Near-Interpolators: Rapid Norm Growth and the Trade-Off between Interpolation and Generalization
arxiv(2024)
摘要
We study the generalization capability of nearly-interpolating linear
regressors: β's whose training error τ is positive but
small, i.e., below the noise floor. Under a random matrix theoretic assumption
on the data distribution and an eigendecay assumption on the data covariance
matrix Σ, we demonstrate that any near-interpolator
exhibits rapid norm growth: for τ fixed, β has squared
ℓ_2-norm 𝔼[β_2^2] =
Ω(n^α) where n is the number of samples and α >1 is the
exponent of the eigendecay, i.e., λ_i(Σ) ∼
i^-α. This implies that existing data-independent norm-based bounds are
necessarily loose. On the other hand, in the same regime we precisely
characterize the asymptotic trade-off between interpolation and generalization.
Our characterization reveals that larger norm scaling exponents α
correspond to worse trade-offs between interpolation and generalization. We
verify empirically that a similar phenomenon holds for nearly-interpolating
shallow neural networks.
更多查看译文
AI 理解论文
溯源树
样例
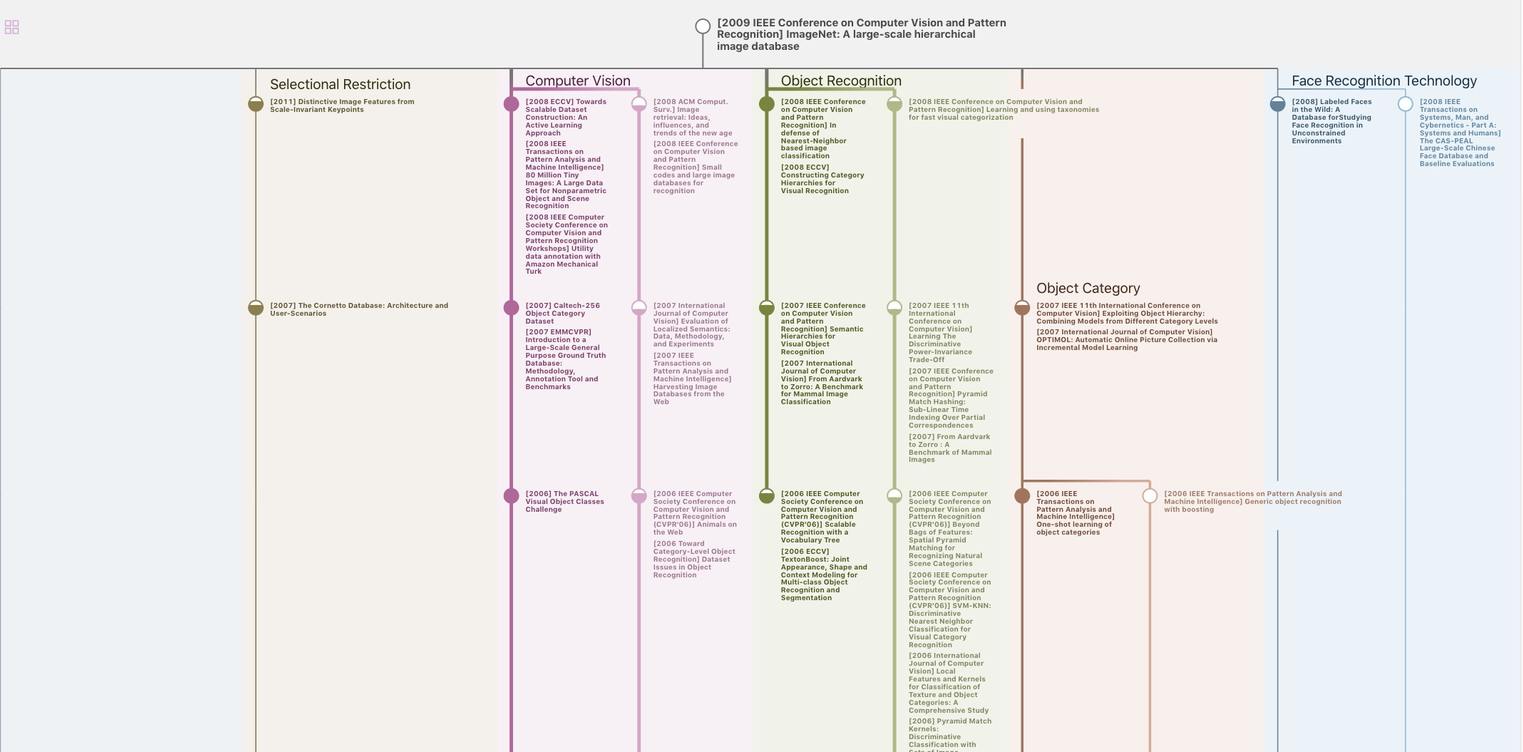
生成溯源树,研究论文发展脉络
Chat Paper
正在生成论文摘要