Comparing Performances of Predictive Models of Toxicity after Radiotherapy for Breast Cancer Using Different Machine Learning Approaches
CANCERS(2024)
摘要
Purpose. Different ML models were compared to predict toxicity in RT on a large cohort (n = 1314). Methods. The endpoint was RTOG G2/G3 acute toxicity, resulting in 204/1314 patients with the event. The dataset, including 25 clinical, anatomical, and dosimetric features, was split into 984 for training and 330 for internal tests. The dataset was standardized; features with a high p-value at univariate LR and with Spearman rho>0.8 were excluded; synthesized data of the minority were generated to compensate for class imbalance. Twelve ML methods were considered. Model optimization and sequential backward selection were run to choose the best models with a parsimonious feature number. Finally, feature importance was derived for every model. Results. The model's performance was compared on a training-test dataset over different metrics: the best performance model was LightGBM. Logistic regression with three variables (LR3) selected via bootstrapping showed performances similar to the best-performing models. The AUC of test data is slightly above 0.65 for the best models (highest value: 0.662 with LightGBM). Conclusions. No model performed the best for all metrics: more complex ML models had better performances; however, models with just three features showed performances comparable to the best models using many (n = 13-19) features.
更多查看译文
关键词
AI models,early-stage breast cancer,modeling,radiotherapy,toxicity
AI 理解论文
溯源树
样例
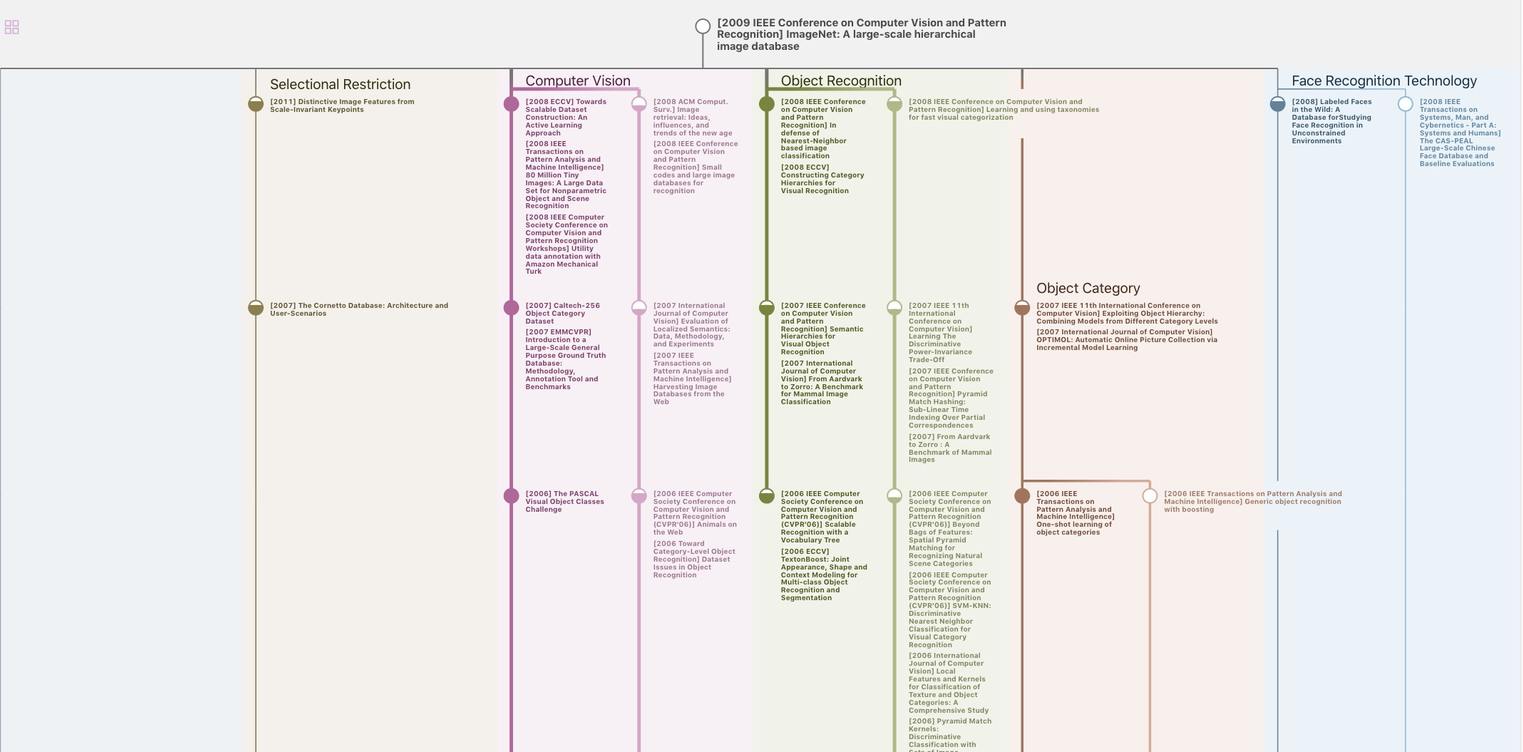
生成溯源树,研究论文发展脉络
Chat Paper
正在生成论文摘要