Causal Representation Learning for GAN-Generated Face Image Quality Assessment
IEEE Transactions on Circuits and Systems for Video Technology(2024)
摘要
Recent years have witnessed significant advancements in face image generation using generative adversarial networks (GANs), leading to a high demand for GAN-generated face image quality assessment (GFIQA). However, the intrinsic distortion caused by the generation brings a significant challenge for existing image quality assessment (IQA) models which are typically designed for natural images. In addition, the image distortion usually varies depending on different GAN models, resulting in a high generalization capability that a GFIQA model should possess. To account for this, we first establish a large GFIQA database by collecting various GFIs from existing popular GAN models. Subsequently, we further propose a causal representation learning (CRL) scheme for the generalized GFIQA model (CRL-GFIQA) with the assumption that the causal knowledge of human quality assessment is shareable in different scenarios. In particular, we disentangle the learned features into casual and non-causal components by an invertible neural network, facilitating the proposed CRL-GFIQA model with a high generalization on unseen domains. Extensive experimental results demonstrate the effectiveness of our CRL-GFIQA model. The codes and the constructed dataset will be publicly available.
更多查看译文
关键词
Face image quality assessment,generative adversarial network,causal representation learning,human visual system
AI 理解论文
溯源树
样例
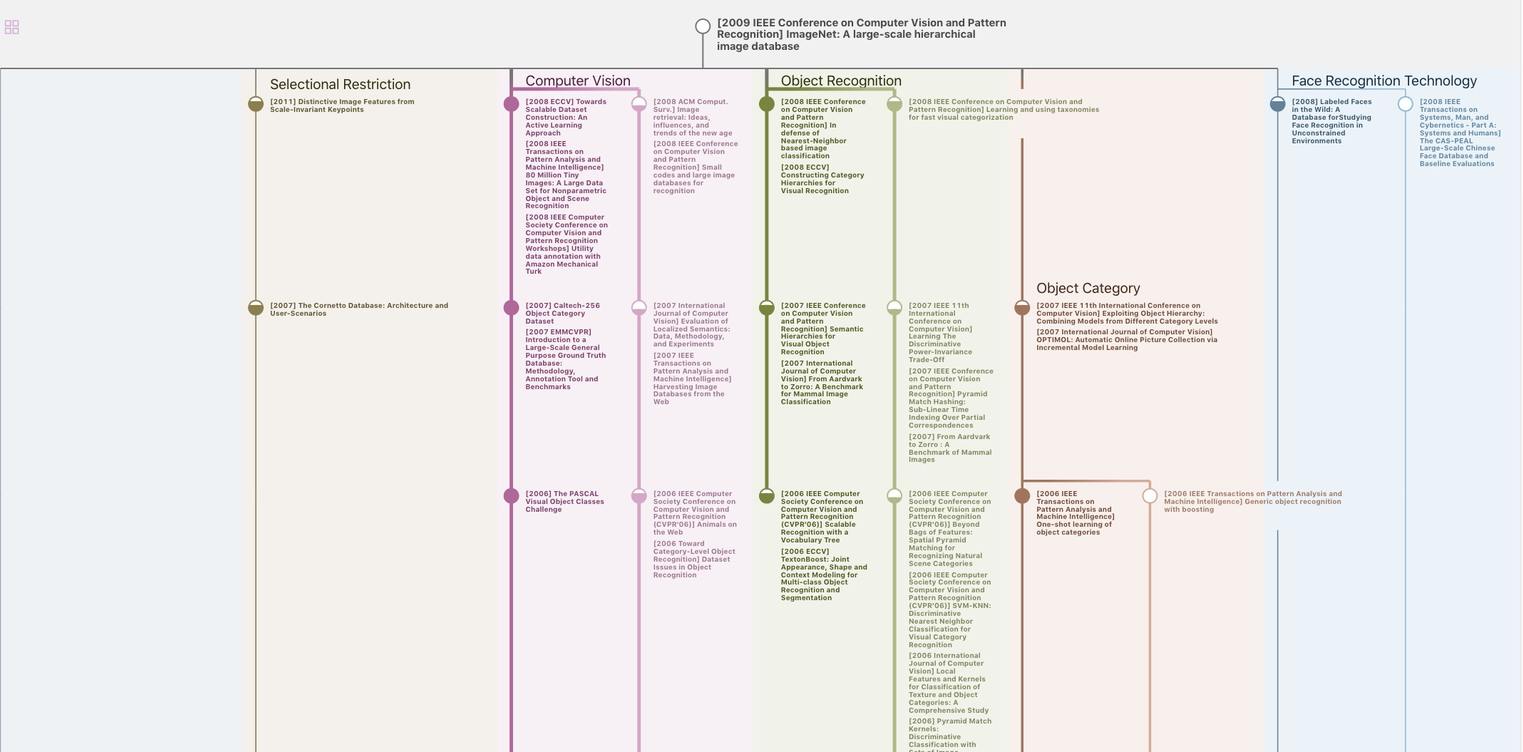
生成溯源树,研究论文发展脉络
Chat Paper
正在生成论文摘要