Face De-Identification Using Convolutional Neural Network (CNN) Models for Visual-Copy Detection
APPLIED SCIENCES-BASEL(2024)
摘要
The proliferation of media-sharing platforms has led to issues with illegally edited content and the distribution of pornography. To protect personal information, de-identification technologies are being developed to prevent facial identification. Existing de-identification methods directly alter the pixel values in the face region, leading to reduced feature representation and identification accuracy. This study aims to develop a method that minimizes the possibility of personal identification while effectively preserving important features for image- and video-copy-detection tasks, proposing a new deep-learning-based de-identification approach that surpasses traditional pixel-based alteration methods. We introduce two de-identification models using different approaches: one emphasizing the contours of the original face through feature inversion and the other generating a blurred version of the face using D2GAN (Dual Discriminator Generative Adversarial Network). Both models were evaluated on their performance in image- and video-copy-detection tasks before and after de-identification, demonstrating effective feature preservation. This research presents new possibilities for personal-information protection and digital-content security, contributing to digital-rights management and law enforcement.
更多查看译文
关键词
de-identification,CNN,feature inversion,GAN,D2GAN,image-copy detection,video-copy detection
AI 理解论文
溯源树
样例
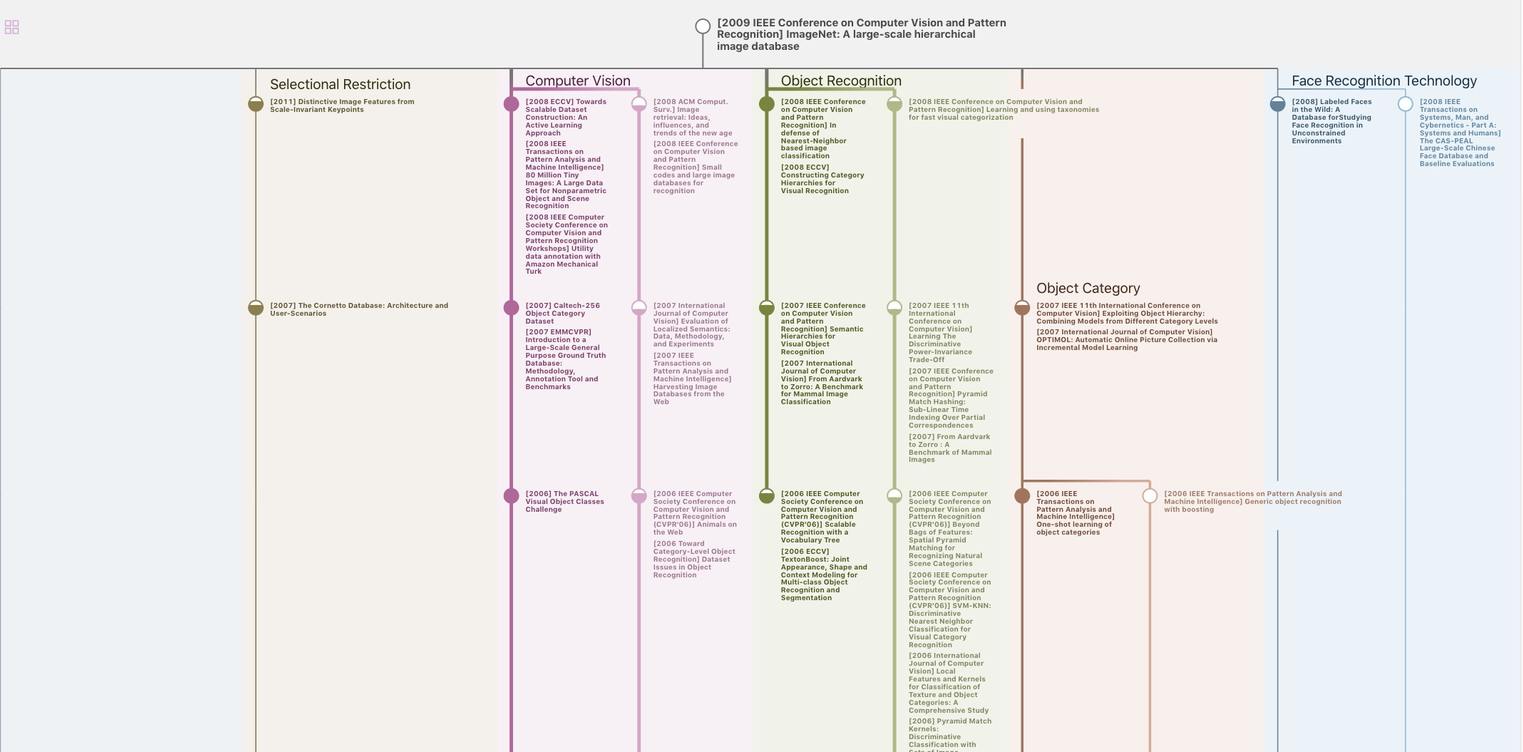
生成溯源树,研究论文发展脉络
Chat Paper
正在生成论文摘要