Hierarchical representation and interpretable learning for accelerated quality monitoring in machining process
CIRP JOURNAL OF MANUFACTURING SCIENCE AND TECHNOLOGY(2024)
摘要
While modern 5 -axis computer numerical control (CNC) systems offer enhanced design flexibility and reduced production time, the dimensional accuracy of the workpiece is significantly compromised by geometric errors, thermal deformations, cutting forces, tool wear, and fixture -related factors. In -situ sensing, in conjunction with machine learning (ML), has recently been implemented on edge devices to synchronously acquire and agilely analyze high -frequency and multifaceted data for the prediction of workpiece quality. However, limited edge computational resources and lack of interpretability in ML models obscure the understanding of key quality -influencing signals. This research introduces InterpHD, a novel graph -based hyperdimensional computing framework that not only assesses workpiece quality in 5 -axis CNC on edge, but also characterizes key signals vital for evaluating the quality from in -situ multichannel data. Specifically, a hierarchical graph structure is designed to represent the relationship between channels (e.g., spindle rotation, three linear axes movements, and the rotary A and C axes), parameters (e.g., torque, current, power, and tool speed), and the workpiece dimensional accuracy. Additionally, memory refinement, separability, and parameter significance are proposed to assess the interpretability of the framework. Experimental results on a hybrid 5 -axis LASERTEC 65 DED CNC machine indicate that InterpHD not only achieves a 90.7% F1 -Score in characterizing a 25.4 mm counterbore feature deviation but also surpasses other ML models with an F1 -Score margin of up to 73.0%. The interpretability of the framework reveals that load and torque have 12 times greater impact than power and velocity feed forward for the characterization of geometrical dimensions. InterpHD offers the potential to facilitate causal discovery and provide insights into the relationships between process parameters and part quality in manufacturing.
更多查看译文
关键词
Computer-integrated manufacturing,Statistical quality control,Edge computing,Symbolic learning,Graph representation
AI 理解论文
溯源树
样例
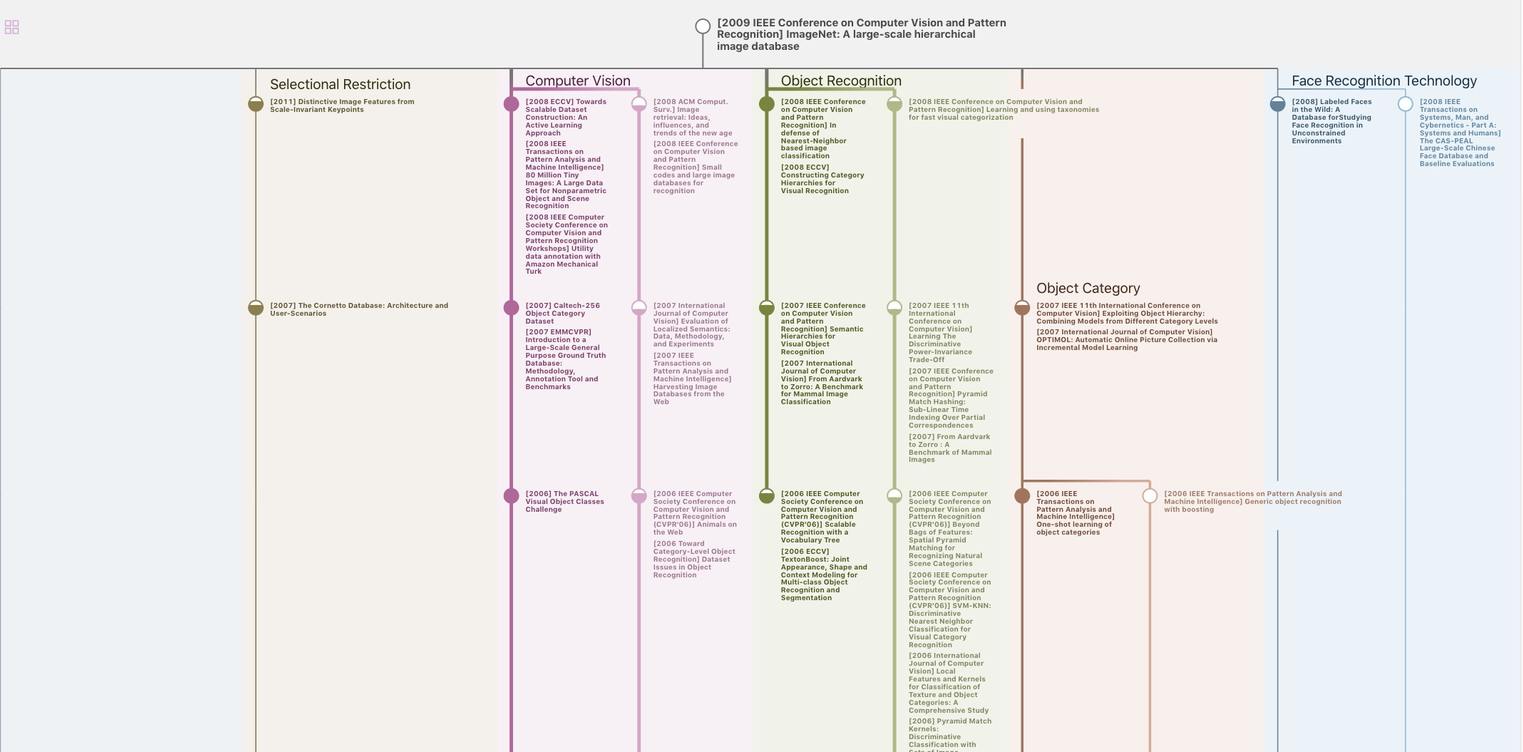
生成溯源树,研究论文发展脉络
Chat Paper
正在生成论文摘要