A privacy-preserving anomaly diagnosis scheme for AC microgrids
Sustainable Energy, Grids and Networks(2024)
摘要
The integration of cyber infrastructure in renewable energy source based AC microgrids (MGs) raises concerns about potential cyber attacks on such systems. An adversary can fabricate the information being exchanged between system components, which may cause false tripping of the installed protection devices. This creates the need for an anomaly diagnosis scheme that can distinguish between faults and cyber attacks to attain a reliable and secure system under large signal disturbances. This work presents a machine learning-based anomaly diagnosis scheme which characterizes these anomalies. The scheme uses the well-known XGBoost approach, and requires local measurements (such as, active power, reactive power, and frequency) of inverter-interfaced distributed energy resources (IIDERs). Since such measurements may be difficult for participants in a system to share across organizational boundaries due to commercial or regulatory concerns, the proposed approach obfuscates the measurements by additive noise while preserving detection accuracy. This scheme was tested on an AC MG network with four IIDERs, modeled on a real-time simulation platform in an OPAL-RT environment. The results validate the effectiveness and accuracy of the proposed scheme under different conditions.
更多查看译文
关键词
Anomaly diagnosis,Cyber–physical system,Cyber attacks,Faults,Inverters,Privacy-preserving,XGBoost
AI 理解论文
溯源树
样例
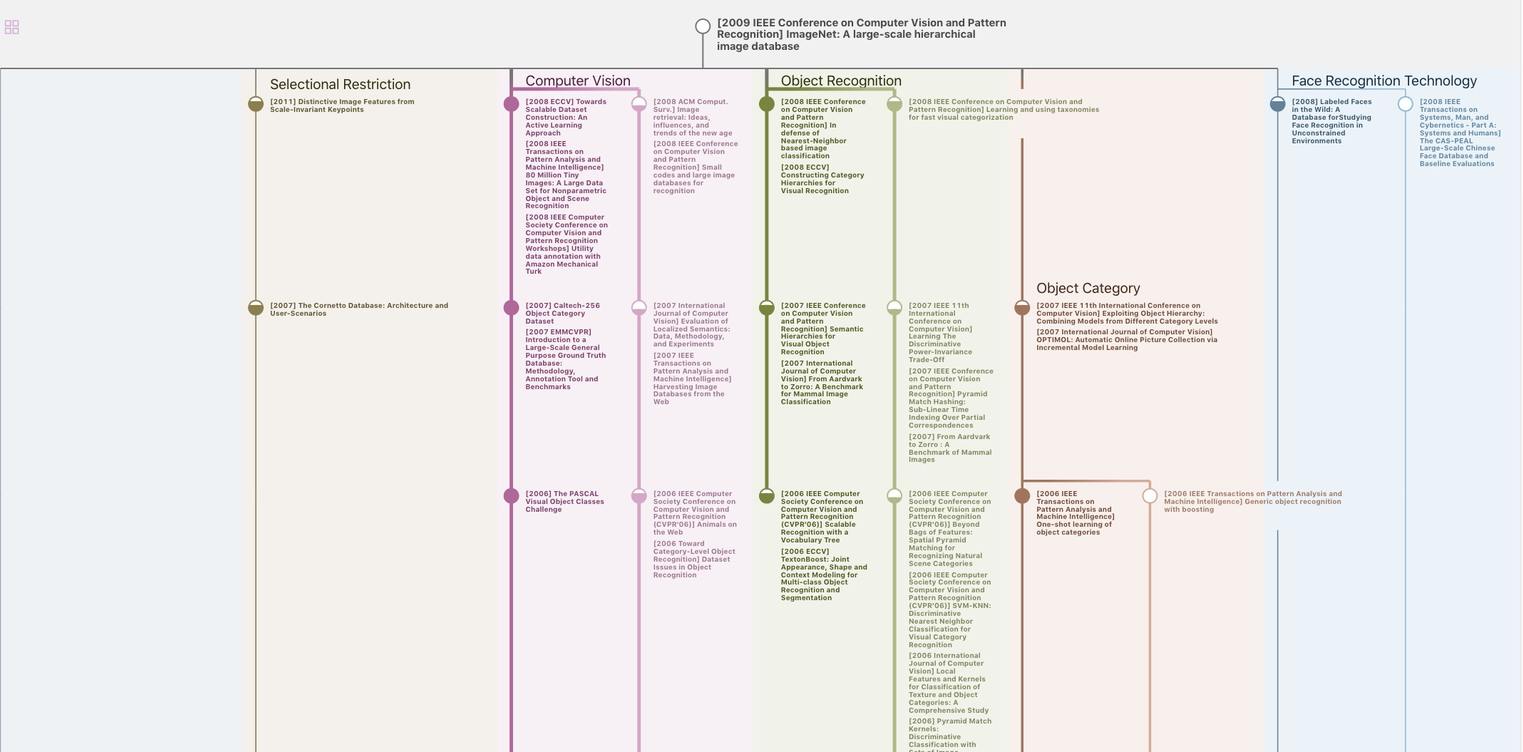
生成溯源树,研究论文发展脉络
Chat Paper
正在生成论文摘要