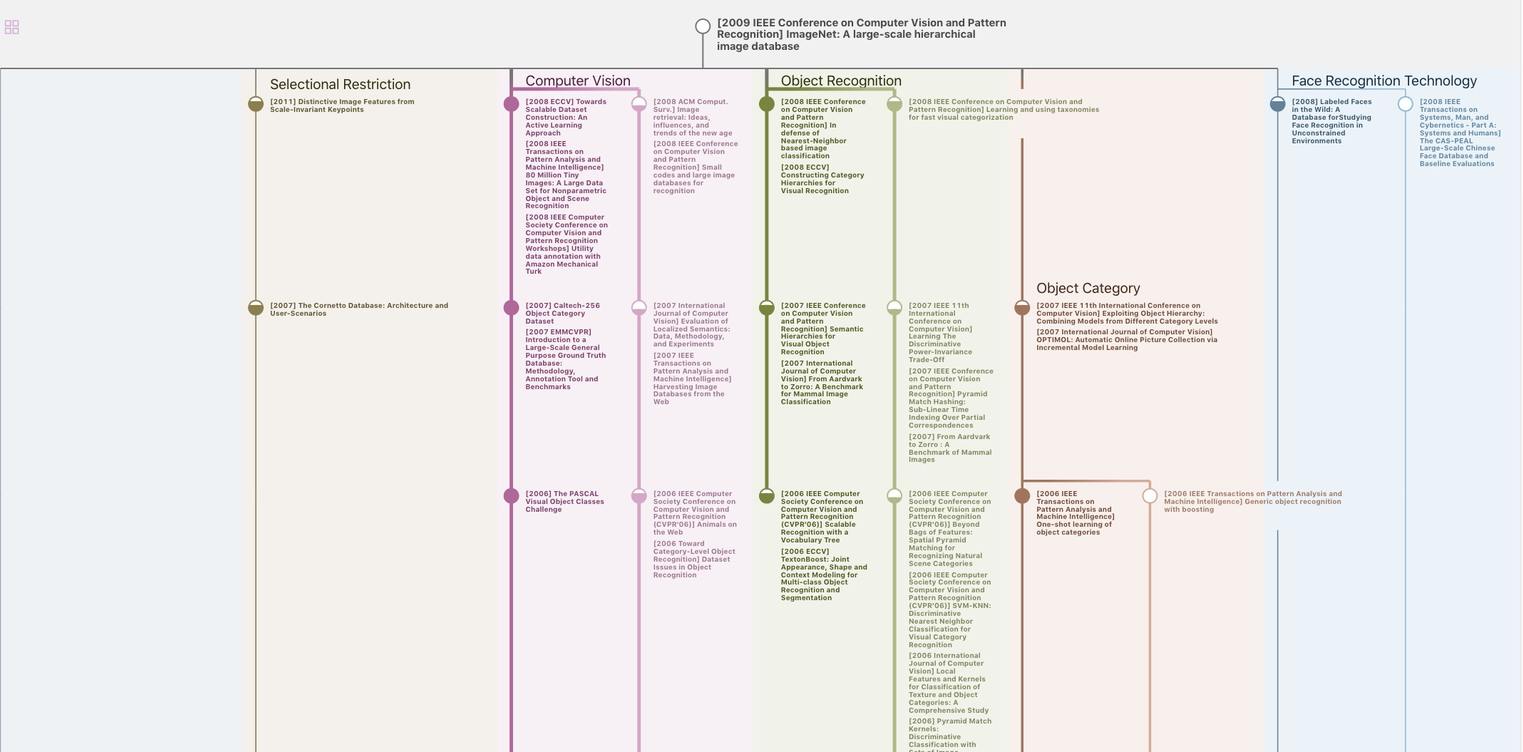
生成溯源树,研究论文发展脉络
Chat Paper
正在生成论文摘要