Multicriteria Machine Learning Model Assessment-Residuum Analysis Review
ELECTRONICS(2024)
摘要
The use of machine learning (ML) and its applications is one of the leading research areas nowadays. Neural networks have recently gained enormous popularity and many works in various fields use them in the hope of improving previous results. The application of the artificial intelligence (AI) methods and the rationale for this decision is one issue, but the assessment of such a model is a completely different matter. People mostly use mean square error or less often mean absolute error in the absolute or percentage versions. One should remember that an error does not equal an error and a single value does not provide enough knowledge about the causes of some behavior. Proper interpretation of the results is crucial. It leads to further model improvement. It might be challenging, but allows us to obtain better and more robust solutions, which ultimately solve real-life problems. The ML model assessment is the multicriteria task. A single measure delivers only a fraction of the picture. This paper aims at filling that research gap. Commonly used integral measures are compared with alternative measures like factors of the Gaussian and non-Gaussian statistics, robust statistical estimators, tail index and the fractional order. The proposed methodology delivers new single-criteria indexes or the multicriteria approach, which extend the statistical concept of the moment ratio diagram (MRD) into the index ratio diagram (IRD). The proposed approach is validated using real data from the Full Truck Load cost estimation example. It compares 35 different ML regression algorithms applied to that task. The analysis gives an insight into the properties of the selected methods, enables their comparison and homogeneity analysis and ultimately leads towards constructive suggestions for their eventual proper use. The paper proposes new indexes and concludes that correct selection of the residuum analysis methodology makes the assessment and the ML regression credible.
更多查看译文
关键词
machine learning,residuum analysis,tail index,L-moments,fractional order,alpha-stable distribution,robust statistics,full truck loads
AI 理解论文
溯源树
样例
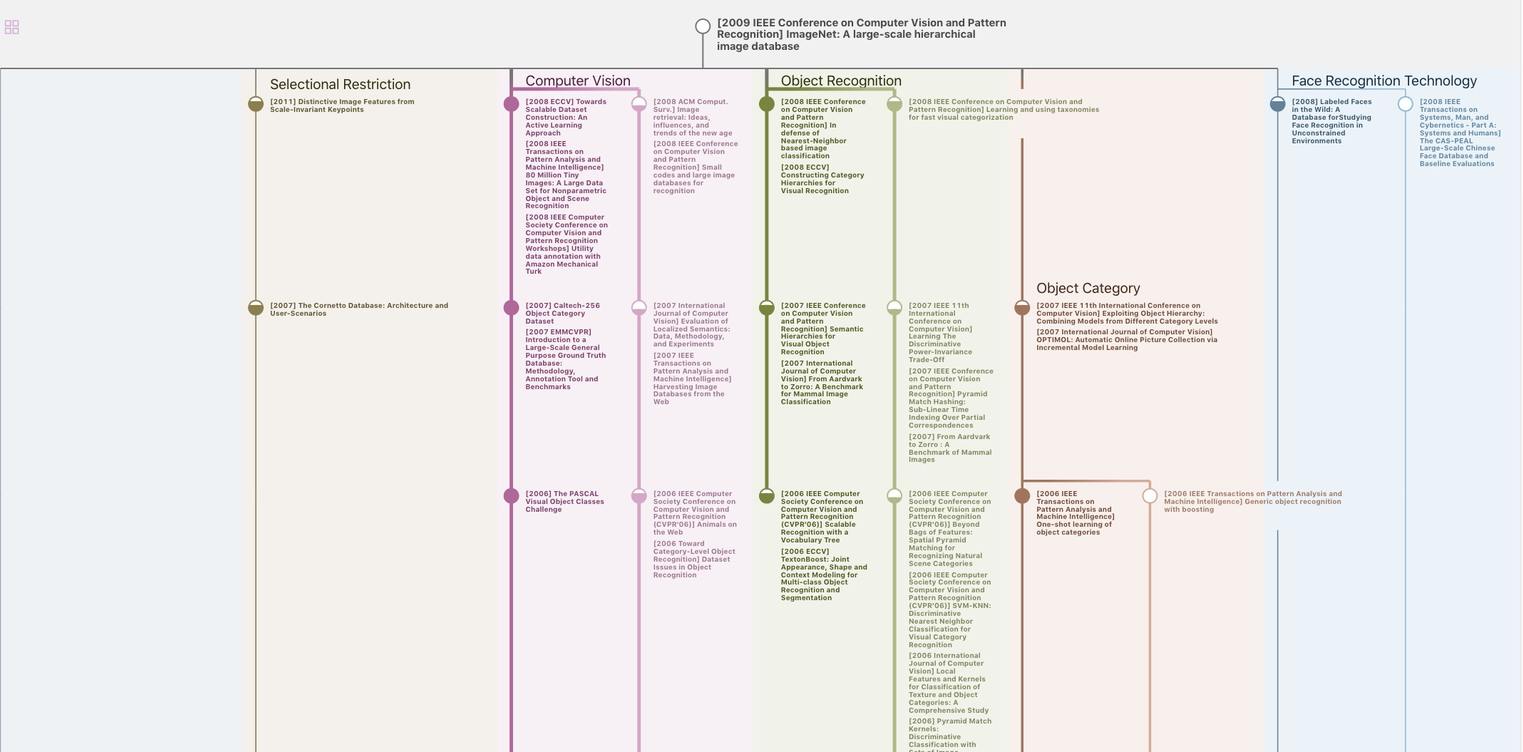
生成溯源树,研究论文发展脉络
Chat Paper
正在生成论文摘要