A Distributed Bayesian Data Fusion Algorithm with Uniform Consistency
IEEE Transactions on Automatic Control(2024)
摘要
Distributed Data Fusion (DDF) methods which possess guaranteed performance for ad-hoc and arbitrarily connected networks empower more scalable, flexible and robust information fusion for multi-robot sensor networks. This paper proposes a novel distributed Bayesian data fusion algorithm, which ensures uniform consistency, i.e., all the locally estimated distributions converge to the true distribution, for arbitrary periodically connected communication graphs. Conservative fusion via the Weighted Exponential Product (WEP) rule is utilized to combat inconsistencies that arise from double-counting common information between fusion agents, and the WEP fusion weight is chosen based on the dynamic communication network topology. The uniform consistency of the proposed algorithm is rigorously proved, and the cooperative consistency conditions that guarantee uniform consistency have been explicitly identified. The performance and convergence properties of the proposed algorithm are validated through simulations.
更多查看译文
关键词
Bayesian Learning,Bayesian Consistency,Distributed Data Fusion
AI 理解论文
溯源树
样例
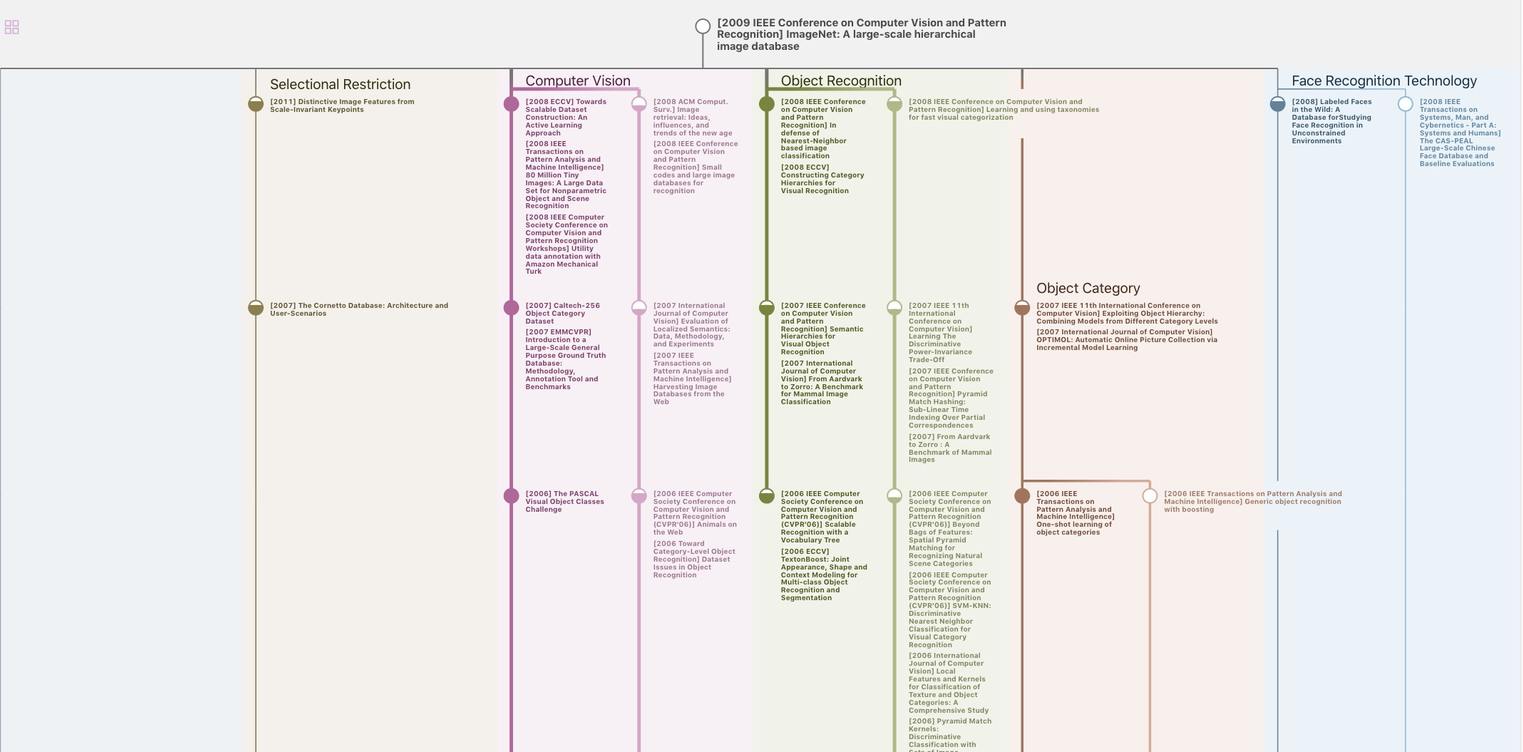
生成溯源树,研究论文发展脉络
Chat Paper
正在生成论文摘要