Hybrid Metaheuristic Optimization Algorithms with Least-Squares Support Vector Machine and Boosted Regression Tree Models for Prediction of Air-Blast Due to Mine Blasting
Natural Resources Research(2024)
摘要
After each blasting operation in surface mines, undesirable environmental impacts, such as air-blast (AB) and ground vibration, are inevitable. Therefore, minimizing and controlling these impacts are crucial in order to reduce environmental problems. This study presents new and practical advanced machine learning methods for AB prediction using 62 datasets gathered from four quarry sites in Malaysia. The developed models were constructed based on the boosted regression tree (BRT) and least-squares support vector machine (LSSVM), improved with three metaheuristic algorithms: the gray wolf optimizer (GWO), genetic algorithm (GA), and artificial bee colony (ABC). Six hybrid models, namely BRT–GA, BRT–ABC, BRT–GWO, LSSVM–GA, LSSVM–ABC, and LSSVM–GWO models, were developed and their performances were evaluated using metrics such as R-squared correlation and other methods like the Taylor diagram and quantile–quantile plots. To provide a better assessment of the models' performances, the dataset were categorized into training and testing parts. The results demonstrated that, among the six hybrid models, the LSSVM–GWO model provided the highest efficiency in the testing part while the BRT–GWO model had the best accuracy but the performance of BRT–GWO was the best in the training part. In other words, the BRT hybrid models had the best performance in training, and LSSVM hybrid models in the testing part. The results indicate the effectiveness of combining GWO with LSSVM and BRT models to predict AB.
更多查看译文
关键词
Blast-induced air-blast,LSSVM,BRT,Optimization algorithms
AI 理解论文
溯源树
样例
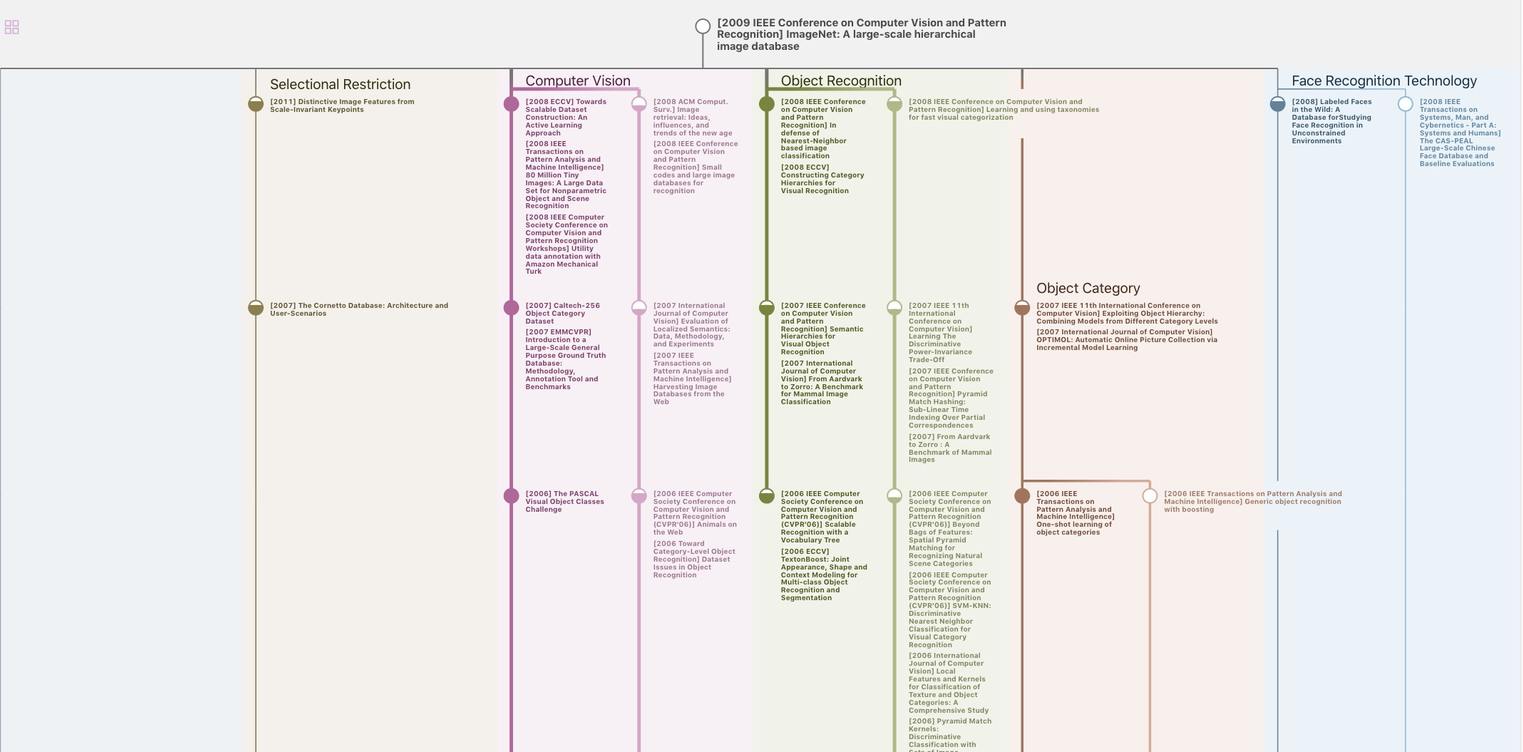
生成溯源树,研究论文发展脉络
Chat Paper
正在生成论文摘要