Premonition: Using Generative Models to Preempt Future Data Changes in Continual Learning
arxiv(2024)
摘要
Continual learning requires a model to adapt to ongoing changes in the data
distribution, and often to the set of tasks to be performed. It is rare,
however, that the data and task changes are completely unpredictable. Given a
description of an overarching goal or data theme, which we call a realm, humans
can often guess what concepts are associated with it. We show here that the
combination of a large language model and an image generation model can
similarly provide useful premonitions as to how a continual learning challenge
might develop over time. We use the large language model to generate text
descriptions of semantically related classes that might potentially appear in
the data stream in future. These descriptions are then rendered using Stable
Diffusion to generate new labelled image samples. The resulting synthetic
dataset is employed for supervised pre-training, but is discarded prior to
commencing continual learning, along with the pre-training classification head.
We find that the backbone of our pre-trained networks can learn representations
useful for the downstream continual learning problem, thus becoming a valuable
input to any existing continual learning method. Although there are
complexities arising from the domain gap between real and synthetic images, we
show that pre-training models in this manner improves multiple Class Incremenal
Learning (CIL) methods on fine-grained image classification benchmarks.
Supporting code can be found at https://github.com/cl-premonition/premonition.
更多查看译文
AI 理解论文
溯源树
样例
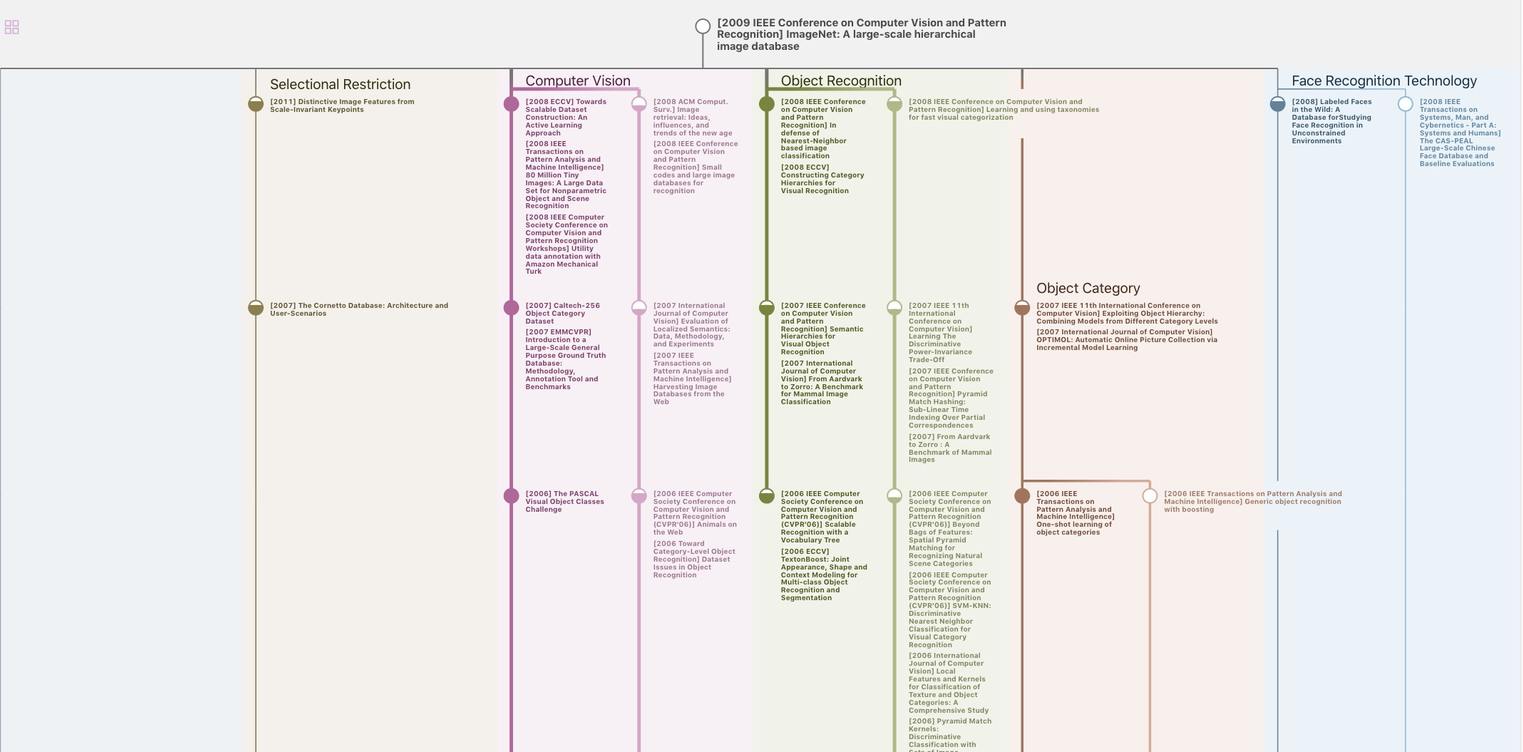
生成溯源树,研究论文发展脉络
Chat Paper
正在生成论文摘要