Reliable prediction of difficult airway for tracheal intubation from patient preoperative photographs by machine learning methods
Computer Methods and Programs in Biomedicine(2024)
摘要
Background
Estimating the risk of a difficult tracheal intubation should help clinicians in better anaesthesia planning, to maximize patient safety. Routine bedside screenings suffer from low sensitivity.
Objective
To develop and evaluate machine learning (ML) and deep learning (DL) algorithms for the reliable prediction of intubation risk, using information about airway morphology.
Methods
Observational, prospective cohort study enrolling n=623 patients who underwent tracheal intubation: 53/623 difficult cases (prevalence 8.51%).First, we used our previously validated deep convolutional neural network (DCNN) to extract 2D image coordinates for 27 + 13 relevant anatomical landmarks in two preoperative photos (frontal and lateral views). Here we propose a method to determine the 3D pose of the camera with respect to the patient and to obtain the 3D world coordinates of these landmarks. Then we compute a novel set of dM=59 morphological features (distances, areas, angles and ratios), engineered with our anaesthesiologists to characterize each individual's airway anatomy towards prediction.Subsequently, here we propose four ad hoc ML pipelines for difficult intubation prognosis, each with four stages: feature scaling, imputation, resampling for imbalanced learning, and binary classification (Logistic Regression, Support Vector Machines, Random Forests and eXtreme Gradient Boosting). These compound ML pipelines were fed with the dM=59 morphological features, alongside dD=7 demographic variables. Here we trained them with automatic hyperparameter tuning (Bayesian search) and probability calibration (Platt scaling). In addition, we developed an ad hoc multi-input DCNN to estimate the intubation risk directly from each pair of photographs, i.e. without any intermediate morphological description.Performance was evaluated using optimal Bayesian decision theory. It was compared against experts' judgement and against state-of-the-art methods (three clinical formulae, four ML, four DL models).
Results
Our four ad hoc ML pipelines with engineered morphological features achieved similar discrimination capabilities: median AUCs between 0.746 and 0.766. They significantly outperformed both expert judgement and all state-of-the-art methods (highest AUC at 0.716). Conversely, our multi-input DCNN yielded low performance due to overfitting. This same behaviour occurred for the state-of-the-art DL algorithms. Overall, the best method was our XGB pipeline, with the fewest false negatives at the optimal Bayesian decision threshold.
Conclusions
We proposed and validated ML models to assist clinicians in anaesthesia planning, providing a reliable calibrated estimate of airway intubation risk, which outperformed expert assessments and state-of-the-art methods. Our novel set of engineered features succeeded in providing informative descriptions for prognosis.
更多查看译文
关键词
Difficult airway,Anaesthesia,Probability calibration,Explainable machine learning,Trustworthy machine learning
AI 理解论文
溯源树
样例
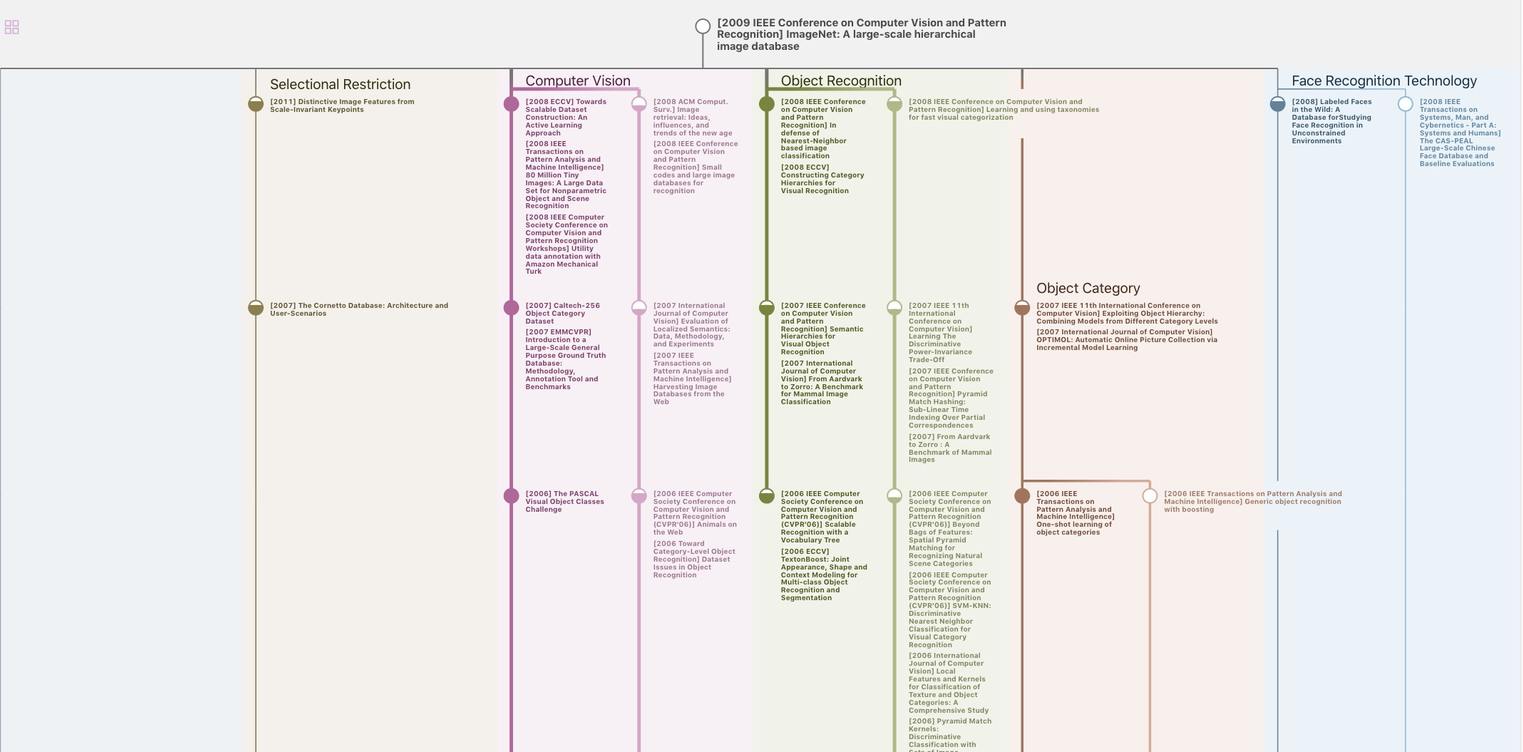
生成溯源树,研究论文发展脉络
Chat Paper
正在生成论文摘要