Large Window-based Mamba UNet for Medical Image Segmentation: Beyond Convolution and Self-attention
arxiv(2024)
摘要
In clinical practice, medical image segmentation provides useful information
on the contours and dimensions of target organs or tissues, facilitating
improved diagnosis, analysis, and treatment. In the past few years,
convolutional neural networks (CNNs) and Transformers have dominated this area,
but they still suffer from either limited receptive fields or costly long-range
modeling. Mamba, a State Space Sequence Model (SSM), recently emerged as a
promising paradigm for long-range dependency modeling with linear complexity.
In this paper, we introduce a Large Window-based Mamba U}-shape Network, or
LMa-UNet, for 2D and 3D medical image segmentation. A distinguishing feature of
our LMa-UNet is its utilization of large windows, excelling in locally spatial
modeling compared to small kernel-based CNNs and small window-based
Transformers, while maintaining superior efficiency in global modeling compared
to self-attention with quadratic complexity. Additionally, we design a novel
hierarchical and bidirectional Mamba block to further enhance the global and
neighborhood spatial modeling capability of Mamba. Comprehensive experiments
demonstrate the effectiveness and efficiency of our method and the feasibility
of using large window size to achieve large receptive fields. Codes are
available at https://github.com/wjh892521292/LMa-UNet.
更多查看译文
AI 理解论文
溯源树
样例
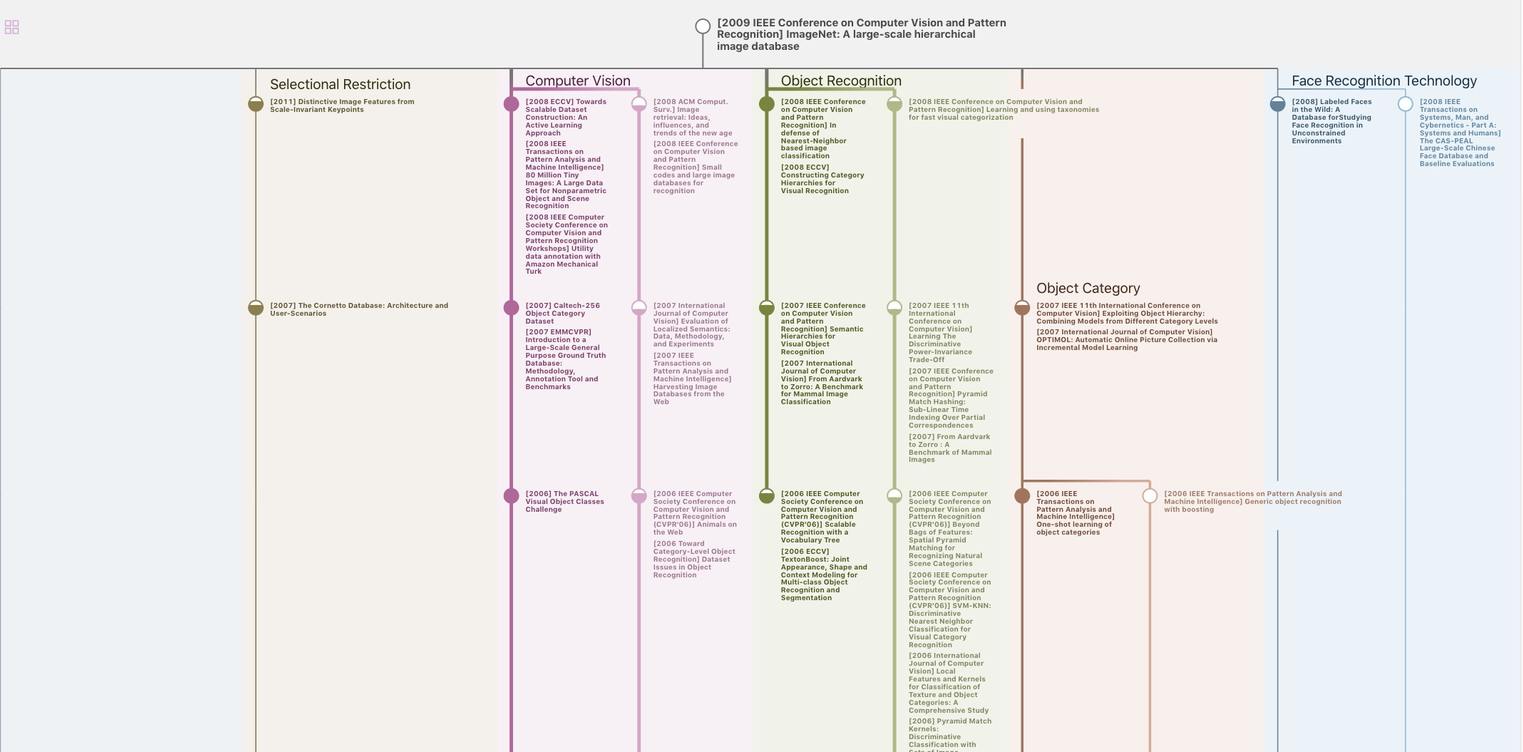
生成溯源树,研究论文发展脉络
Chat Paper
正在生成论文摘要