Cross Hyperspectral and LiDAR Attention Transformer: An Extended Self-Attention for Land Use and Land Cover Classification
IEEE TRANSACTIONS ON GEOSCIENCE AND REMOTE SENSING(2024)
摘要
The successes of attention-driven deep models like the vision transformer (ViT) have sparked interest in cross-domain exploration. However, current transformer-based techniques in remote sensing (RS) primarily focus on single-modal data, limiting their potential to exploit the growing array of multimodal Earth observation (EO) data fully. Enhancing these models for multimodal integration is crucial for comprehensive RS applications. To achieve this, we extend the traditional self-attention mechanism by introducing cross hyperspectral and light detection and ranging (LiDAR) (Cross-HL) attention. We present a novel multimodal deep learning framework that effectively fuses RS data, intending to improve land use and land cover (LULC) recognition. To enhance the accurate exchange of information across different modalities, we fuse their patch projections using the Cross-HL self-attention module. In this process, LiDAR patch tokens serve as queries (Q), while keys (K) and values (V) are derived from HS patch tokens. To demonstrate the superiority of Cross-HL in the proposed multimodal deep learning framework, we conducted extensive experiments on three multimodal RS benchmark datasets: Houston, Trento, and MUUFL. These datasets contain hyperspectral (HS) and LiDAR data. The source code for Cross-HL will be made available publicly at https://github.com/AtriSukul1508/Cross-HL.
更多查看译文
关键词
Convolutional neural networks (CNNs),deep learning,hyperspectral (HS) image classification,vision transformers (ViTs)
AI 理解论文
溯源树
样例
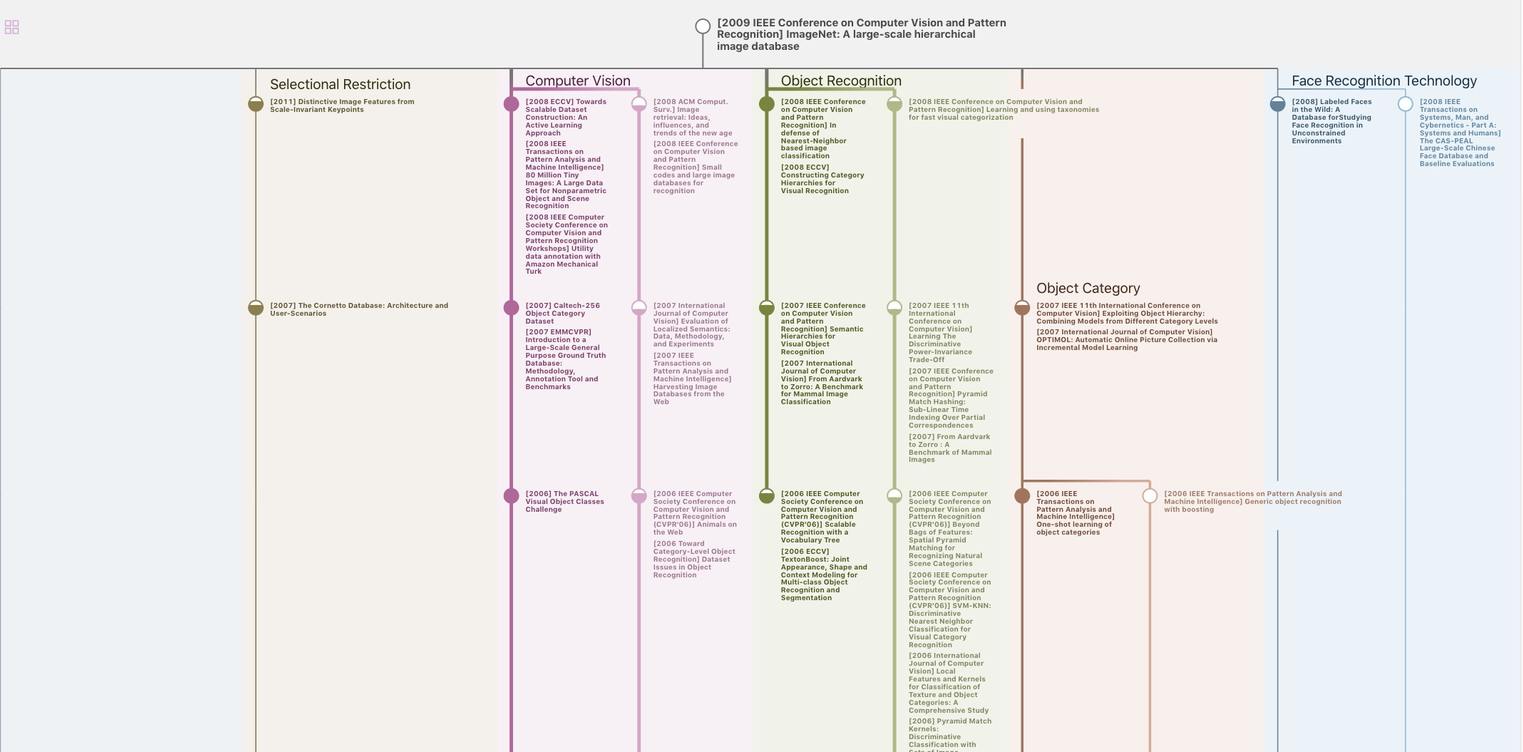
生成溯源树,研究论文发展脉络
Chat Paper
正在生成论文摘要