A Task-Adaptive In-Situ ReRAM Computing for Graph Convolutional Networks
IEEE Transactions on Computer-Aided Design of Integrated Circuits and Systems(2024)
摘要
ReRAM-based Computing-in-Memory (CiM) architecture has been considered a promising solution to high-efficiency neural network accelerator, by conducting in-situ matrix multiplications and eliminating the movement of neural parameters from off-chip memory to computing units. However, we observed specific features of Graph Convolutional Network (GCN) tasks pose design challenges to implement a high-efficiency ReRAM GCN accelerator. The ultra-large input feature data in some GCN tasks incur massive data movements, the extremely sparse adjacency matrix and input feature data involve the valid computation, and the super-large adjacency matrix that exceeds available ReRAM capacity causes frequent expensive write operations. To address the above challenges, we propose TARe, a Task-Adaptive CiM architecture, which consists of a hybrid in-situ computing mode to support the input feature in crossbar computing, a compact mapping scheme for efficient sparse matrix computing, and a write-free mapping to eliminate write activities in the computations with the super-large adjacency matrix. Additionally, TARe is facilitated with a task adaptive selection algorithm to generate optimized design schemes for graph neural network tasks that have various operand sizes and data sparsity. We evaluate TARe on 11 diverse graph neural network tasks and compare it with different design counterparts, and the results show that achieves 168.06× speedup and 10.95× energy consumption reduction on average over the baseline in common graph convolutional network workloads.
更多查看译文
AI 理解论文
溯源树
样例
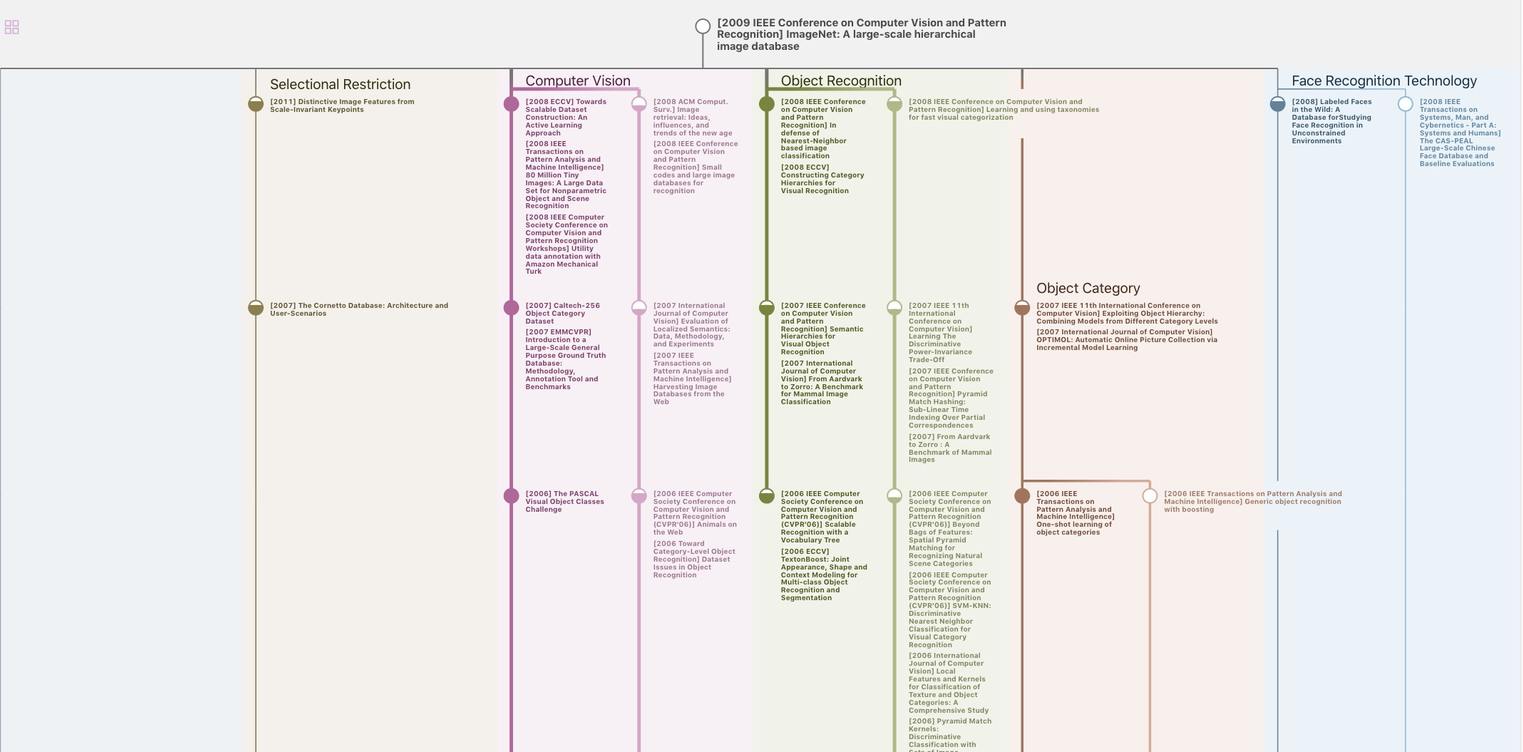
生成溯源树,研究论文发展脉络
Chat Paper
正在生成论文摘要