Factors and pathways of non-suicidal self-injury in children: insights from computational causal analysis.
Frontiers in public health(2024)
摘要
Background:Non-suicidal self-injury (NSSI) has become a significant public health issue, especially prevalent among adolescents. The complexity and multifactorial nature of NSSI necessitate a comprehensive understanding of its underlying causal factors. This research leverages the causal discovery methodology to explore these causal associations in children.
Methods:An observational dataset was scrutinized using the causal discovery method, particularly employing the constraint-based approach. By integrating machine learning and causal inference techniques, the study aimed to determine direct causal relationships associated with NSSI. The robustness of the causal relationships was evaluated using three methods to construct and validate it: the PC (Peter and Clark) method, Fast Causal Inference (FCI) method, and the GAE (Graphical Autoencoder) method.
Results:Analysis identified nine nodes with direct causal relationships to NSSI, including life satisfaction, depression, family dysfunction, sugary beverage consumption, PYD (positive youth development), internet addiction, COVID-19 related PTSD, academic anxiety, and sleep duration. Four principal causal pathways were identified, highlighting the roles of lockdown-induced lifestyle changes, screen time, positive adolescent development, and family dynamics in influencing NSSI risk.
Conclusions:An in-depth analysis of the factors leading to Non-Suicidal Self-Injury (NSSI), highlighting the intricate connections among individual, family, and pandemic-related influences. The results, derived from computational causal analysis, underscore the critical need for targeted interventions that tackle these diverse causative factors.
更多查看译文
关键词
NSSI,causal discovery,mental health,artificial intelligence,risk factors,COVID-19
AI 理解论文
溯源树
样例
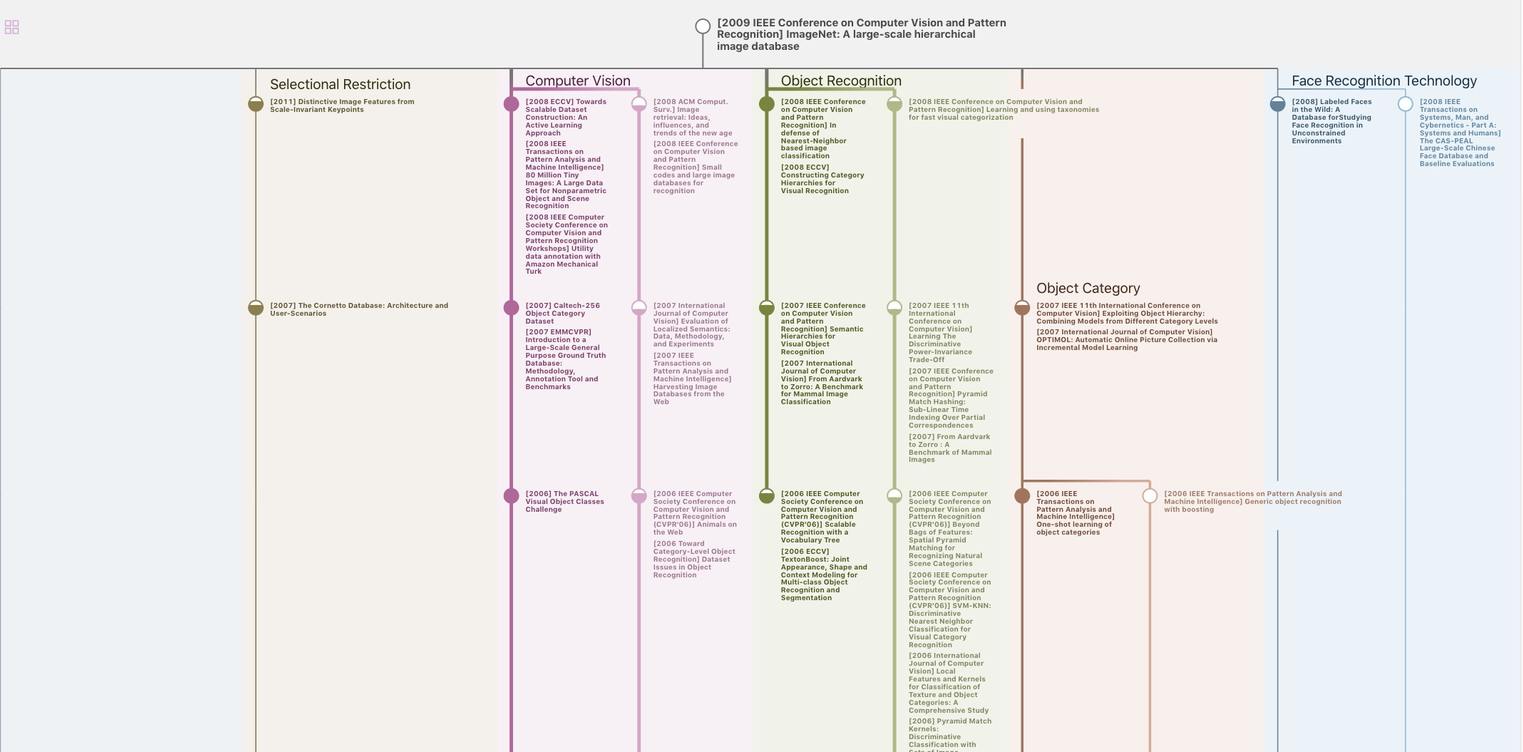
生成溯源树,研究论文发展脉络
Chat Paper
正在生成论文摘要