Dual-path dehazing network with spatial-frequency feature fusion
Pattern Recognition(2024)
摘要
With rapid improvement of deep learning, significant progress has been made in image dehazing, leading to favorable outcomes in many methods. However, a common challenge arises as most of these methods struggle to restore intricate details with vibrant colors in complex haze. In response to this challenge, we present a novel dual-path dehazing network with spatial-frequency feature fusion (DDN-SFF) to remove heterogeneous haze. The proposed dual-path network consists of a spatial-domain vanilla path and a frequency-domain frequency-guided path, effectively harnessing spatial-frequency knowledge. To maximize the versatility of the learned features, we introduce a relaxation dense feature fusion (RDFF) module in the vanilla path. This module can skillfully re-exploit features from non-adjacent levels and concurrently generate new features. In the frequency-guided path, we integrate the discrete wavelet transform (DWT) and introduce a frequency attention (FA) mechanism for the flexible handling of specific channels. More precisely, we deploy a channel attention (CA) and a dense feature fusion (DFF) module for low-frequency channels, whereas a pixel attention (PA) and a residual dense block (RDB) module are implemented for high-frequency channels. In summary, the deep dual-path network fuses sub-bands with specific spatial-frequency features, effectively eliminating the haze and restoring intricate details along with rich textures. Extensive experimental results demonstrate the superior performance of the proposed DDN-SFF over state-of-the-art dehazing algorithms.
更多查看译文
关键词
Image dehazing,Deep learning,Frequency,Convolutional neural network
AI 理解论文
溯源树
样例
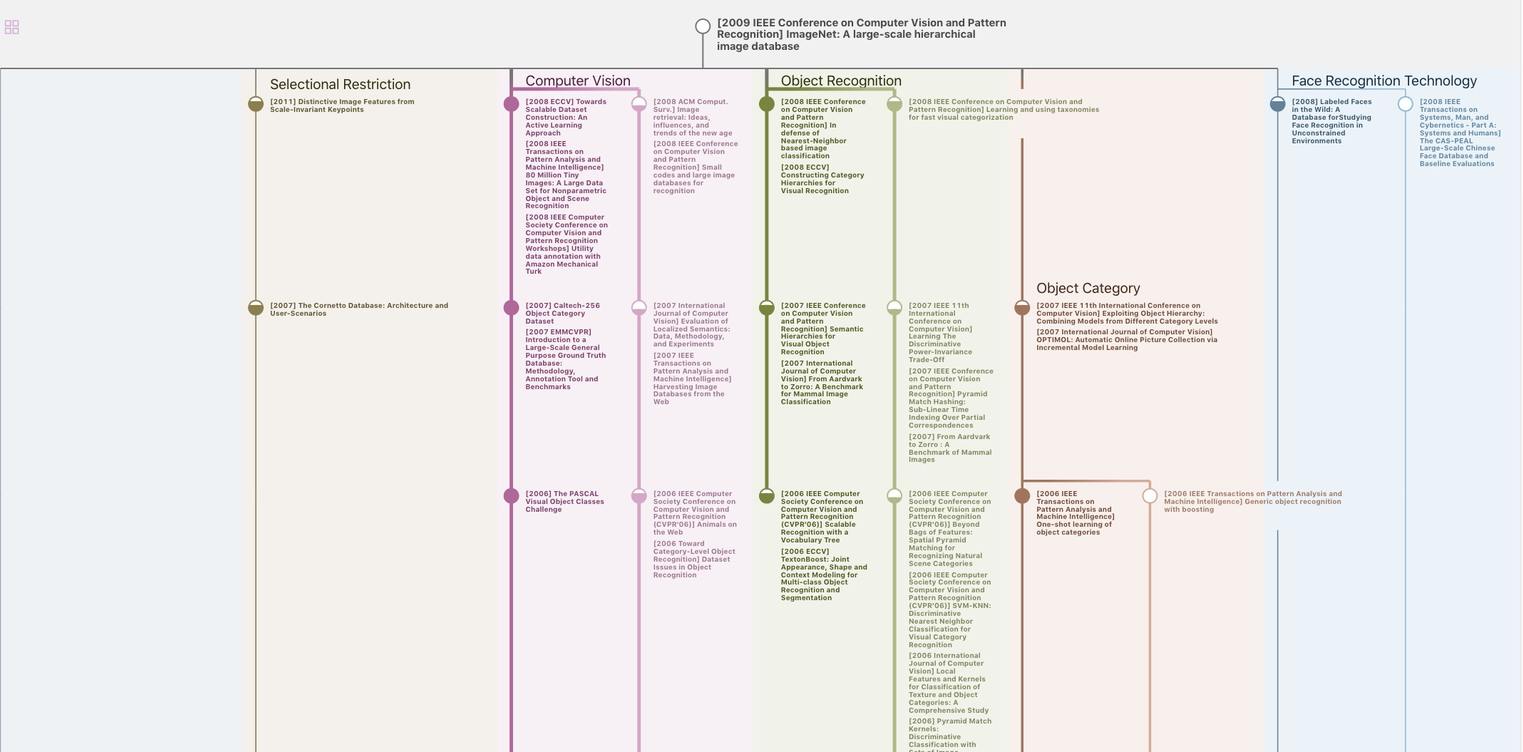
生成溯源树,研究论文发展脉络
Chat Paper
正在生成论文摘要