Machine learning-based soil quality assessment for enhancing environmental monitoring in iron ore mining-impacted ecosystems
Journal of Environmental Management(2024)
摘要
In November 2015, a catastrophic rupture of the Fundão dam in Mariana (Brazil), resulted in extensive socio-economic and environmental repercussions that persist to this day. In response, several reforestation programs were initiated to remediate the impacted regions. However, accurately assessing soil health in these areas is a complex endeavor. This study employs machine learning techniques to predict soil quality indicators that effectively differentiate between the stages of recovery in these areas. For this, a comprehensive set of soil parameters, encompassing 3 biological, 16 chemical, and 3 physical parameters, were evaluated for samples exposed to mining tailings and those unaffected, totaling 81 and 6 samples, respectively, which were evaluated over 2 years. The most robust model was the decision tree with a restriction of fewer levels to simplify the tree structure. In this model, Cation Exchange Capacity (CEC), Microbial Biomass Carbon (MBC), Base Saturation (BS), and Effective Cation Exchange Capacity (eCEC) emerged as the most pivotal factors influencing model fitting. This model achieved an accuracy score of 92% during training and 93% during testing for determining stages of recovery. The model developed in this study has the potential to revolutionize the monitoring efforts conducted by regulatory agencies in these regions. By reducing the number of parameters that necessitate evaluation, this enhanced efficiency promises to expedite recovery monitoring, simultaneously enhancing cost-effectiveness while upholding the analytical rigor of assessments.
更多查看译文
关键词
Feature selection,Fundão dam,Monitoring,Decision tree and soil health
AI 理解论文
溯源树
样例
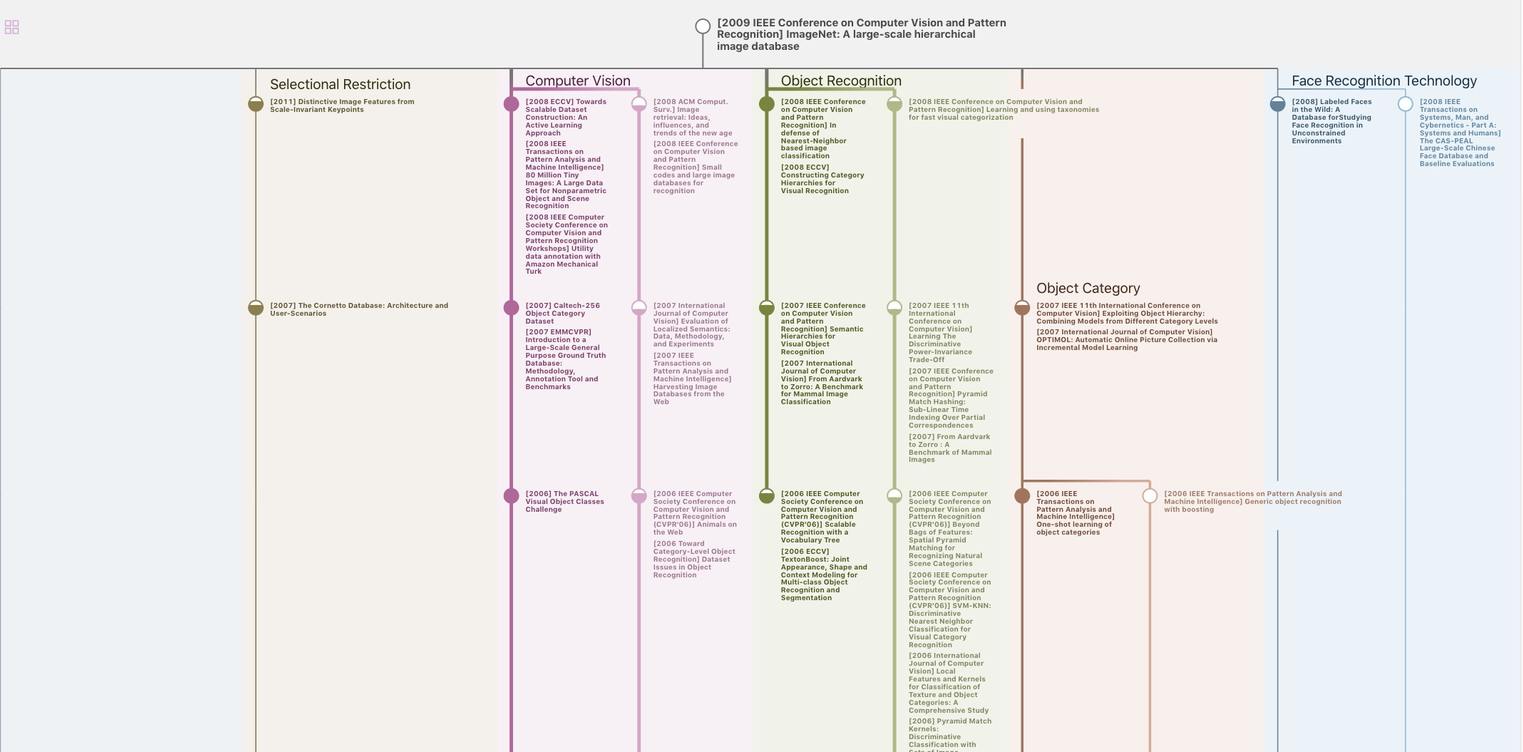
生成溯源树,研究论文发展脉络
Chat Paper
正在生成论文摘要