Unsupervised Feature Selection via Collaborative Embedding Learning
IEEE Transactions on Emerging Topics in Computational Intelligence(2024)
摘要
Unsupervised feature selection is vital in explanatory learning and remains challenging due to the difficulty of formulating a learnable model. Recently, graph embedding learning has gained widespread popularity in unsupervised learning, which extracts low-dimensional representation based on graph structure. Nevertheless, such an embedding scheme for unsupervised feature selection will distort original features due to the spatial transformation by extraction. To address this problem, this paper proposes a collaborative graph embedding model for unsupervised feature selection via jointly using soft-threshold and low-dimensional embedding learning. The former learns a threshold selection matrix for feature weighting in the original space. The latter extracts embedded representation in low-dimensional space to reveal the latent graph structure. By collaborative learning, the proposed method can simultaneously perform unsupervised feature selection in the original space and adaptive graph learning via dual embedding. Extensive experiments on five benchmark datasets demonstrate that the proposed method achieves superior performance compared to eight competing methods.
更多查看译文
关键词
Embedding learning,adaptive graph,unsupervised feature selection,feature extraction,graph learning
AI 理解论文
溯源树
样例
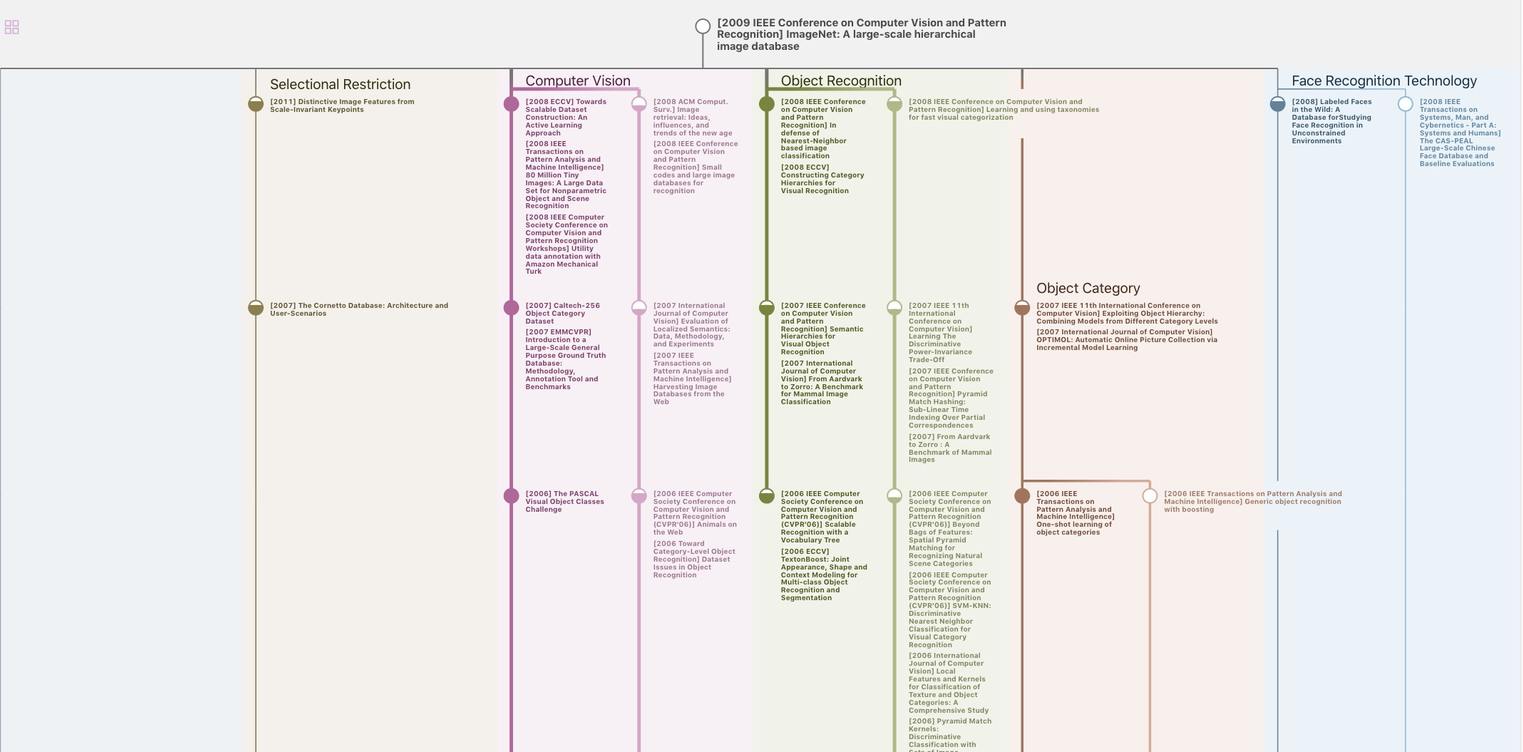
生成溯源树,研究论文发展脉络
Chat Paper
正在生成论文摘要