Influence of data assimilation on tropical waves
crossref(2024)
摘要
Precipitation forecasts in the tropics are poor due to large model and initial condition errors, leaving ample room for improvement. In particular, it has been hypothesized that the coupling of tropical waves and convection offers a source of predictability, suggesting that capturing these waves accurately in the model and in initial conditions could lead to improved precipitation forecasts. In this work, we investigate whether standard data assimilation (DA) algorithms like the Ensemble Kalman Filter (EnKF) are fundamentally capable of recovering tropical waves and thereby provide initial conditions that lead to skillful precipitation forecasts. To capture the essence of tropical dynamics without contamination of land-sea contrasts, sea-surface temperature gradients and influences from the extra-tropics, we use a tropical aqua channel configuration at 13km grid-spacing with the ICON numerical weather prediction model. Further, to isolate the role of the initial conditions provided by DA, we assume a perfect model. In our setup, Kelvin waves dominate over other wave types and primarily modulate precipitation. In addition, there is evidence of a Madden-Julian-Oscillation (MJO)-like feature that appears coupled to large-scale convective activity. We show that when sufficient wind observations are assimilated, the DA can reduce the errors in the representation of the Kelvin waves sufficiently to provide accurate precipitation forecasts up to several weeks. Surprisingly, even the MJO-like rainfall event, which starts after a forecast lead time of 10 days, is captured by the forecast ensemble. Further, we find that accurate initial conditions for humidity are important to slow down error growth for all model variables. Like emphasized by several other studies, we conclude that wind observations are by far the most important input to achieve skillful tropical forecasts. A secondary challenge is to improve initial conditions of humidity by developing DA algorithms to account for non-Gaussian error statistics. Investigating the role of model error in DA and the resulting forecasts is left for future work.
更多查看译文
AI 理解论文
溯源树
样例
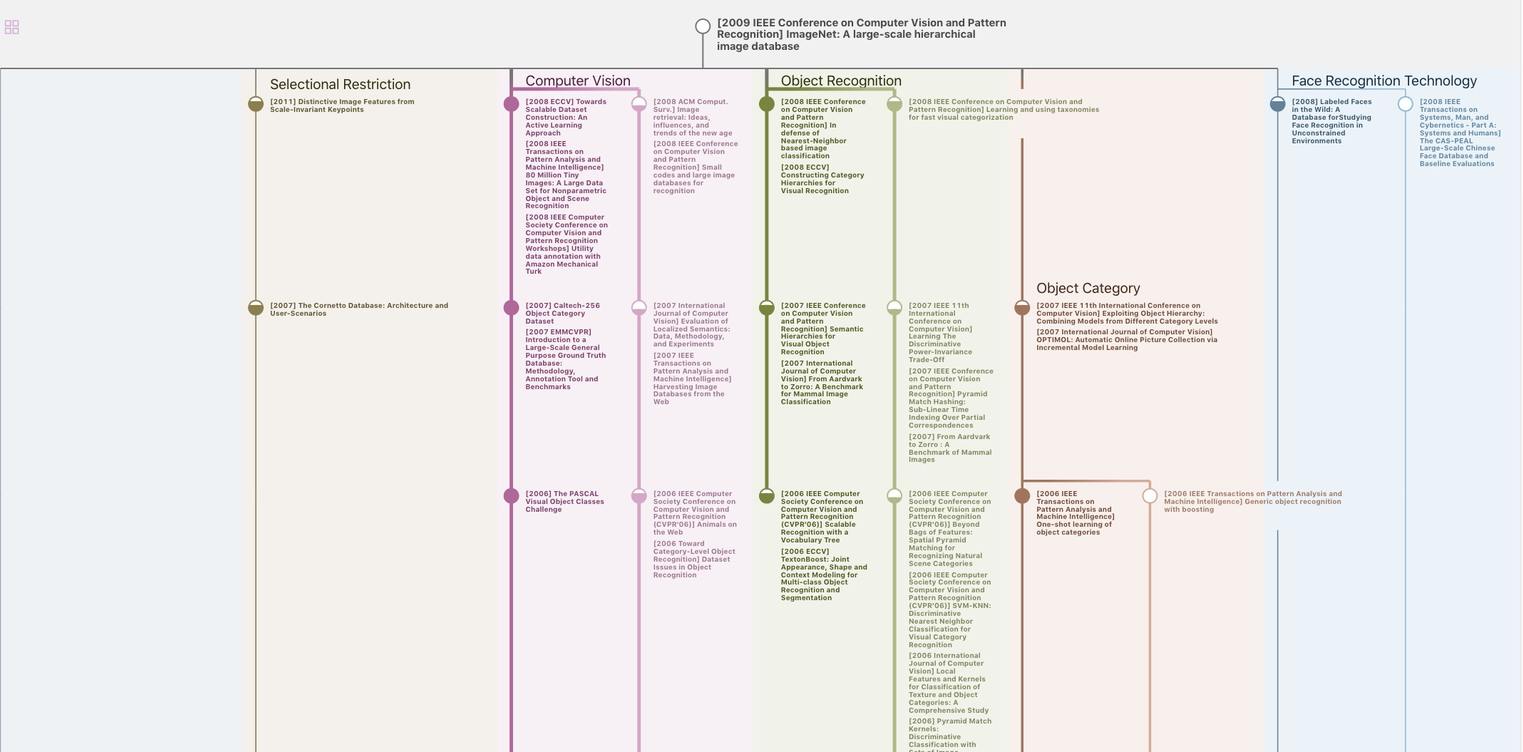
生成溯源树,研究论文发展脉络
Chat Paper
正在生成论文摘要