Radiomics Models Identifying Dementia in Patients with Normal Age-Related Hippocampal Atrophy: A study based on Machine Learning
crossref(2024)
摘要
Abstract Background: Our study aimed to employ a machine learning models based on radiomics for automated dementia identification in cerebral small vessel disease (CSVD) patients with normal age-related hippocampal atrophy (HA). Methods: CSVD patients were divided randomly into training and validation sets in a 6:4 ratio. Bilateral hippocampus segmented based on T2 and FLAIR were used for radiomics. Dementia radiomics models (T2 model, T2FLAIR model, T2-T2FLAIR model) were developed in the training sets and was further verified in the validation sets. The rad_score from the best models, along with clinical factors, was integrated to construct clinic-radiomics models for dementia through multifactor logistic regression analysis. Results: 116 CSVD patients were recruited, 30 patients were dementia and 86 patients were non-dementia. In predicting dementia in the training and validation sets, the area under curves (AUC) were 0.811 and 0.779 for the T2 model, 0.807 and 0.755 for the T2FLAIR model, and 0.864 and 0.801 for the T2-T2FLAIR model. In the overall 116 patients, the AUCs were 0.801 for of the T2 model, 0.783 for the T2FLAIR model, and 0.840 for the T2FLAIR model. No statistical differences were found among the three models. Given the highest AUC value in the T2-FLAIR model, converting the rad-score into binary T2-FLAIR (No, Yes) revealed a correlation between a 'Yes' response and dementia (OR = 13.21, 95% CI 3.487−59.922, p < 0.001) after adjusting for risk factors. Conclusion: Radiomic analysis of the hippocampus in T2-FLAIR images can effectively identify dementia in CSVD patients with normal age-related HA.
更多查看译文
AI 理解论文
溯源树
样例
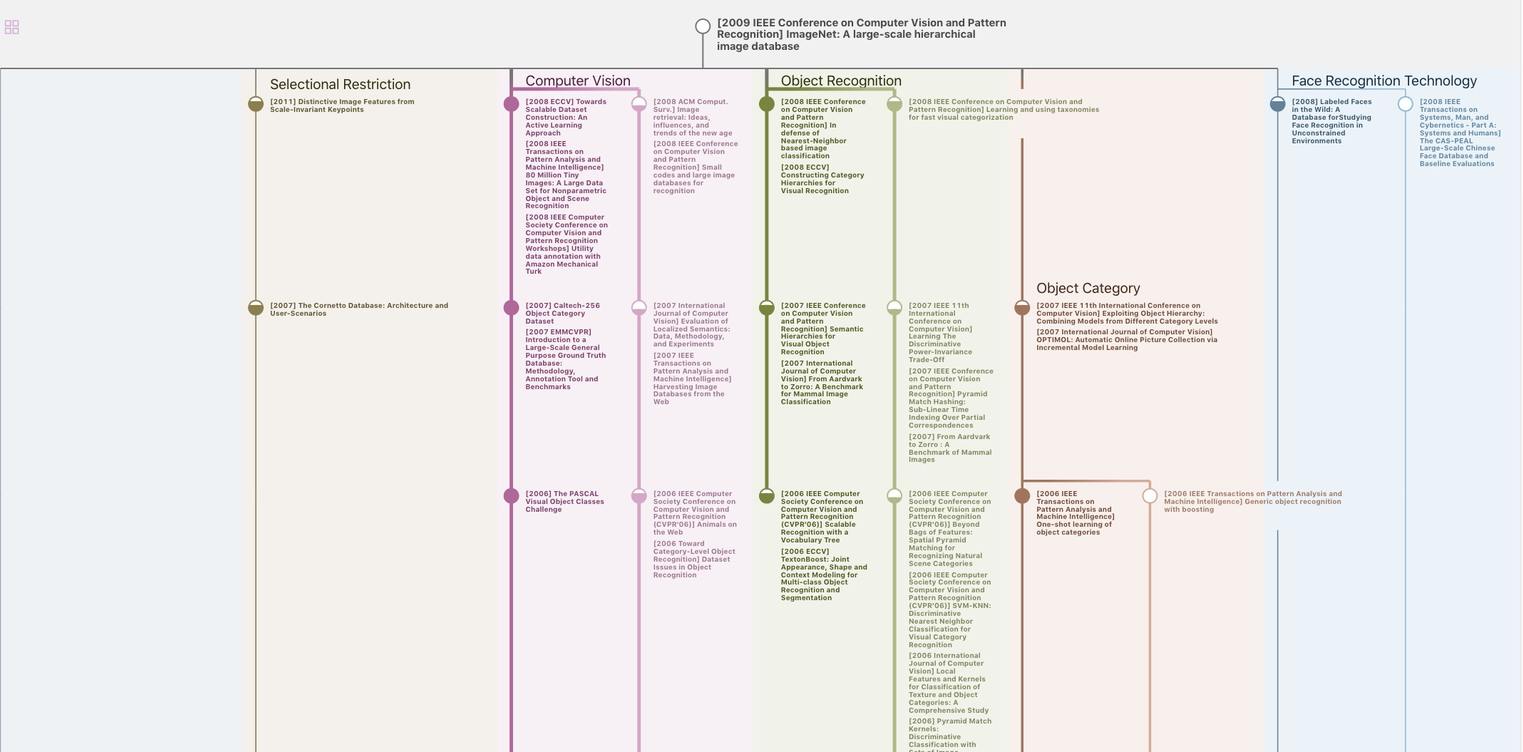
生成溯源树,研究论文发展脉络
Chat Paper
正在生成论文摘要