Time Synchronized Multivariate Regressive Convolution Deep Neural Network Model for Sinkhole Attack Detection in WSN
Wireless Personal Communications(2024)
摘要
As a result of technological growth, WSNs enable creative practical solutions for anything from basic communication to sophisticated applications, which are not confined to a particular domain. In addition to the advances, there is an ongoing issue concerning the frequent threats or attacks on WSNs. The sinkhole attack, which jeopardizes the accuracy and trustworthiness of information in a WSN, is one of the most devastating attacks. Moreover, WSN routing schemes are extremely vulnerable to sinkhole attacks. To address the security challenges faced by WSNs, timely and reliable intrusion detection is of utmost importance. In this paper, a novel intrusion detection scheme, called Time Synchronized Multivariate Regressive Convolution Deep Neural Network Model (TSMR-CDNN), is introduced for sinkhole attack detection. TSMR-CDNN incorporates a reverse time synchronization scheme for its potential to provide precise clock skews and offsets, effectively reducing detection delays. The Broken-stick regression method is applied for the analysis of multivariate data such as clock features, RSSI, LQI, and energy by setting the threshold value, for enhancing its detection capabilities. The steepest gradient function is used to update the weight parameter in order to reduce the false positive rate. This approach minimizes the error rate, resulting in superior classification performance. The experimental results confirm that TSMR-CDNN outperforms the existing methods in terms of various metrics, and demonstrates 5
更多查看译文
关键词
WSN,Sinkhole attack detection,Convolution deep neural network,Reverse time synchronization mechanism,Broken-stick regression
AI 理解论文
溯源树
样例
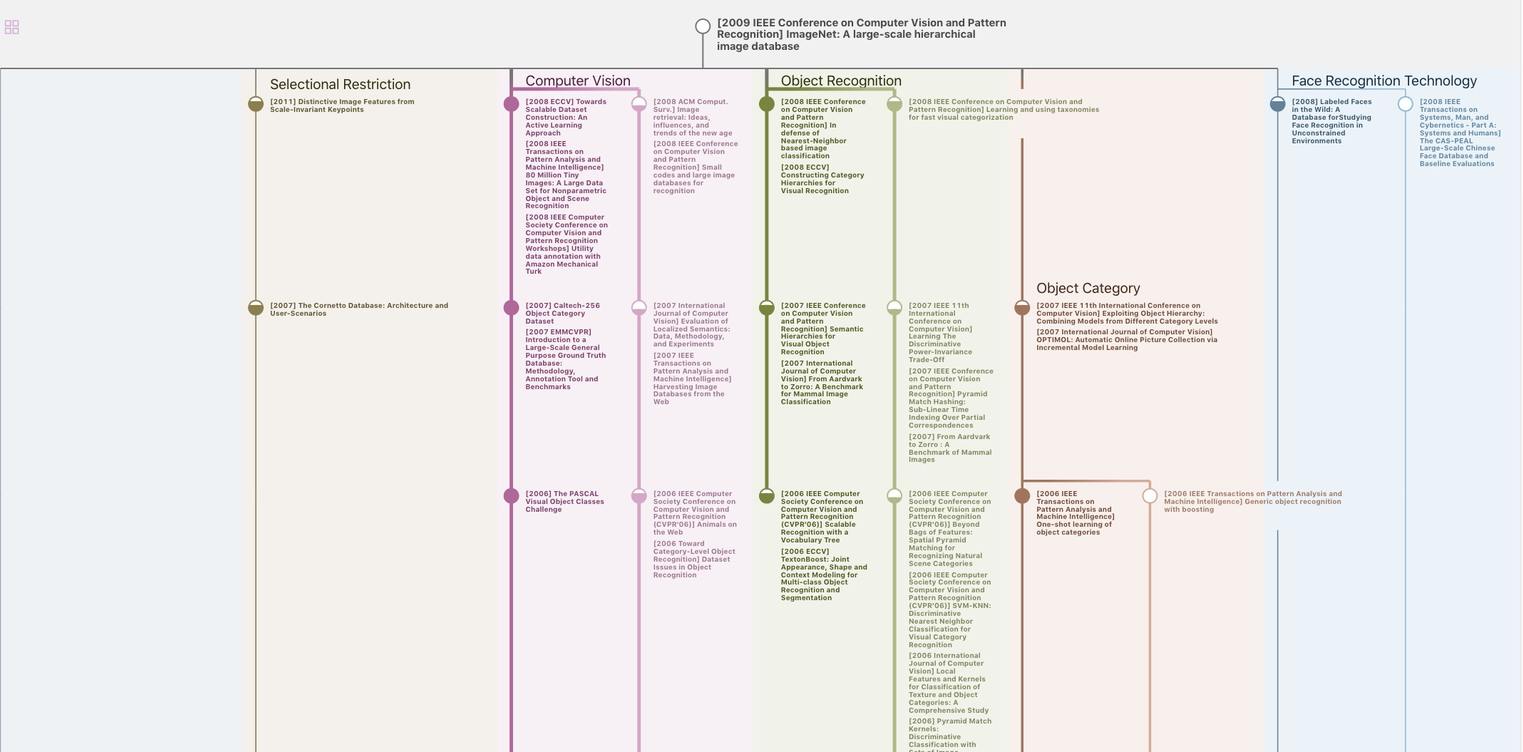
生成溯源树,研究论文发展脉络
Chat Paper
正在生成论文摘要