Value creation and sustainable business model: are ESG ratings a matter of class?
Annals of Operations Research(2024)
摘要
In recent years, sustainable finance has undergone a very consistent expansion boosted by regulatory and cultural changes due to the greater awareness of the positive role of sustainable finance in mitigating the negative externalities of economic activities. In this context, the incorporation of Environmental, Social, and Corporate Governance (ESG) factors into companies’ business models represents an incentive to invest in innovation for growth, attract and cultivate young talents, and seek new strategies to reduce business risks. Hence, the adoption of good ESG practices can be the source of long-term competitive advantages. In the scientific literature, numerous studies try to assess whether the risk-return trade-off of sustainable financial products improves with increasing ESG ratings, but the absence of convergence in the empirical results leaves some open issues that need further investigation. The main goal of this work is to contribute to the debate on the assumption of an existing relationship between ESG compliance and the financial performance of companies. Accepting that ESG ratings are a reliable indicator of ESG practices, we aim to verify if ESG ratings do have informative content useful to predict a company’s future financial performance in terms of: (i) Return on Equity, (ii) Return on Investments, and (iii) systematic risk. We test our hypothesis by using a machine-learning approach. By analyzing the set of EuroStoXX600 Index companies for the period 2016–2021 via Random Forest and Sliding Windows Random Forest Models, we verify whether the integration of ESG criteria into corporate businesses helps to explain the financial performance of listed companies over time and, thus, to identify a strategic tool for all investors.
更多查看译文
关键词
Machine learning,Dynamic random forest,ESG risks,Risk-return profile,Forecasting,Variables selection,G11,G17,G32,C53
AI 理解论文
溯源树
样例
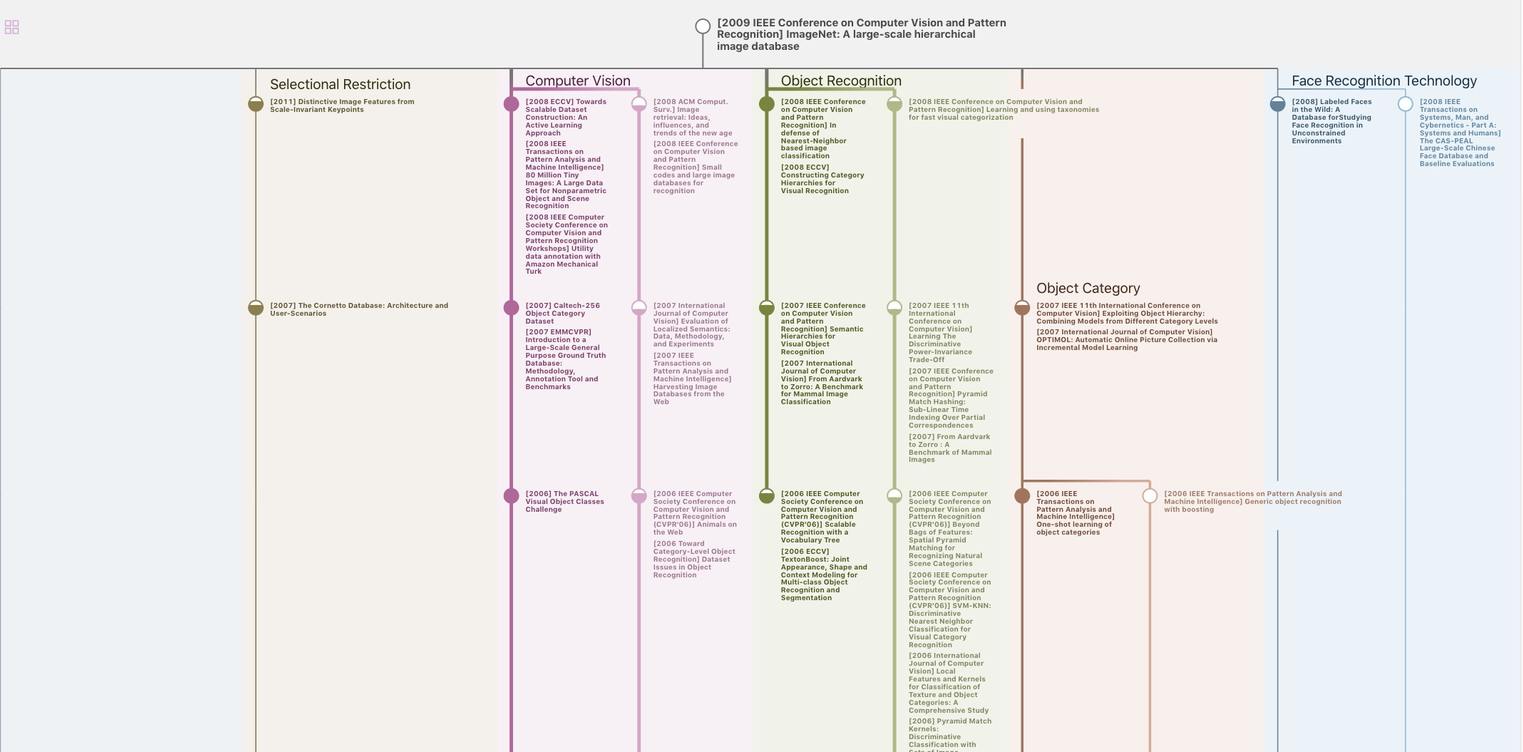
生成溯源树,研究论文发展脉络
Chat Paper
正在生成论文摘要