Self-supervised Contrastive Learning for Implicit Collaborative Filtering
arxiv(2024)
摘要
Contrastive learning-based recommendation algorithms have significantly
advanced the field of self-supervised recommendation, particularly with BPR as
a representative ranking prediction task that dominates implicit collaborative
filtering. However, the presence of false-positive and false-negative examples
in recommendation systems hampers accurate preference learning. In this study,
we propose a simple self-supervised contrastive learning framework that
leverages positive feature augmentation and negative label augmentation to
improve the self-supervisory signal. Theoretical analysis demonstrates that our
learning method is equivalent to maximizing the likelihood estimation with
latent variables representing user interest centers. Additionally, we establish
an efficient negative label augmentation technique that samples unlabeled
examples with a probability linearly dependent on their relative ranking
positions, enabling efficient augmentation in constant time complexity. Through
validation on multiple datasets, we illustrate the significant improvements our
method achieves over the widely used BPR optimization objective while
maintaining comparable runtime.
更多查看译文
AI 理解论文
溯源树
样例
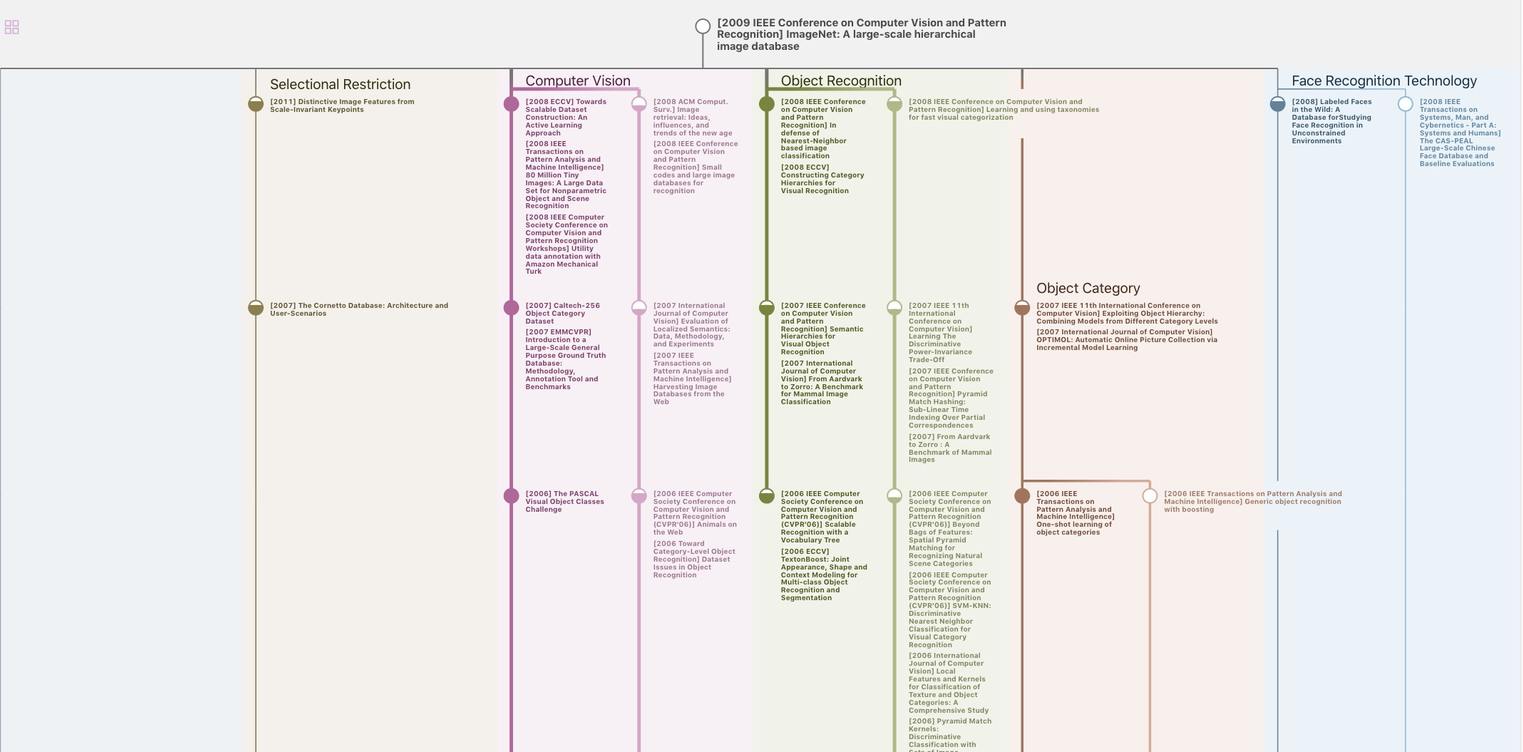
生成溯源树,研究论文发展脉络
Chat Paper
正在生成论文摘要