Efficient and Model-Agnostic Parameter Estimation Under Privacy-Preserving Post-randomization Data
arxiv(2024)
摘要
Protecting individual privacy is crucial when releasing sensitive data for
public use. While data de-identification helps, it is not enough. This paper
addresses parameter estimation in scenarios where data are perturbed using the
Post-Randomization Method (PRAM) to enhance privacy. Existing methods for
parameter estimation under PRAM data suffer from limitations like being
parameter-specific, model-dependent, and lacking efficiency guarantees. We
propose a novel, efficient method that overcomes these limitations. Our method
is applicable to general parameters defined through estimating equations and
makes no assumptions about the underlying data model. We further prove that the
proposed estimator achieves the semiparametric efficiency bound, making it
optimal in terms of asymptotic variance.
更多查看译文
AI 理解论文
溯源树
样例
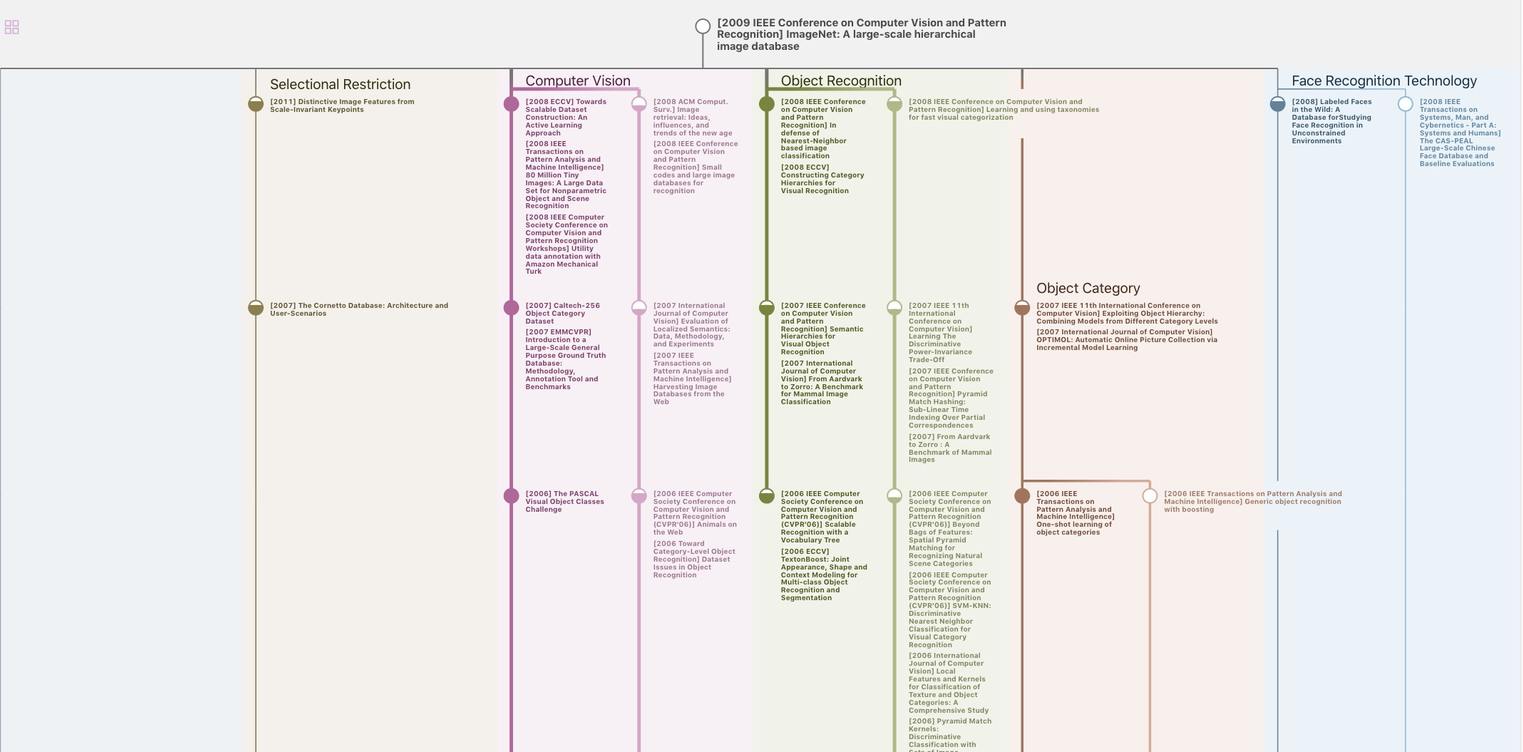
生成溯源树,研究论文发展脉络
Chat Paper
正在生成论文摘要