A Triple-Branch Hybrid Attention Network With Bitemporal Feature Joint Refinement for Remote-Sensing Image Semantic Change Detection
IEEE TRANSACTIONS ON GEOSCIENCE AND REMOTE SENSING(2024)
摘要
Compared with binary change detection (BCD), semantic change detection (SCD) further provides the category information of bitemporal changed regions which is significant for the practical application of Earth observation. Although the recently proposed triple-branch structures including one BCD branch and two classification branches can effectively achieve task balance, they still need to employ the carefully designed difference extraction (DE) module and branch interactions to capture the bitemporal correlations, which increases the complexity of the semantic information utilization. In this article, we propose a new triple-branch network named JFRNet to tackle this challenge. From the perspective of the SCD process, because the category information and the change information are both derived from bitemporal images, we take the joint bitemporal features as the unified input, which can help each branch perceive the bitemporal semantic correlations without any additional interaction operations. From the perspective of the SCD structure, we introduce the convolutional attention fusion module (CAFM) and the convolutional attention refinement module (CARM) to unify the branch structure, which can help our model refine the unique semantic information without any specially designed DE modules. Extensive experiment results on three available datasets indicate that compared with the baseline methods, our proposed JFRNet successfully simplifies the reasoning process and obtains better SCD performance.
更多查看译文
关键词
Semantics,Decoding,Task analysis,Correlation,Feature extraction,Data mining,Convolution,Attention mechanism,branch interaction,convolutional neural network (CNN),semantic change detection (SCD)
AI 理解论文
溯源树
样例
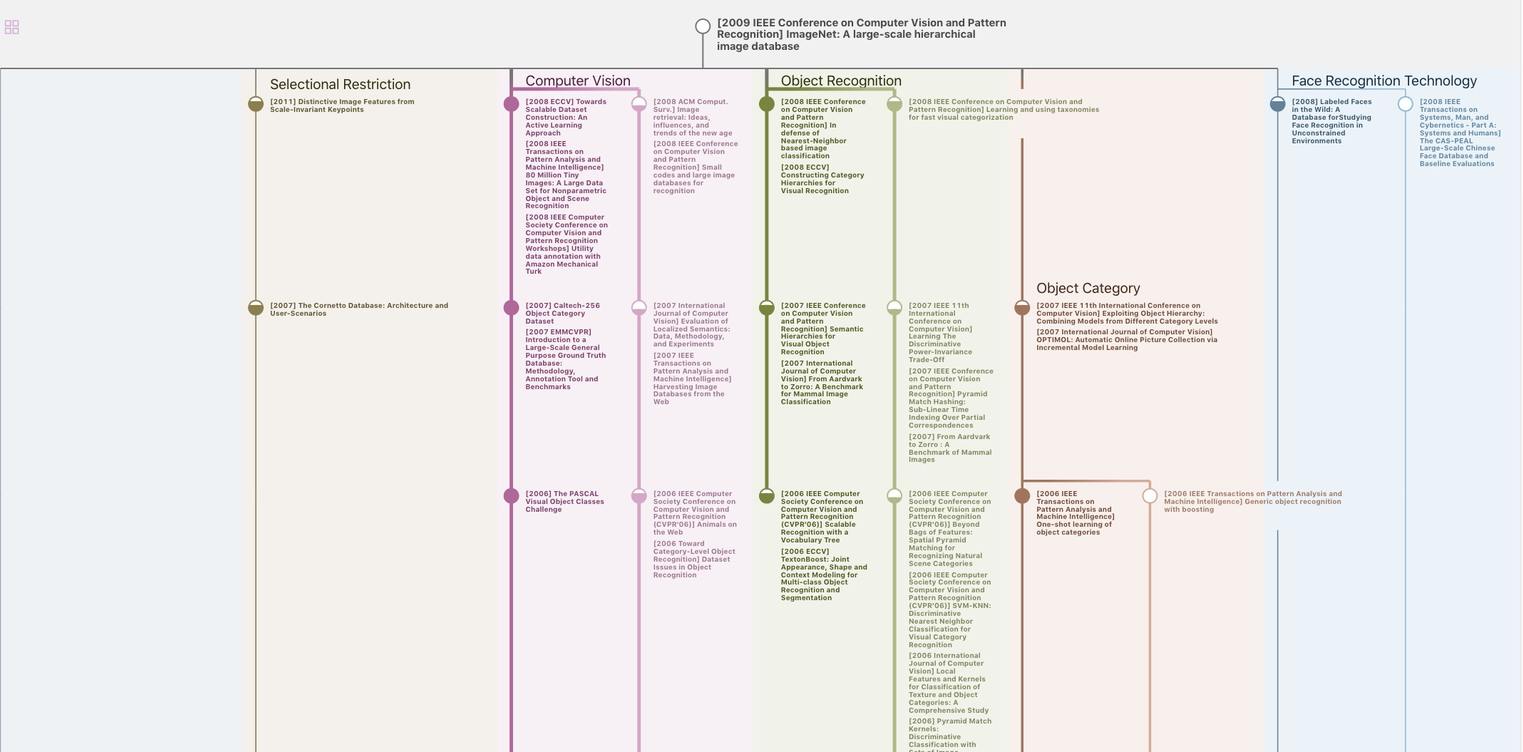
生成溯源树,研究论文发展脉络
Chat Paper
正在生成论文摘要