An Efficient Learning-based Solver Comparable to Metaheuristics for the Capacitated Arc Routing Problem
CoRR(2024)
摘要
Recently, neural networks (NN) have made great strides in combinatorial
optimization. However, they face challenges when solving the capacitated arc
routing problem (CARP) which is to find the minimum-cost tour covering all
required edges on a graph, while within capacity constraints. In tackling CARP,
NN-based approaches tend to lag behind advanced metaheuristics, since they lack
directed arc modeling and efficient learning methods tailored for complex CARP.
In this paper, we introduce an NN-based solver to significantly narrow the gap
with advanced metaheuristics while exhibiting superior efficiency. First, we
propose the direction-aware attention model (DaAM) to incorporate
directionality into the embedding process, facilitating more effective
one-stage decision-making. Second, we design a supervised reinforcement
learning scheme that involves supervised pre-training to establish a robust
initial policy for subsequent reinforcement fine-tuning. It proves particularly
valuable for solving CARP that has a higher complexity than the node routing
problems (NRPs). Finally, a path optimization method is proposed to adjust the
depot return positions within the path generated by DaAM. Experiments
illustrate that our approach surpasses heuristics and achieves decision quality
comparable to state-of-the-art metaheuristics for the first time while
maintaining superior efficiency.
更多查看译文
AI 理解论文
溯源树
样例
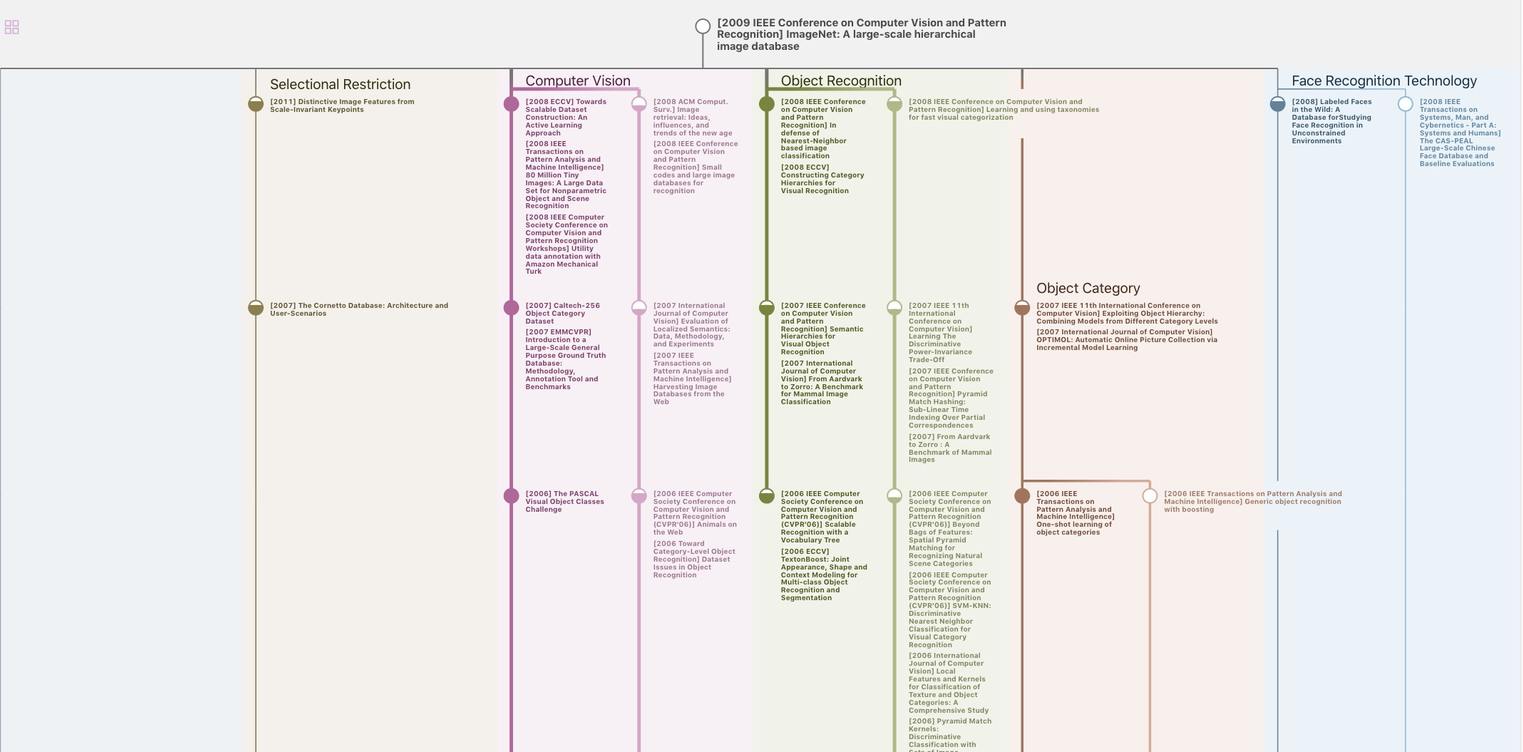
生成溯源树,研究论文发展脉络
Chat Paper
正在生成论文摘要