Graph Data Condensation via Self-expressive Graph Structure Reconstruction
CoRR(2024)
摘要
With the increasing demands of training graph neural networks (GNNs) on
large-scale graphs, graph data condensation has emerged as a critical technique
to relieve the storage and time costs during the training phase. It aims to
condense the original large-scale graph to a much smaller synthetic graph while
preserving the essential information necessary for efficiently training a
downstream GNN. However, existing methods concentrate either on optimizing node
features exclusively or endeavor to independently learn node features and the
graph structure generator. They could not explicitly leverage the information
of the original graph structure and failed to construct an interpretable graph
structure for the synthetic dataset. To address these issues, we introduce a
novel framework named Graph Data Condensation via
Self-expressive Graph Structure Reconstruction
(GCSR). Our method stands out by (1) explicitly incorporating the
original graph structure into the condensing process and (2) capturing the
nuanced interdependencies between the condensed nodes by reconstructing an
interpretable self-expressive graph structure. Extensive experiments and
comprehensive analysis validate the efficacy of the proposed method across
diverse GNN models and datasets. Our code is available at
https://www.dropbox.com/scl/fi/2aonyp5ln5gisdqtjimu8/GCSR.zip?rlkey=11cuwfpsf54wxiiktu0klud0x dl=0
更多查看译文
AI 理解论文
溯源树
样例
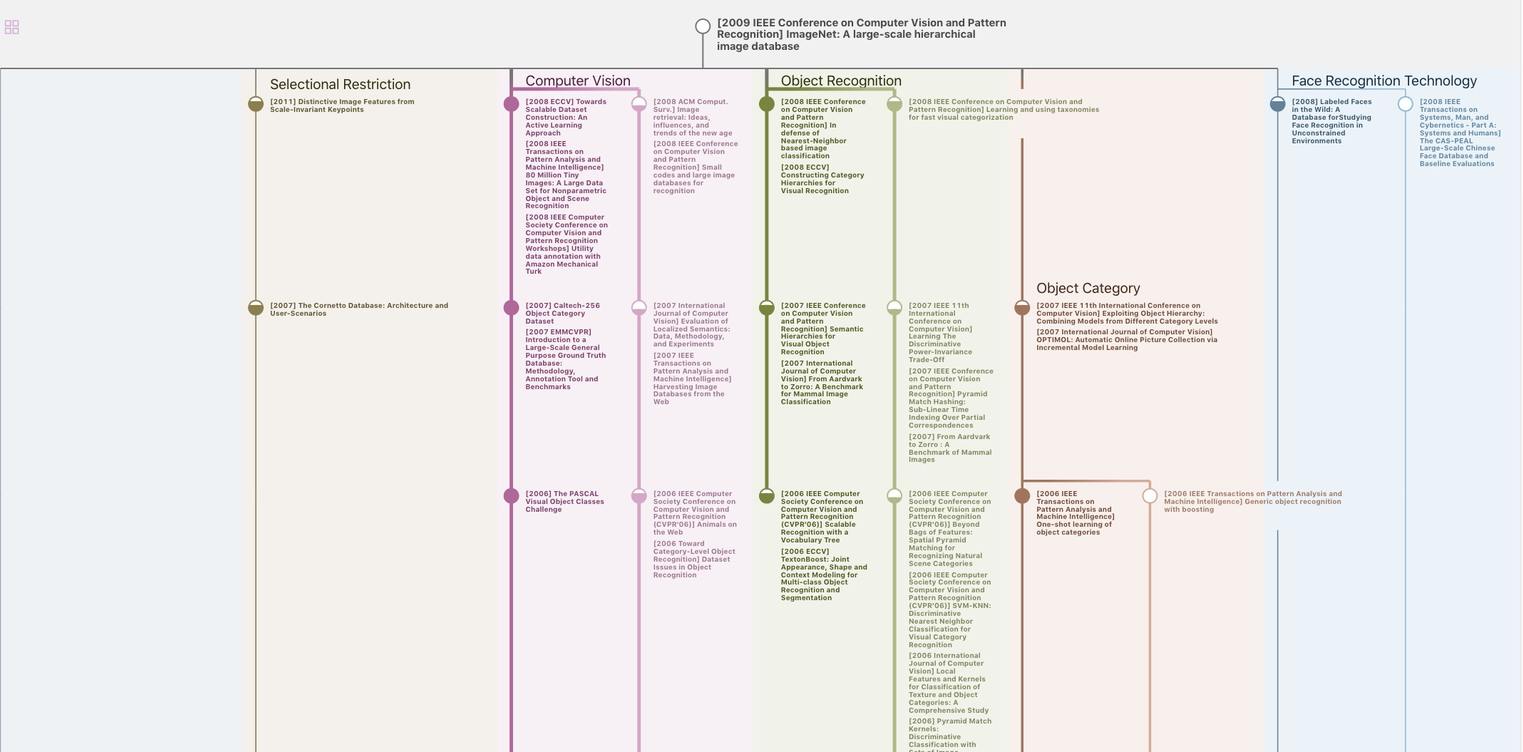
生成溯源树,研究论文发展脉络
Chat Paper
正在生成论文摘要