A high resolution model of the grapevine leaf morphospace predicts synthetic leaves
biorxiv(2024)
摘要
Grapevine leaves are a model morphometric system. Sampling over ten thousand leaves using dozens of landmarks, the genetic, developmental, and environmental basis of leaf shape has been studied and a morphospace for the genus Vitis predicted. Yet, these representations of leaf shape fail to capture the exquisite features of leaves at high resolution. We measure the shapes of 139 grapevine leaves using 1672 pseudo-landmarks derived from 90 homologous landmarks with Procrustean approaches. From hand traces of the vasculature and blade, we have derived a method to automatically detect landmarks and place pseudo-landmarks that results in a high-resolution representation of grapevine leaf shape. Using polynomial models, we create continuous representations of leaf development in 10 Vitis spp. We visualize a high-resolution morphospace in which genetic and developmental sources of leaf shape variance are orthogonal to each other. Using classifiers, V. vinifera, Vitis spp., rootstock and dissected leaf varieties as well as developmental stages are accurately predicted. Theoretical eigenleaf representations sampled from across the morphospace that we call synthetic leaves can be classified using models. By predicting a high-resolution morphospace and delimiting the boundaries of leaf shapes that can plausibly be produced within the genus Vitis, we can sample synthetic leaves with realistic qualities. From an ampelographic perspective, larger numbers of leaves sampled at lower resolution can be projected onto this high-resolution space; or, synthetic leaves can be used to increase the robustness and accuracy of machine learning classifiers.
### Competing Interest Statement
The authors have declared no competing interest.
更多查看译文
AI 理解论文
溯源树
样例
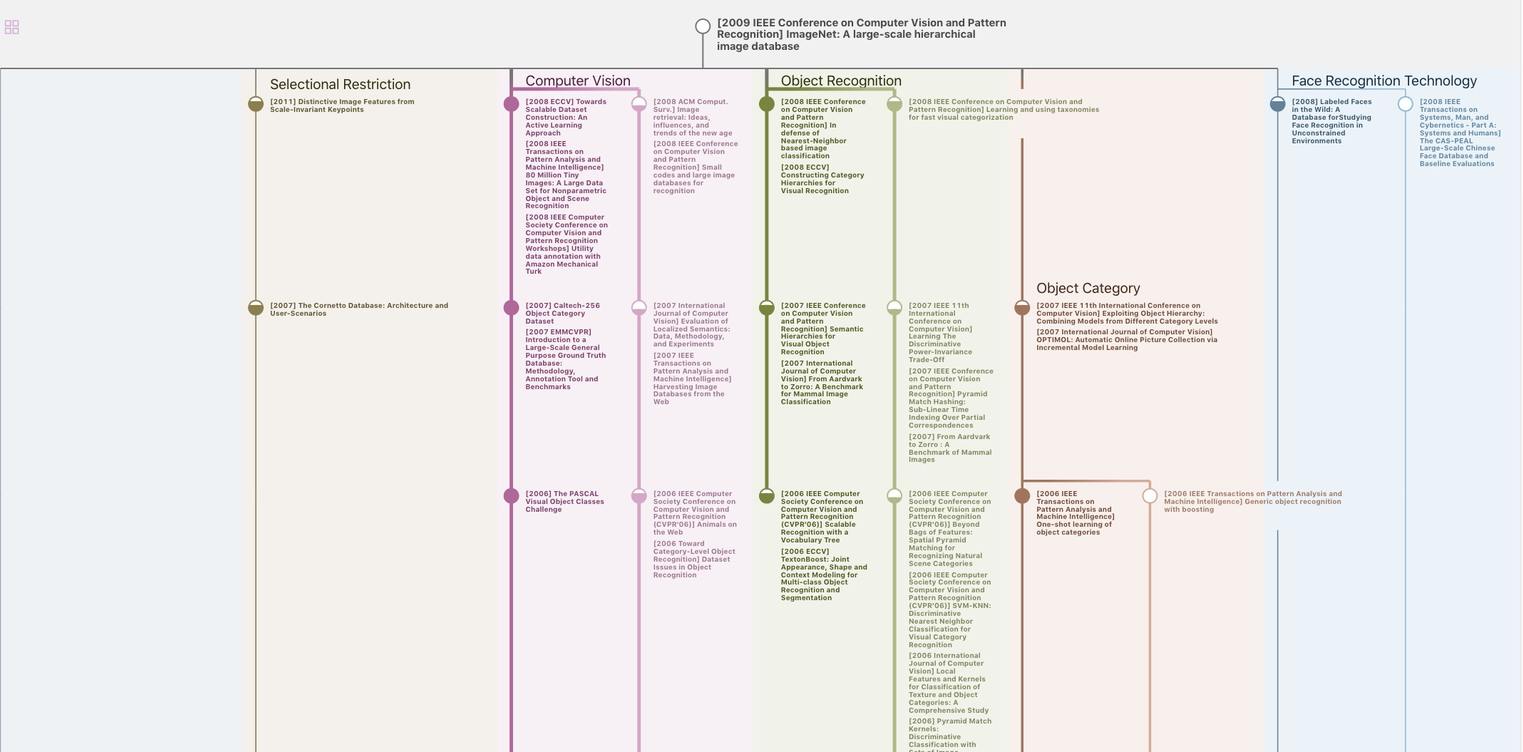
生成溯源树,研究论文发展脉络
Chat Paper
正在生成论文摘要