(𝐍,𝐊)-Puzzle: A Cost-Efficient Testbed for Benchmarking Reinforcement Learning Algorithms in Generative Language Model
arxiv(2024)
摘要
Recent advances in reinforcement learning (RL) algorithms aim to enhance the
performance of language models at scale. Yet, there is a noticeable absence of
a cost-effective and standardized testbed tailored to evaluating and comparing
these algorithms. To bridge this gap, we present a generalized version of the
24-Puzzle: the (N,K)-Puzzle, which challenges language models to reach a
target value K with N integers. We evaluate the effectiveness of
established RL algorithms such as Proximal Policy Optimization (PPO), alongside
novel approaches like Identity Policy Optimization (IPO) and Direct Policy
Optimization (DPO).
更多查看译文
AI 理解论文
溯源树
样例
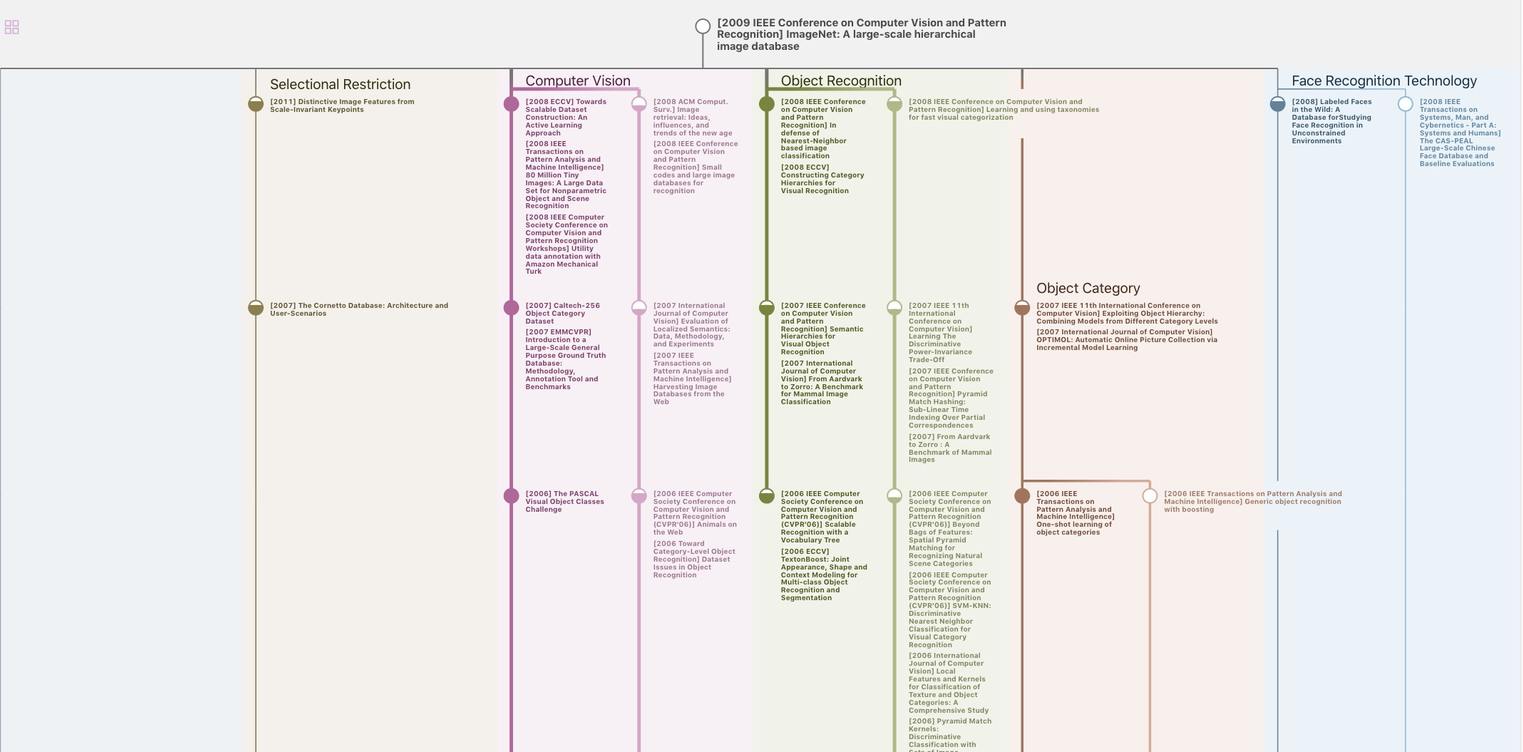
生成溯源树,研究论文发展脉络
Chat Paper
正在生成论文摘要