Research on evaluation method of underwater image quality and performance of underwater structure defect detection model
Engineering Structures(2024)
摘要
Due to the unique environment for the underwater imaging, the acquired images are often blurred, color-distorted, and low-contrast, resulting in low-quality images. Enhancing these images is necessary to improve the effectiveness of target detection. However, there currently lacks of a comprehensive image quality evaluation system specifically designed for underwater images, which hinders the objective analysis of the impact of image enhancement on underwater recognition models and the improvement of model performance. This study proposes an underwater image quality evaluation system based on the improved Multi-Exposure Fusion-based (MEFB) method. The results show that the evaluation system exhibits good consistency between quantitative and qualitative indicators, indicating its value and practical significance. Combining the evaluation system with the performance of the YOLOv8 model trained on various underwater enhancement algorithm datasets, the following conclusions are drawn: 1) The results of UCIQE and UIQM indicators do not always align with qualitative results; 2) Detection models trained on enhanced image datasets exhibit varying degrees of decrease in recall rate compared to models trained on the original dataset, and the accuracy depends on the quality of enhanced images; 3) Secondary enhancement does not notably improve both quantitative image indicators and detection model performance; 4) Detection models trained on low-quality datasets generally have better generalization than models trained on high-quality datasets. Furthermore, an improved defect detection model of underwater structures based on YOLOv8 is also proposed, which addresses the challenges of multi-scale and lightweight design for underwater structural defects. The ablation experiment shows that the improved model achieves an increase in mAP50 from 96.8% to 97.3%, mAP50–95 from 81.9% to 84.4%, and score from 0.8474 to 0.8673, as compared to the original model.
更多查看译文
关键词
Deep learning,Evaluation system,Image enhancement,Object detection,Underwater structures,YOLOv8
AI 理解论文
溯源树
样例
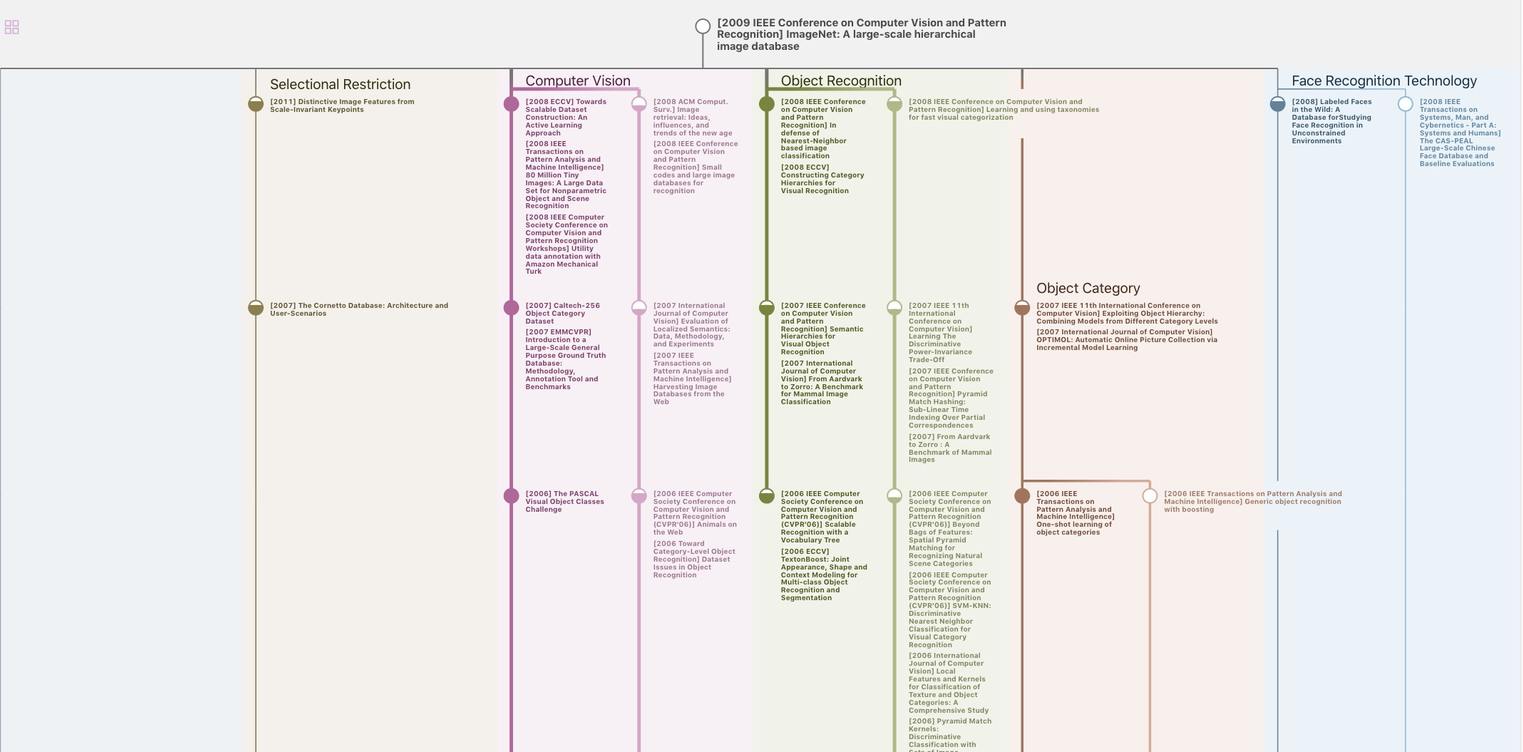
生成溯源树,研究论文发展脉络
Chat Paper
正在生成论文摘要