Handling Overlapping Asymmetric Data Sets-A Twice Penalized P-Spline Approach
MATHEMATICS(2024)
摘要
Aims: Overlapping asymmetric data sets are where a large cohort of observations have a small amount of information recorded, and within this group there exists a smaller cohort which have extensive further information available. Missing imputation is unwise if cohort size differs substantially; therefore, we aim to develop a way of modelling the smaller cohort whilst considering the larger. Methods: Through considering traditionally once penalized P-Spline approximations, we create a second penalty term through observing discrepancies in the marginal value of covariates that exist in both cohorts. Our now twice penalized P-Spline is designed to firstly prevent over/under-fitting of the smaller cohort and secondly to consider the larger cohort. Results: Through a series of data simulations, penalty parameter tunings, and model adaptations, our twice penalized model offers up to a 58% and 46% improvement in model fit upon a continuous and binary response, respectively, against existing B-Spline and once penalized P-Spline methods. Applying our model to an individual's risk of developing steatohepatitis, we report an over 65% improvement over existing methods. Conclusions: We propose a twice penalized P-Spline method which can vastly improve the model fit of overlapping asymmetric data sets upon a common predictive endpoint, without the need for missing data imputation.
更多查看译文
关键词
P-Spline,penalized regression,smoothing,asymmetric data,B-Spline,non-Parametric,MASLD,MASH,health data science
AI 理解论文
溯源树
样例
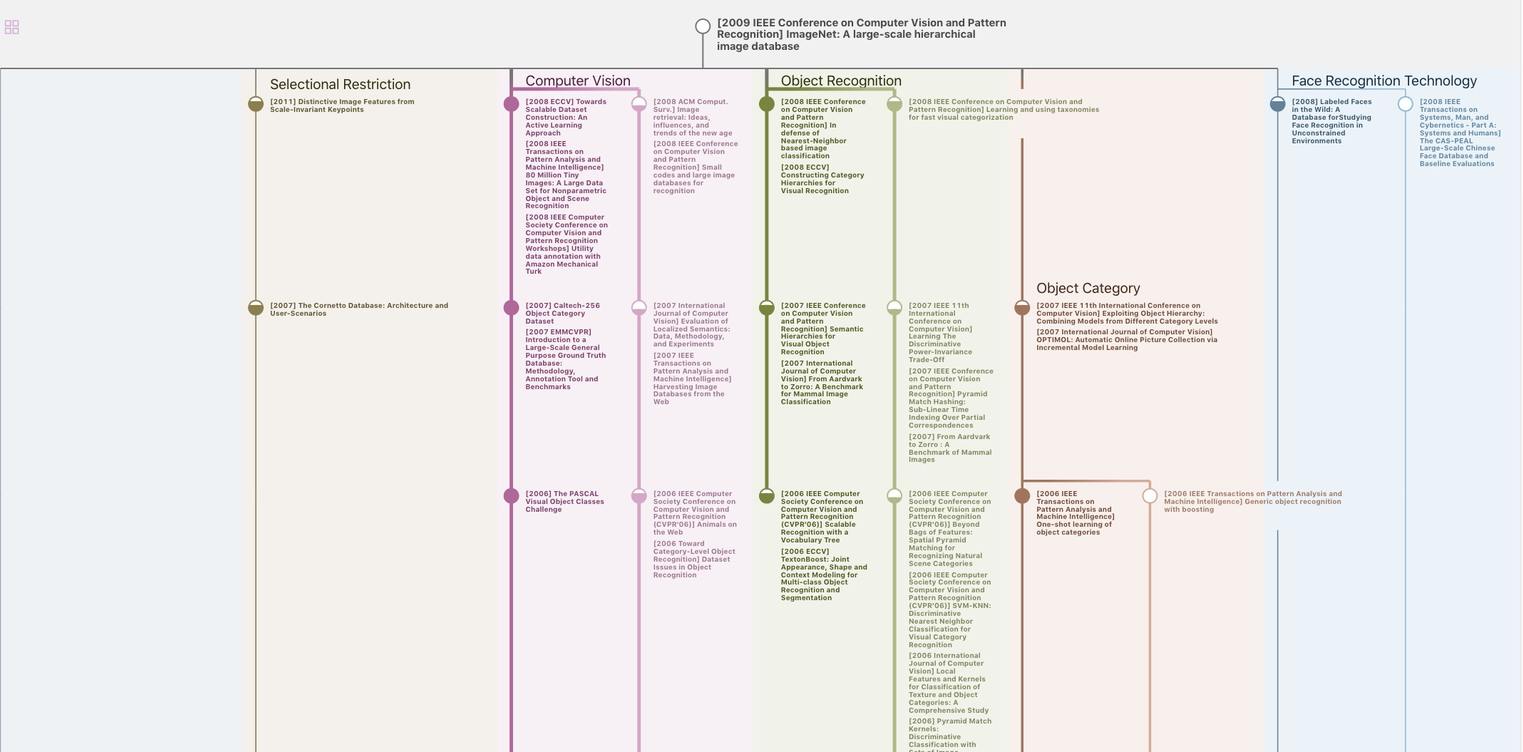
生成溯源树,研究论文发展脉络
Chat Paper
正在生成论文摘要