ASG-HOMGAT: a high-order multi-head graph attention network with adaptive small graph structure for rolling bearing fault diagnosis
MEASUREMENT SCIENCE AND TECHNOLOGY(2024)
摘要
Traditional Euclidean spatial data processing is difficult to capture the inherent relationships of unstructured data such as bearing vibration signals. Representing vibration signals in graphical form helps to preserve their topological structure and temporal information. Secondly, most existing graph convolutional network methods are based on large graph structured data, which incurs certain memory overhead when aggregating high-order neighborhood node information and ignores important information between samples in the global graph structure. To address these issues, this paper proposes a high-order multi-head graph attention network based on an adaptive small graph structure (ASG-HOMGAT) for fault diagnosis of rolling bearings. Firstly, the adaptive preprocessing layer is used to adaptively denoise and compress the one-dimensional time-domain vibration signal, generating small rule graph data with topological structure. Then, these small graph structured data samples are input into a higher-order graph neural network, which aggregates features from multiple higher-order neighborhoods to achieve richer feature representations and fully explore the intrinsic correlation between samples. Finally, these features are aggregated into a reinforced representation of graph nodes through a multi head attention mechanism, and a SoftMax classifier is used for fault classification. The experimental results show that the ASG-HOMGAT method has better performance compared to mainstream graph neural network diagnostic models. The code and model will be released at: https://github.com/ding-ss/ASG-HOMGAT.
更多查看译文
关键词
fault diagnosis,graph convolutional networks,adaptive small graph structure,rolling bearing,high-order multi-head graph attention network
AI 理解论文
溯源树
样例
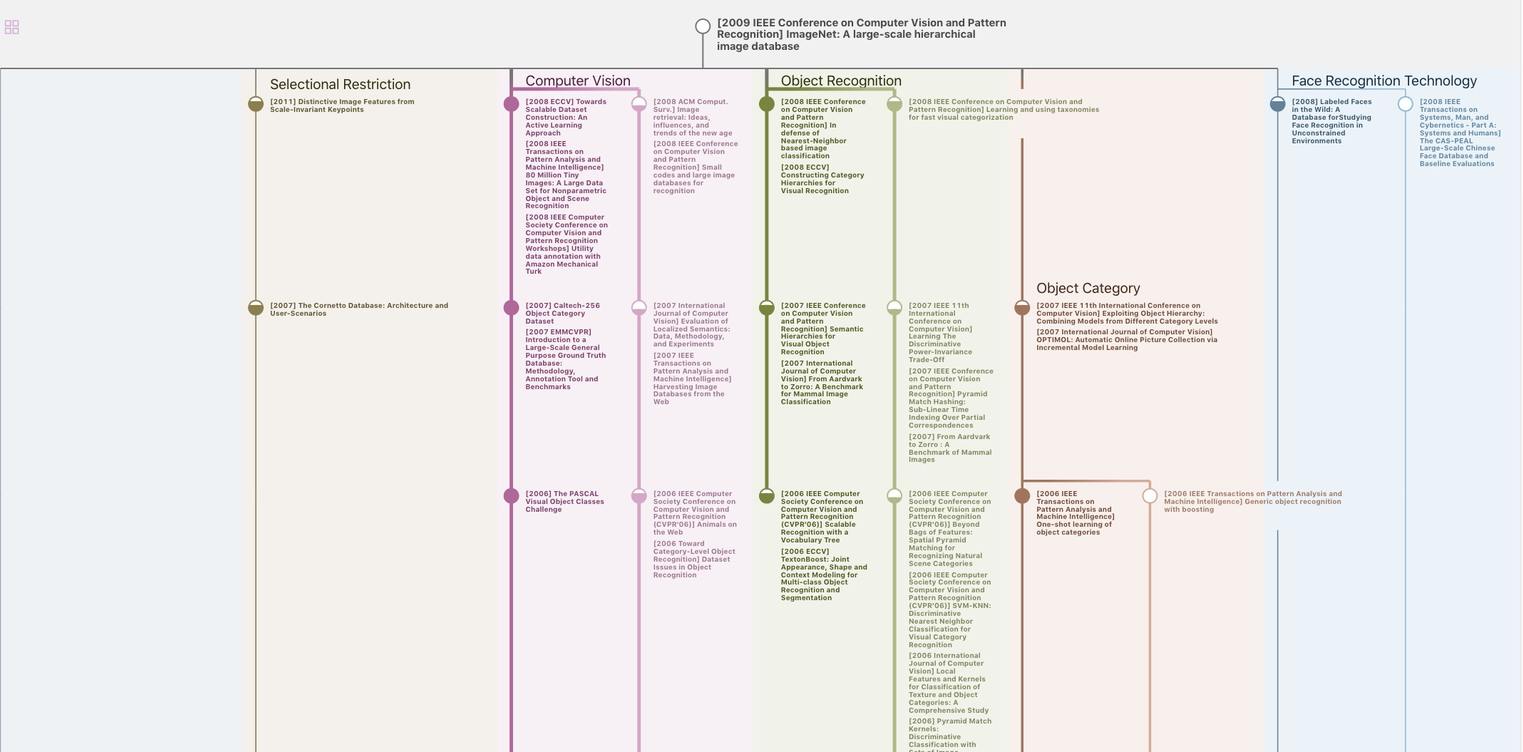
生成溯源树,研究论文发展脉络
Chat Paper
正在生成论文摘要