A Distributed Generative Adversarial Network for Data Augmentation under Vertical Federated Learning
IEEE Transactions on Big Data(2024)
摘要
Vertical federated learning can aggregate participant data features. To address the issue of insufficient overlapping data in vertical federated learning, this study presents a generative adversarial network model that allows distributed data augmentation. First, this study proposes a distributed generative adversarial network FeCGAN for multiple participants with insufficient overlapping data, considering the fact that the generative adversarial network can generate simulation samples. This network is suitable for multiple data sources and can augment participants' local data. Second, to address the problem of learning divergence caused by different local distributions of multiple data sources, this study proposes the aggregation algorithm FedKL. It aggregates the feedback of the local discriminator to interact with the generator and learns the local data distribution more accurately. Finally, given the problem of data waste caused by the unavailability of nonoverlapping data, this study proposes a data augmentation method called VFeDA. It uses FeCGAN to generate pseudo features and expands more overlapping data, thereby improving the data use. Experiments showed that the proposed model is suitable for multiple data sources and can generate high-quality data.
更多查看译文
关键词
Vertical Federated Learning,Data Augmentation,Generative Adversarial Networks,Multiple Data Sources
AI 理解论文
溯源树
样例
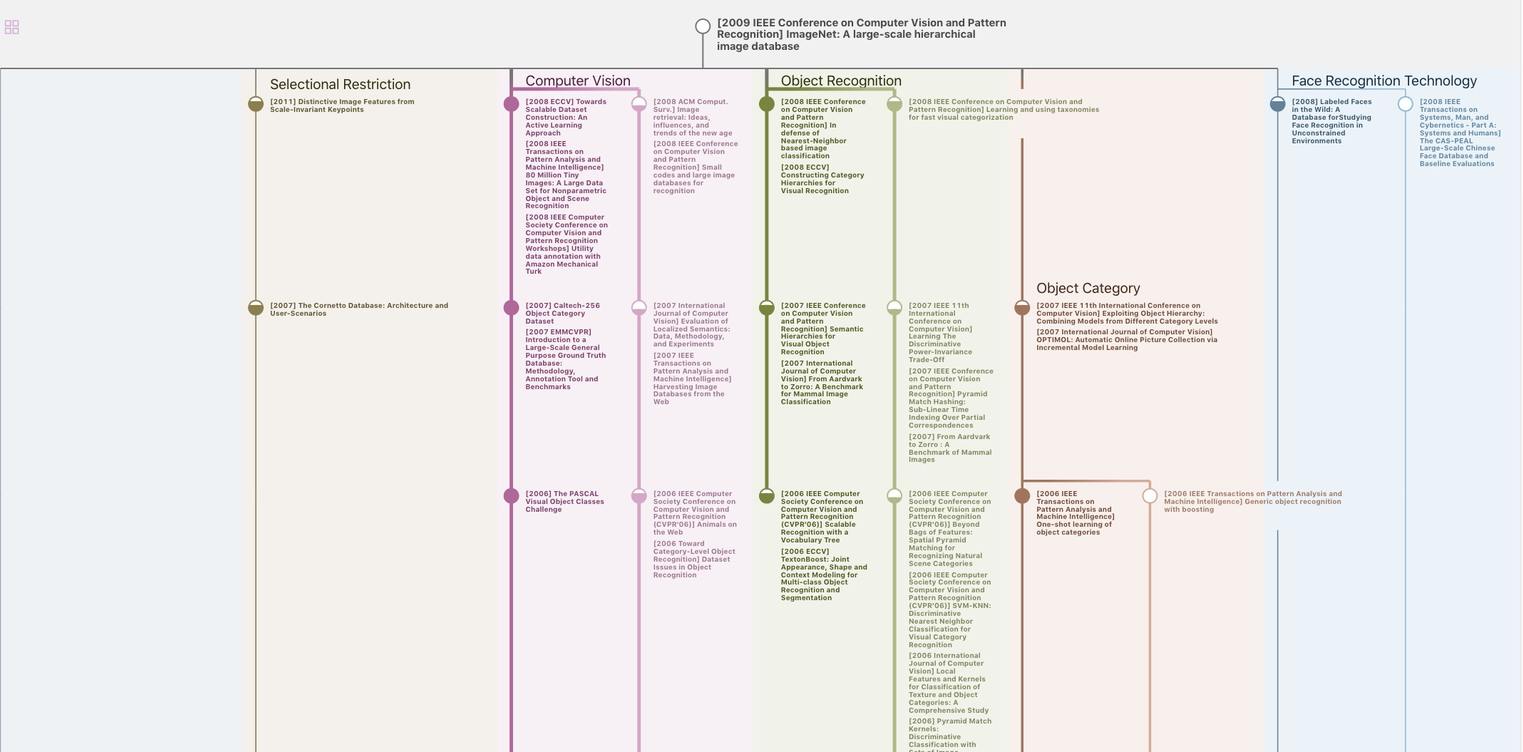
生成溯源树,研究论文发展脉络
Chat Paper
正在生成论文摘要