Inter- and Intra-Domain Potential User Preferences for Cross-Domain Recommendation
IEEE Transactions on Multimedia(2024)
摘要
Data sparsity poses a persistent challenge in Recommender Systems (RS), driving the emergence of Cross-Domain Recommendation (CDR) as a potential remedy. However, most existing CDR methods often struggle to circumvent the transfer of domain-specific information, which are perceived as noise in the target domain. Additionally, they primarily concentrate on inter-domain information transfer, disregarding the comprehensive exploration of data within intra-domains. To address these limitations, we propose SUCCDR (
S
eparating
U
ser features with
C
ompound samples), a novel approach that tackles data sparsity by leveraging both cross-domain knowledge transfer and comprehensive intra-domain analysis. Specifically, to ensure the exclusion of noisy domain-specific features during the transfer process, user preferences are separated into domain-invariant and domain-specific features through three efficient constraints. Furthermore, the unobserved items are leveraged to generate compound samples that intelligently merge observed and unobserved potential user-item interaction, utilizing a simple yet efficient attention mechanism to enable a comprehensive and unbiased representation of user preferences. We evaluate the performance of SUCCDR on two real-world datasets, Douban and Amazon, and compare it with state-of-the-art single-domain and cross-domain recommendation methods. The experimental results demonstrate that SUCCDR outperforms existing approaches, highlighting its ability to effectively alleviate data sparsity problem.
更多查看译文
关键词
Cross-Domain Recommendation,Transfer Learning,Attention Mechanism
AI 理解论文
溯源树
样例
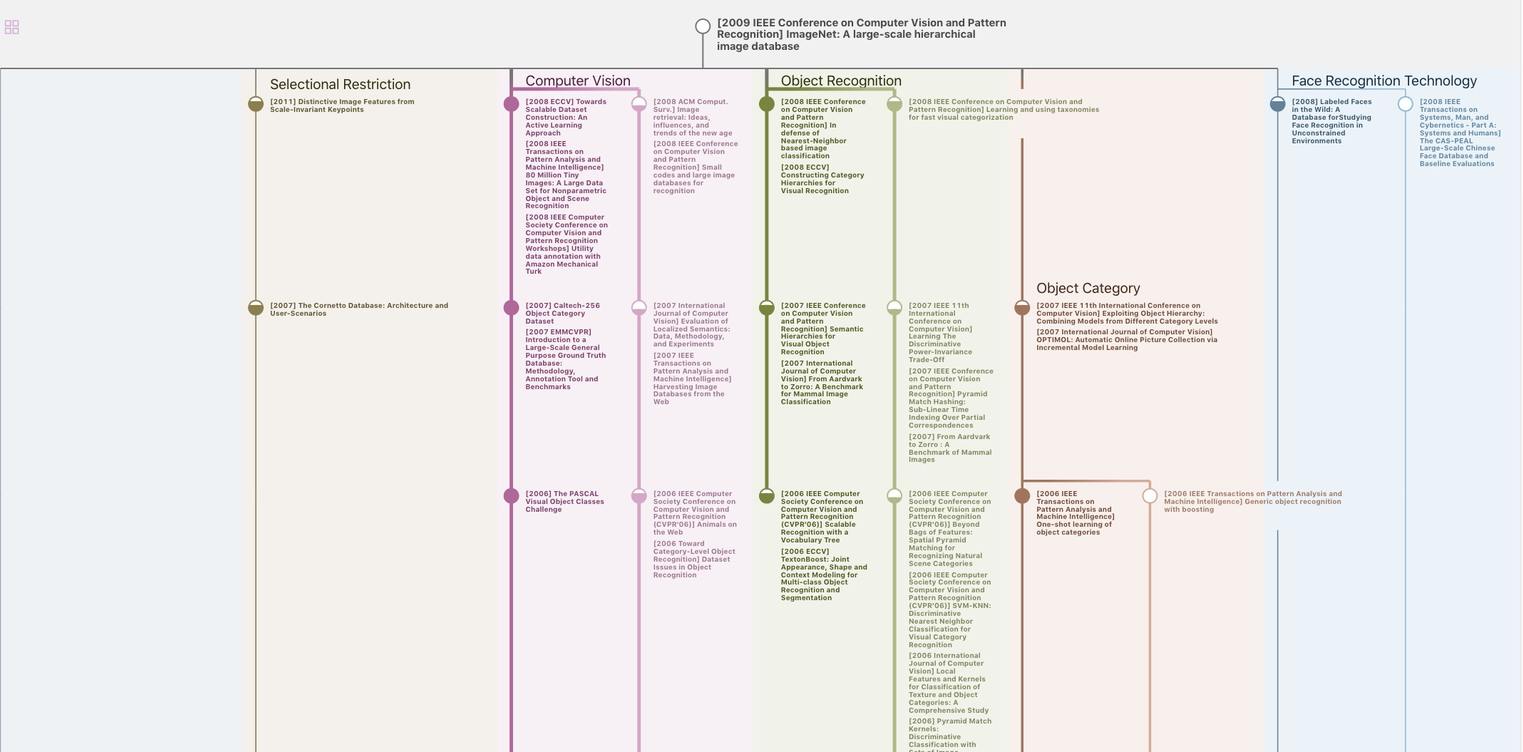
生成溯源树,研究论文发展脉络
Chat Paper
正在生成论文摘要