Synergizing Remora Optimization Algorithm and Transfer Learning for Visual Places Recognition in Intelligent Transportation Systems and Consumer Electronics
IEEE Transactions on Consumer Electronics(2024)
摘要
Visual Place Recognition (VPR) sits at the junction of artificial intelligence (AI) and our physical world, promising to modernize both Consumer Electronics (CE) and Intelligent Transportation Systems (ITS). VPR authorizes self-driving cars to recognize landmarks, straight difficult connections, and realize traffic signs, making independent driving securer and more effective. VPR in ITS includes the application of computer vision (CV) models to study and understand visual information from the environment, permitting transports and set up to understand and direct different surroundings. This sophisticated technique includes the identification and understanding of landmarks, road signs, and other related visual cues, simplifying present decision-making for improved navigation, traffic management, and complete system intelligence. By connecting innovative image processing models and machine learning (ML) methods, visual place detection plays an essential part in enhancing protection, efficacy, and functionality within intelligent transportation methods. Therefore, this study designs a new Remora Optimization Algorithm and Transfer Learning for Visual Places Recognition (ROATL-VPR) in the ITS environment. The ROATL-VPR technique aims to effectually and robustly detect the visual places using an optimal DL model. At the preliminary stage, the ROATL-VPR model mainly pre-processes input images using the Wiener filtering (WF) technique. For learning the complex and intrinsic patterns in the image, the ROATL-VPR technique follows the EfficientNetB3 model as feature extraction. Moreover, the hyperparameter tuning of the EfficientNetB3 method takes place by utilizing the ROA. To identify the visual places precisely, the ROATL-VPR technique applies Manhattan distance which detects the visual places proficiently. The performance validation of the ROATL-VPR method takes place by utilizing a benchmark VPR database. The experimental outcomes highlighted that the ROATL-VPR technique reaches better performance over other DL models in terms of different measures. To learn feature patterns in the preprocessed images, the GANSMIA-CDIA technique follows a feature fusion method comprising different DL methods such as MobileNetV2, AlexNet, ResNet50, and Inceptionv3. Meanwhile, the hyperparameter tuning of these DL techniques takes place using the Social Network Search (SNS) algorithm. Furthermore, the bidirectional long short-term memory (BiLSTM) system can be implemented for the detection and classification of melanoma. For evaluating the performance of the GANSMIA-CDIA technique, a series of simulations were performed on the benchmark Dermoscopic image dataset. The experimental values indicate the excellence of the GANSMIA-CDIA technique over existing techniques.
更多查看译文
关键词
Visual Place Recognition,Intelligent Transportation Systems,Computer Vision,Transfer Learning,Remora Optimization Algorithm
AI 理解论文
溯源树
样例
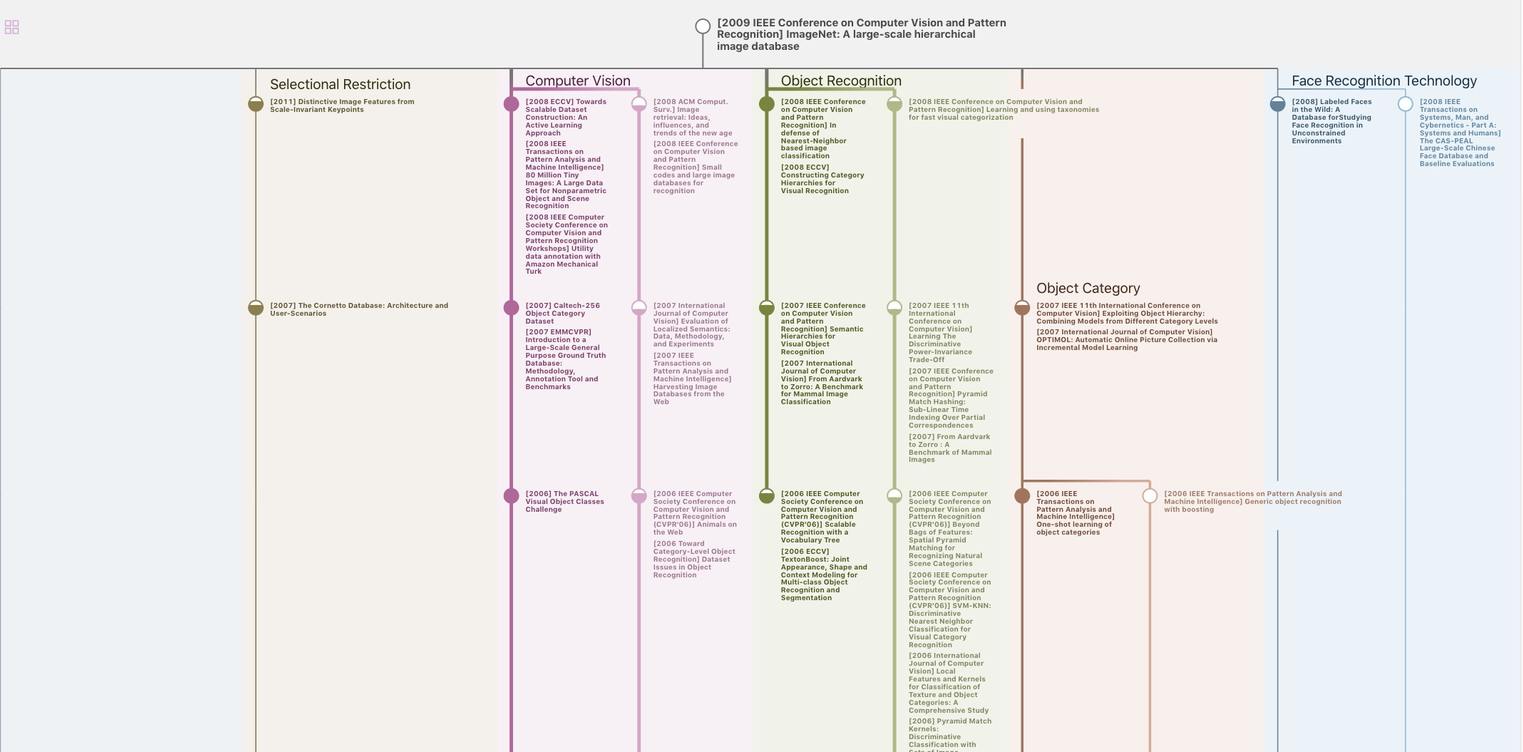
生成溯源树,研究论文发展脉络
Chat Paper
正在生成论文摘要